Generative AI in cybersecurity is reshaping threat management by bolstering detection capabilities and fortifying defenses. Through advanced data analytics and real-time anomaly detection, it enables proactive responses to dynamic cyber threats. This innovative technology not only enhances threat detection but also facilitates the creation of synthetic data to build robust models. By revolutionizing traditional cybersecurity approaches, generative AI offers a powerful tool in combating evolving threats. Its ability to adapt defenses in real-time and simulate realistic attacks marks a significant shift in cybersecurity strategies. Embrace this transformative technology for a more resilient cybersecurity framework.
Key Takeaways
- Synthetic data generation for robust threat simulations.
- Real-time anomaly detection enhancing proactive defense.
- AI-driven threat detection for dynamic response.
- Advanced threat simulation for predictive measures.
- Harmonious blend of human expertise and AI technology.
The Impact of Generative AI
With its advanced capabilities in analyzing data and detecting anomalies in real-time, generative AI has greatly influenced the field of cybersecurity. This innovative technology plays an essential role in combating evolving cyber threats by providing efficient threat detection mechanisms that outperform traditional methods.
By comparing current behaviors with historical data, generative AI can identify deviations and enable immediate preventive action, bolstering security measures against cyber attacks.
This proactive approach to cybersecurity not only enhances threat detection but also empowers organizations to predict and prevent cyber threats before they materialize. Generative AI's ability to block malicious activities effectively showcases its practical applications in fortifying cybersecurity defenses.
Additionally, the ethical considerations surrounding the use of AI in cybersecurity highlight the importance of responsible implementation to guarantee data privacy and security are upheld.
Enhancing Threat Detection
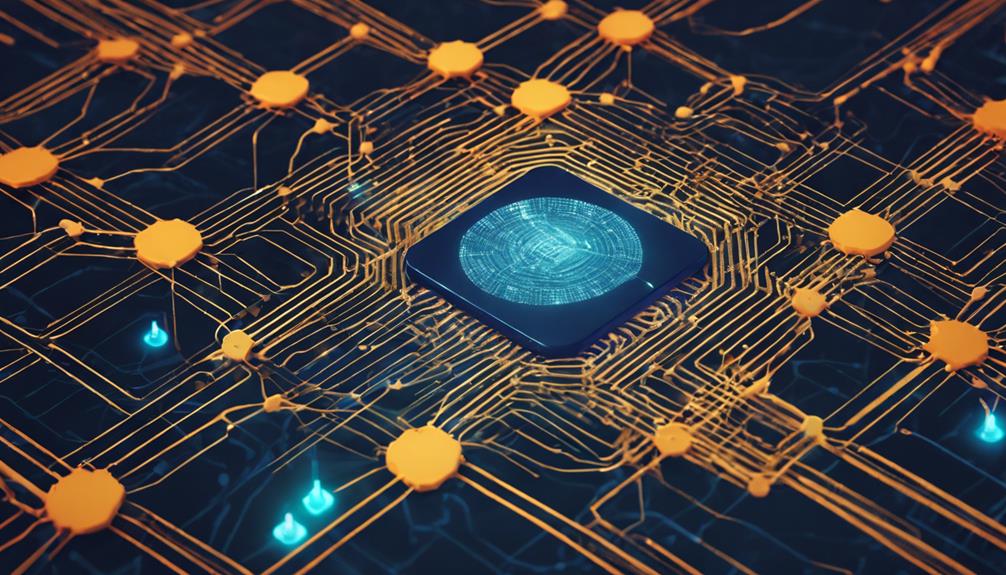
Enhancing threat detection capabilities, generative AI in cybersecurity utilizes real-time data analysis to proactively identify anomalies and patterns in network activities. This innovative approach to threat detection offers significant advantages in combating the ever-evolving landscape of cybersecurity threats.
Here's how generative AI can help:
- Analyzing Large Datasets: By processing vast amounts of data in real-time, generative AI can sift through complex network traffic, user behavior, and system logs to pinpoint potential threats swiftly.
- Identify Patterns: Through continuous adaptation and comparison with historical data, generative AI can identify patterns indicative of malicious activities, enabling organizations to stay ahead of potential threats.
- Proactive Detection and Prevention: By detecting deviations from normal behavior, generative AI empowers organizations to take immediate preventive action, mitigating potential risks before they escalate.
Incorporating generative AI into cybersecurity strategies enhances threat detection and prevention, providing a powerful tool to defend against sophisticated cyber threats.
Synthetic Data Generation
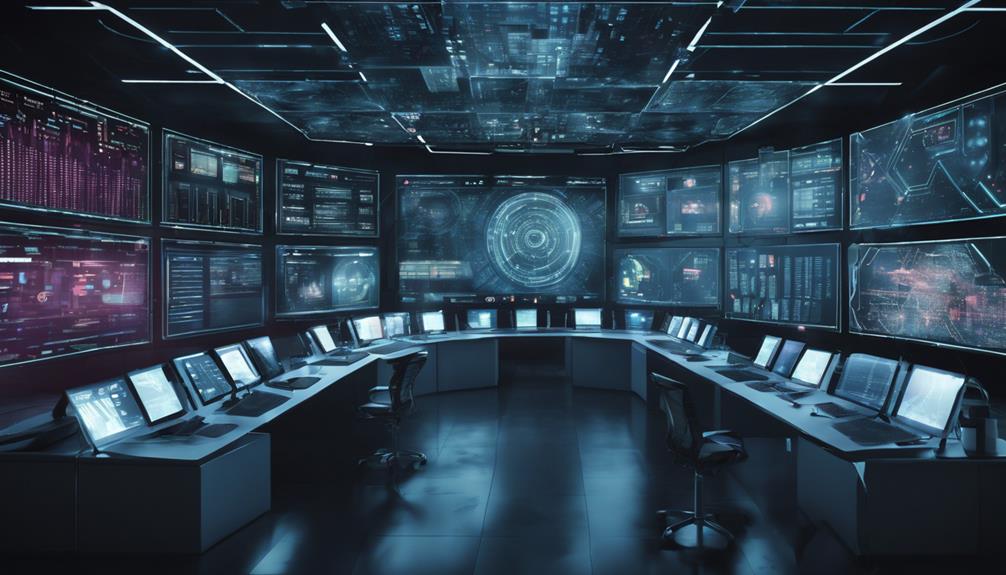
Generative AI cybersecurity leverages synthetic data generation to enhance the training of AI models and bolster threat detection capabilities. By creating artificial data, this technique improves the performance of AI algorithms through the generation of diverse datasets, enabling more robust cybersecurity models. Synthetic data generation plays an essential role in simulating various cyberattack scenarios, aiding in the reinforcement of defense mechanisms against evolving threats. Additionally, it addresses data scarcity issues commonly faced in cybersecurity by providing ample data for training and testing purposes. To illustrate the significance of synthetic data generation in generative AI cybersecurity, the table below highlights key points related to this innovative approach:
Synthetic Data Generation in Generative AI Cybersecurity |
---|
Enhances training of AI algorithms |
Generates diverse datasets for robust models |
Simulates cyberattack scenarios for defense mechanisms |
Addresses data scarcity in cybersecurity |
Improves overall robustness of cybersecurity models |
Adaptive Cyber Defenses
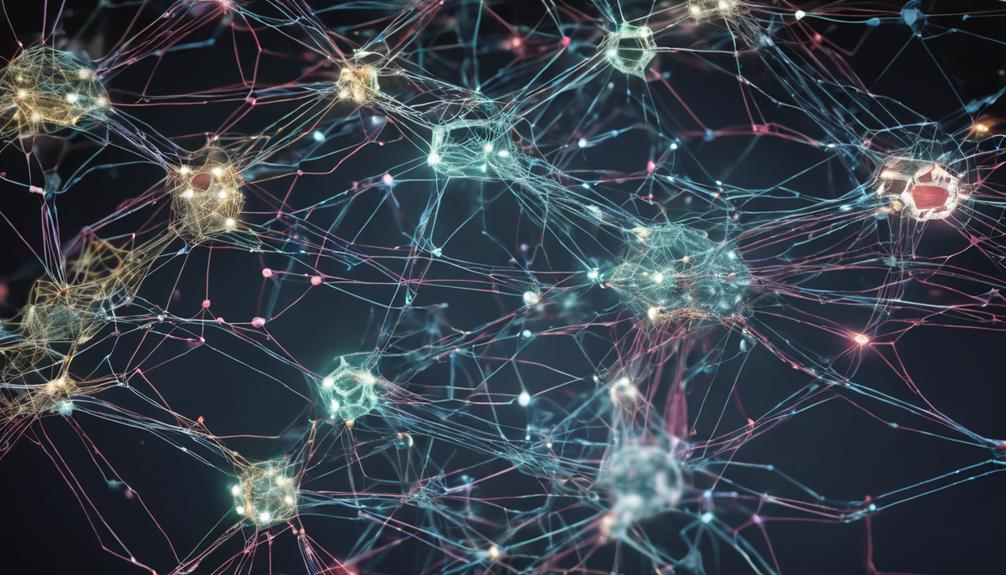
Adaptive cyber defenses are essential in today's ever-evolving threat landscape. By dynamically responding to emerging threats and leveraging AI-driven detection capabilities, organizations can stay ahead of cybercriminals.
These defenses provide a proactive approach to cybersecurity, enabling quick identification and mitigation of potential risks.
Dynamic Threat Response
Employing advanced generative AI technology, dynamic threat response, also known as adaptive cyber defenses, revolutionizes how organizations combat evolving cybersecurity threats in real-time. These defenses leverage generative AI to analyze network traffic, user behavior, and system logs for anomalies and deviations. By comparing current behavior with historical data, adaptive cyber defenses can proactively detect and mitigate potential cyberattacks.
Three key aspects highlight the effectiveness of dynamic threat response:
- Real-time Analysis: Adaptive cyber defenses continuously monitor activities to identify threats promptly.
- Threat Vectors Identification: Generative AI provides detailed insights into potential attack pathways, enabling targeted defense strategies.
- Proactive Nature: By anticipating risks and taking preventive measures, adaptive cyber defenses help organizations minimize breach risks and fortify their cybersecurity posture against emerging threats.
Incorporating dynamic threat response into cybersecurity frameworks enhances resilience and responsiveness, ensuring organizations stay ahead of cyber adversaries in the ever-evolving digital landscape.
Ai-Driven Threat Detection
Enhancing cybersecurity capabilities, AI-driven threat detection leverages advanced technology to analyze real-time data for proactive defense against evolving cyber threats. By employing behavior analysis and anomaly detection, this adaptive cybersecurity approach enables swift identification of emerging threats.
Unlike traditional signature-based methods, AI-driven threat detection continuously evolves by comparing current behavior with historical data to detect deviations promptly.
Through real-time analysis of network traffic, user behavior, and system logs, generative AI enhances cybersecurity defenses by accurately pinpointing potential risks. Its proactive defense mechanisms empower organizations to stay ahead of cyber threats, triggering preventive actions before significant damage occurs.
The extensive applications of AI-driven threat detection are wide-ranging, offering a holistic solution for businesses seeking robust cybersecurity measures in the face of ever-changing threat landscapes. Embracing this innovative approach is key to fortifying digital infrastructures and safeguarding sensitive data from malicious actors.
Realistic Attack Simulation
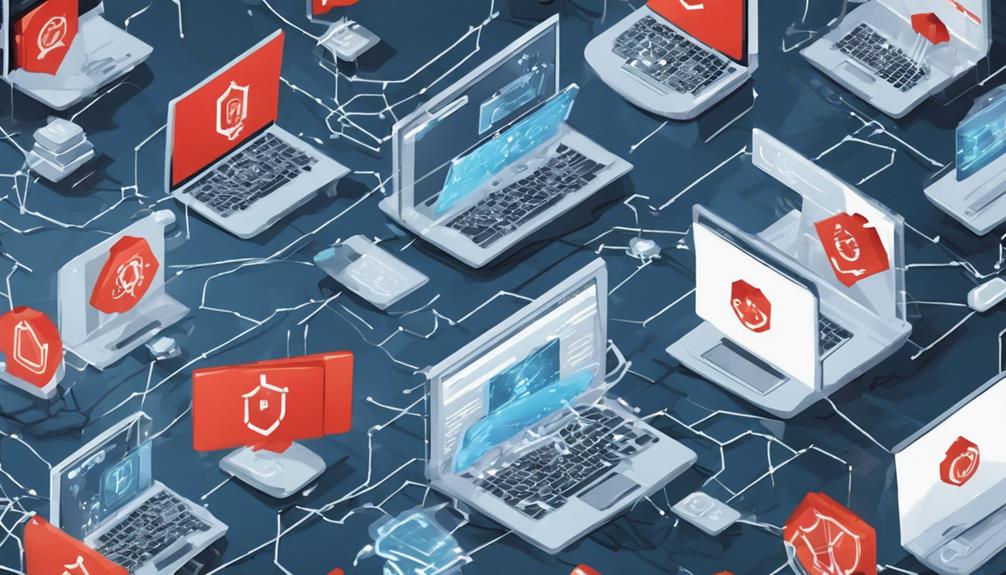
Utilizing generative AI technology in cybersecurity enables organizations to conduct realistic attack simulations, enhancing their preparedness against evolving cyber threats. These simulations play an important role in strengthening defense mechanisms and evaluating the effectiveness of security controls.
Here's how realistic attack simulation with generative AI contributes to cybersecurity:
- Enhanced Preparedness: By creating dynamic attack scenarios that replicate actual threats, organizations can better prepare for potential cyber incidents and proactively improve their security posture.
- Identification of Vulnerabilities: Realistic attack simulations help in identifying weaknesses in existing defenses, allowing organizations to address vulnerabilities before they can be exploited by malicious actors.
- Improved Incident Response: Through simulating advanced attack scenarios, generative AI assists in refining incident response strategies, enabling organizations to react swiftly and effectively to cyber threats, minimizing potential damage.
Revolutionary Cybersecurity Strategies
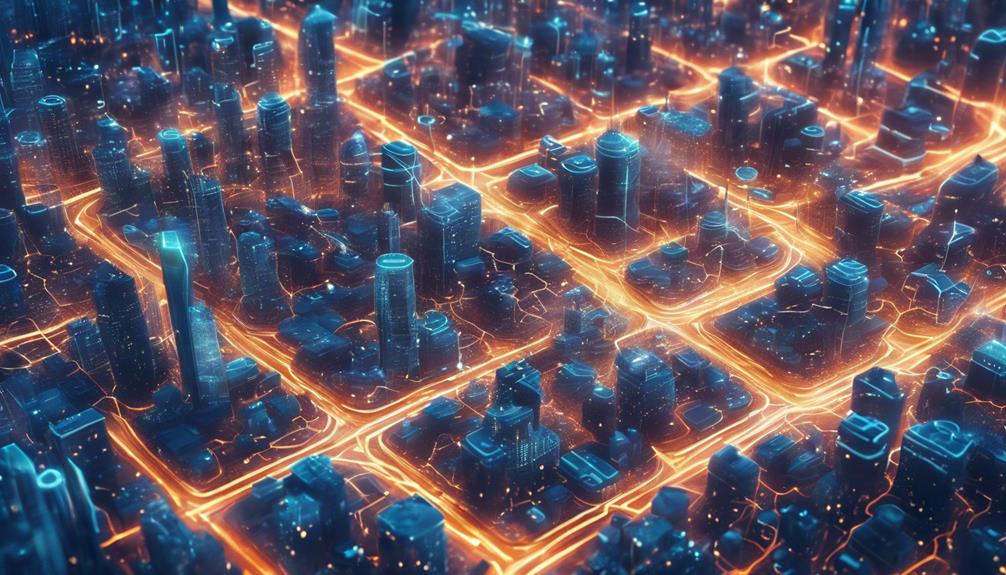
Revolutionary cybersecurity strategies are at the forefront of protecting organizations from evolving cyber threats.
AI threat detection offers proactive measures by leveraging deep learning models to identify and counter sophisticated attacks effectively.
Adaptive defense systems, combining human expertise with AI capabilities, play an important role in transforming traditional defense mechanisms to guarantee resilience in the face of modern cyber challenges.
AI Threat Detection
The integration of AI threat detection strategies marks a significant advancement in modern cybersecurity practices. Leveraging machine learning models such as Generative Adversarial Networks (GANs), cyber threats are identified through the analysis of anomalous behavior patterns.
Here are key points regarding AI threat detection:
- Real-Time Analysis: AI threat detection strategies sift through vast amounts of data instantaneously, enabling the identification of potential security breaches as they occur.
- Proactive Detection: By comparing current behavior with historical data, AI systems can proactively detect deviations that may indicate cyber threats, allowing for swift response and mitigation.
- Insightful Defense Strengthening: Generative AI not only detects threats but also provides detailed insights into threat vectors, empowering cybersecurity experts to implement targeted responses and strengthen overall defense mechanisms efficiently.
In essence, AI-powered threat detection streamlines cybersecurity efforts, automates routine tasks, and offers a focused approach to combatting increasingly complex cyber threats.
Adaptive Defense Systems
Adaptive defense systems represent a cutting-edge approach in cybersecurity, harnessing the power of generative AI to dynamically analyze and respond to evolving cyber threats. These systems utilize advanced machine learning models, such as GANs, to simulate potential cyberattacks and strengthen defensive strategies. By leveraging generative AI, adaptive defense systems enable proactive threat detection by identifying unusual behaviors and patterns in real-time. This automation of security measures not only enhances the overall security posture of organizations but also allows human resources to focus on tackling more complex cybersecurity challenges. The dynamic nature of generative AI within adaptive defense systems fortifies cybersecurity frameworks against emerging threats, providing a robust line of defense for safeguarding sensitive data and organizational assets.
Benefits of Adaptive Defense Systems |
---|
Enhanced threat detection |
Proactive response to cyber threats |
Efficient resource allocation |
Future of Threat Management
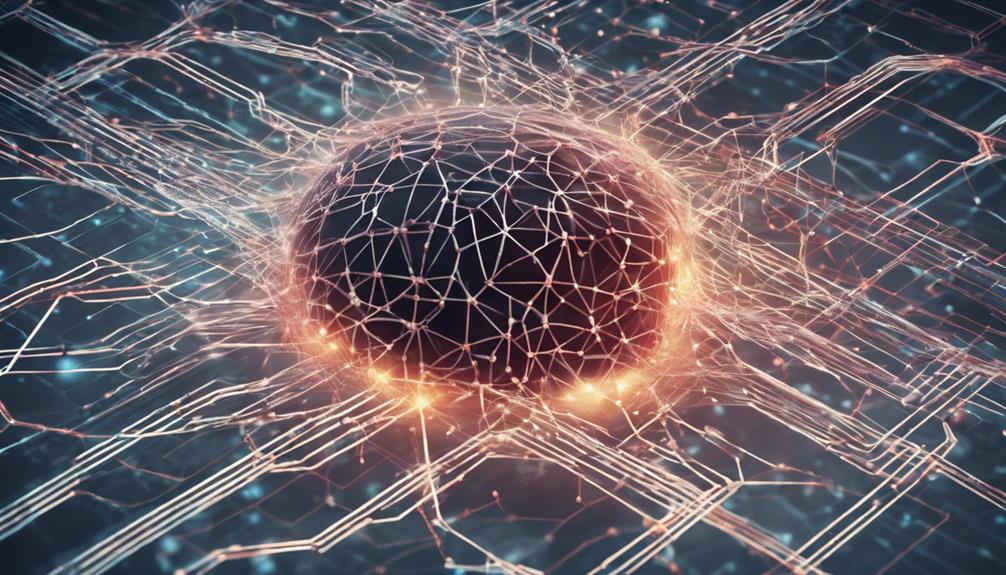
Leveraging generative AI in cybersecurity is poised to revolutionize the future of threat management by enhancing predictive capabilities and enabling proactive defense strategies. As we look ahead, here are key aspects that will shape the future landscape of threat management:
- Advanced Threat Simulation: Generative AI will play a pivotal role in creating sophisticated threat simulations, allowing organizations to anticipate and prepare for evolving cyber threats effectively.
- Predictive Measures: By harnessing generative AI, cybersecurity professionals will be able to predict and prevent potential cyber attacks before they occur, greatly enhancing the overall security posture.
- Human Expertise Integration: Collaborating human expertise with generative AI technologies will be essential to staying ahead of cyber threats. This partnership will leverage the unique strengths of both human analysts and AI systems to establish a robust defense strategy.
The future of threat management will rely on a harmonious blend of cutting-edge technology, human intelligence, and strategic foresight to combat increasingly complex cybersecurity challenges.
Ethical Considerations in AI
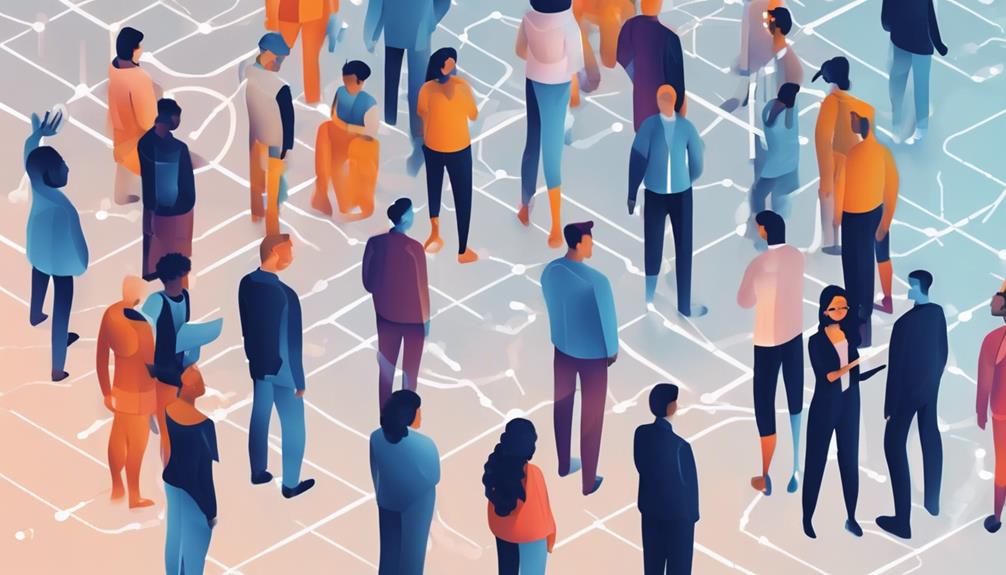
Understanding the ethical considerations in AI is essential for ensuring fair and unbiased cybersecurity practices.
From raising awareness about AI bias to implementing robust privacy protection measures, addressing these concerns is paramount in promoting accountability and transparency.
AI Bias Awareness
An essential aspect of ethical considerations in AI is fostering awareness of AI bias to mitigate potential discriminatory outcomes in decision-making processes. AI bias refers to the unfair outcomes or decisions made by AI systems due to inherent biases in the data used for training.
Unaddressed AI bias can lead to discrimination in hiring, lending, and law enforcement, affecting marginalized groups disproportionately. To guarantee fairness in AI development and prevent biased decisions, ethical considerations emphasize the importance of algorithm auditing, diverse dataset collection, and ongoing monitoring.
By acknowledging and actively addressing AI bias, we can work towards creating more equitable AI systems that serve all individuals fairly. Awareness of AI bias is vital to prevent reinforcing existing societal inequalities and promote ethical AI development practices.
Let's aim for transparency, accountability, and fairness in AI to build a future where technology benefits everyone.
Privacy Protection Measures
To uphold ethical standards in AI development, ensuring robust privacy protection measures is paramount in safeguarding user data and preventing unauthorized access. Implementing encryption techniques and access controls are essential components of maintaining data privacy in AI systems. Striking a delicate balance between data security and AI functionality is important for upholding ethical guidelines and complying with regulations. Regular audits and monitoring of AI algorithms play an important role in identifying and addressing potential privacy vulnerabilities to protect sensitive information.
Privacy Protection Measures | Description | Importance |
---|---|---|
Encryption Techniques | Utilizing encryption to secure sensitive data | Safeguarding information from unauthorized access |
Access Controls | Implementing controls to regulate data access | Preventing unauthorized users from viewing data |
Transparent Data Handling | Practicing transparent data handling for trust | Building user trust and accountability |
Transparent data handling practices and clear communication with users are essential for promoting the ethical use of data and ensuring privacy protection in AI cybersecurity initiatives.
Accountability and Transparency
Establishing clear guidelines for responsible decision-making processes is essential to guarantee accountability in the domain of Artificial Intelligence (AI) ethics. When addressing accountability and transparency in AI, it is important to take into account the following:
- Responsible Use: Ensuring AI systems are utilized ethically and in ways that benefit society while minimizing harm is vital.
- Transparency: Disclosing how AI algorithms function and the data used to train them promotes understanding and trust in the technology.
- Building Trust: Openness about AI systems' capabilities, limitations, and potential risks fosters trust among users and stakeholders.
Case Studies and Applications
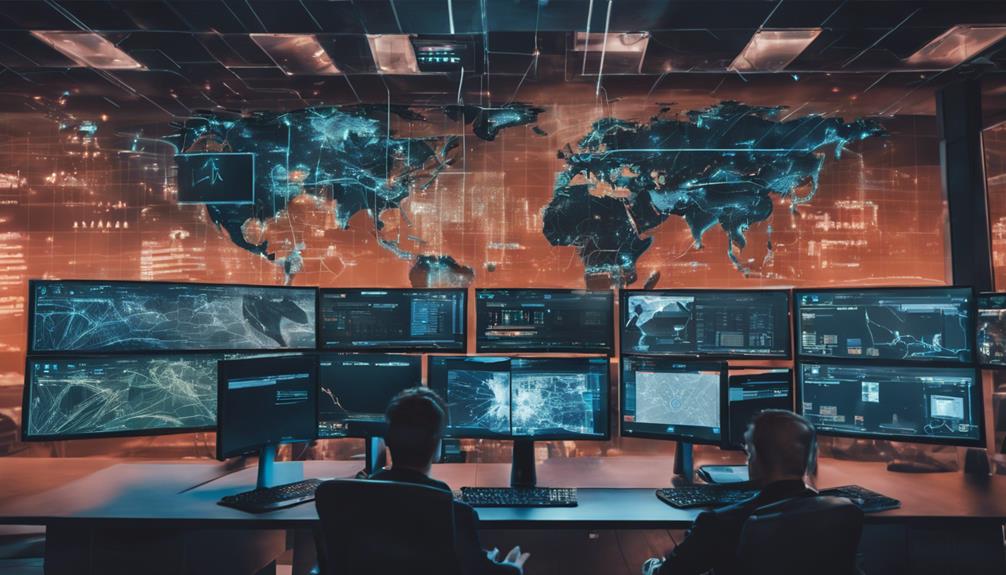
Darktrace's real-time detection of insider threats through generative AI sets a strong foundation for exploring case studies and applications in cybersecurity. Leveraging Generative Adversarial Networks (GANs), companies like Google and IBM Watson have pioneered innovative solutions in threat intelligence and response strategies. By utilizing realistic simulations of potential cyber threats, these technologies enhance model training to adapt to evolving threat landscapes effectively. One notable application is the mitigation of phishing attacks through analyzing user behavior patterns to preemptively identify suspicious activities. This proactive approach not only bolsters cybersecurity defenses but also streamlines incident response procedures. Below is a table highlighting key case studies and applications of generative AI in cybersecurity:
Case Studies and Applications | Description |
---|---|
Insider Threat Detection | Real-time monitoring of employee behavior to identify anomalous actions. |
Automated Response Strategies | Utilizing generative AI for swift and targeted responses to cyber incidents. |
Enhanced Phishing Defense | Analyzing email traffic and communication patterns to thwart phishing attempts. |
Threat Intelligence Analysis | Leveraging generative AI for in-depth analysis of threat landscapes and trends. |
Cybersecurity Risks and Challenges
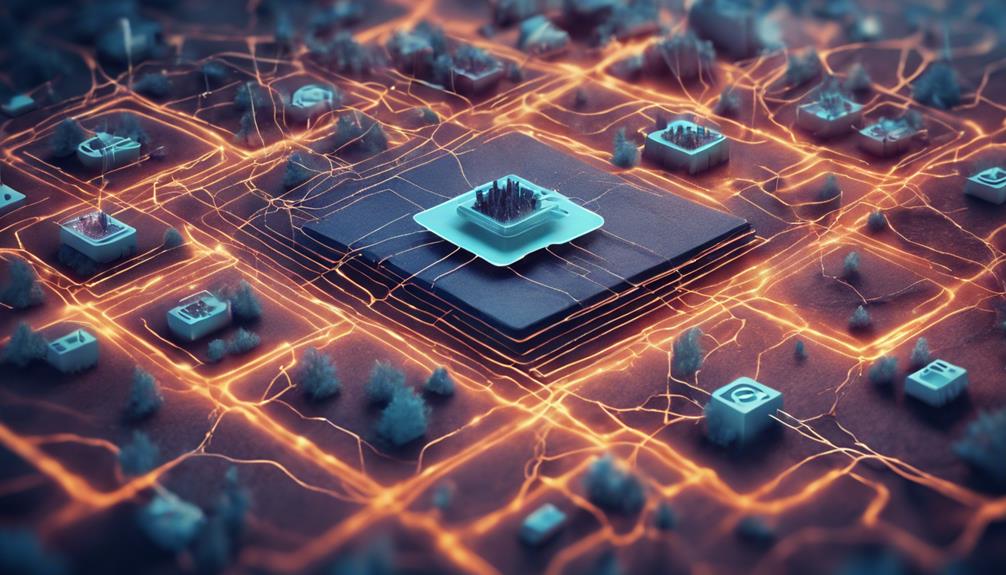
In the domain of cybersecurity, maneuvering through the constantly changing landscape of cyber threats presents a myriad of risks and challenges that organizations must address with vigilance and adaptability.
The evolving cyber landscape demands a proactive approach to combating cyber threats, with traditional security measures proving insufficient in the face of sophisticated attacks. Security policies need to be continuously updated and reinforced to stay ahead of malicious actors.
The challenges of using AI in cybersecurity are evident, as concerns about adversarial attacks on AI systems and data privacy during model training persist. However, recognizing the potential of generative AI in enhancing cybersecurity is essential.
As AI models become more advanced, organizations can leverage them to bolster their defense mechanisms and mitigate the risks posed by evolving cyber threats. Embracing this technology while remaining cautious is key to staying resilient in the ever-evolving cybersecurity landscape.
Frequently Asked Questions
How Is AI Revolutionizing Cyber Security?
AI revolutionizes cybersecurity by automating threat detection and response, offering proactive defense against evolving threats. Through analyzing vast datasets in real-time, AI identifies patterns, anomalies, and malicious activities, outperforming traditional methods for accurate cyber threat detection and prevention.
How Can Generative AI Be Used in Cyber Security?
Generative AI in cybersecurity enhances threat detection by simulating attacks and defenses. It improves anomaly detection in SIEM by analyzing historical data. By predicting and preventing threats, it offers cyber teams a proactive edge against evolving cyber risks.
How to Use Genai in Cyber Security?
In cybersecurity, leveraging generative AI involves employing advanced machine learning techniques like GANs to simulate cyber threats, enhance anomaly detection, automate security measures, and provide scenario-driven training. This approach strengthens defense strategies and improves decision-making in dynamic threat environments.
Can the Integration of AI to Cyber Security Manage System Vulnerability Better?
The integration of AI in cybersecurity greatly enhances the management of system vulnerability by leveraging real-time data analysis to detect anomalies, enabling immediate preventive actions, and offering superior threat detection compared to traditional methods.
Conclusion
In summary, the rise of generative AI in cybersecurity is revolutionizing threat management by enhancing detection capabilities, generating synthetic data, adapting defenses, and simulating realistic attacks.
As we navigate the future of threat management, it is important to contemplate ethical implications and address risks and challenges.
Remember, 'prevention is better than cure.' Stay informed, stay vigilant, and embrace the power of generative AI to protect against evolving cyber threats.