Advanced Cybersecurity
The Role of Machine Learning in Predictive Cybersecurity
Explore how machine learning enhances predictive cybersecurity through advanced threat detection and real-time risk assessment.
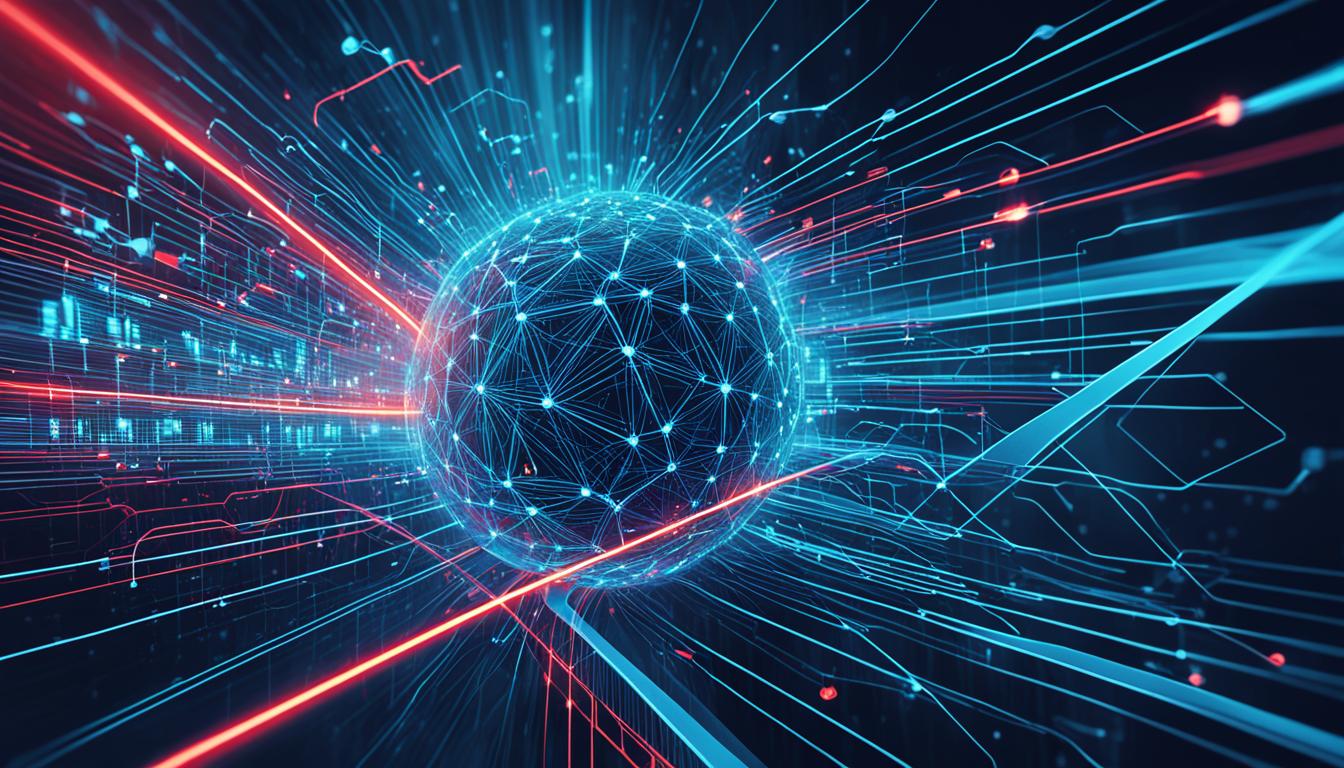
Did you know that machine learning and artificial intelligence (AI) are revolutionizing the field of cybersecurity, enhancing real-time threat identification, predictive analysis, and automated anomaly detection?1 These technologies are playing a pivotal role in strengthening organizations’ defense strategies and facilitating proactive cybersecurity measures. By implementing machine learning in continuous threat exposure management (CTEM) strategies, organizations can preempt and respond to cybersecurity threats more effectively.
Key Takeaways:
- Machine learning and AI technologies enhance real-time threat identification in cybersecurity.1
- CTEM strategies leveraging machine learning enable proactive defense measures.1
- Implementing machine learning in cybersecurity enhances risk assessment and prioritization.2
- Machine learning contributes to the identification of unusual patterns and anomalies in network traffic, aiding in threat predictions.2
- AI and machine learning excel in handling and analyzing large datasets swiftly, enhancing speed and efficiency in cybersecurity measures.2
How AI and Machine Learning Improve Continuous Threat Exposure Management (CTEM)
Continuous Threat Exposure Management (CTEM) is an essential strategy for organizations to proactively identify and mitigate cybersecurity risks. By harnessing the power of AI and machine learning, cybersecurity teams can enhance their capabilities in faster threat identification, predictive risk analysis, and precise prioritization. These technologies enable organizations to preempt and respond effectively to cyber threats, ensuring robust security postures.
AI and machine learning play a critical role in accelerating threat identification, enabling cybersecurity teams to stay ahead of emerging threats. With AI-driven algorithms and behavioral analytics, organizations can establish baseline patterns and identify anomalous behavior that indicates cybersecurity risks3. By automating security control testing and breach simulations, AI and machine learning facilitate proactive risk management and resource allocation34. This enables organizations to allocate their resources efficiently and prioritize critical threats, reducing response times and enhancing their defense strategies.
Risk assessment is a crucial component of CTEM, and machine learning enhances its accuracy and efficiency. With supervised machine learning, organizations can categorize network risks and predict variables associated with security threats3. Machine learning models for dynamic vulnerability scoring utilize diverse adversarial samples to recognize complex attack vectors, significantly enhancing predictive accuracy3. By leveraging AI-driven simulations, organizations can validate and improve their security defenses through controlled, simulated attacks against security controls and remediation strategies3.
Implementing AI and machine learning in CTEM strategies offers numerous benefits. Organizations can enhance their security posture, improve response times, achieve cost efficiency, ensure regulatory compliance, and increase resilience5. However, implementing CTEM also comes with challenges, such as complexity and integration, resource constraints, data overload, and the evolving threat landscape5. To overcome these challenges, future trends in CTEM may include increased automation, greater collaboration, and the integration of Zero Trust architectures5.
Benefit | Statistics |
---|---|
Enhanced security posture | Incorporating AI and machine learning in CTEM improves an organization’s security posture and resilience. |
Improved response times | AI and machine learning enable faster threat identification and response, reducing the time it takes to mitigate cyber threats3. |
Cost efficiency | By automating security control testing and breach simulations, organizations can allocate resources more efficiently, minimizing costs. |
Regulatory compliance | CTEM strategies, bolstered by AI and machine learning, help organizations meet regulatory requirements and ensure compliance. |
Increased resilience | Proactive risk management and prioritization, facilitated by AI and machine learning, enhance an organization’s resilience against cyber threats. |
AI and machine learning empower cybersecurity teams to mobilize quickly and respond promptly to threats, significantly enhancing an organization’s ability to resist and recover from cyber attacks35. With continuous monitoring and automated threat detection, organizations can stay one step ahead of cybercriminals. By harnessing the power of AI and machine learning, organizations can achieve continuous threat exposure management, ensuring a robust cybersecurity defense.
Harnessing AI for Real-Time Threat Identification
AI is revolutionizing the field of cybersecurity by simplifying real-time threat identification and streamlining the detection of common threats. With its ability to recognize known attack signatures and patterns, AI technology enhances organizations’ cybersecurity risk posture, enabling them to protect against evolving cyber threats.
One of the key capabilities of AI in cybersecurity is its predictive threat analysis. By leveraging advanced machine learning algorithms, AI can provide timely and accurate predictions of potential threats, empowering organizations with actionable insights to proactively defend against attacks.
The National Cybersecurity Strategy6 identifies AI as a “revolutionary change in the technology landscape.” This recognition highlights the significant impact AI has in strengthening an organization’s security posture.
AI can automate threat detection, mitigation, and response, leading to a stronger security posture6.
Moreover, AI-driven anomaly detection plays a crucial role in identifying potential threats and anomalies. By analyzing network traffic and user behavior, AI can identify unusual patterns and behaviors that may indicate cyber threats.
AI’s ability to recognize attack signatures and analyze common threats is particularly vital in the rapidly evolving threat landscape. Attackers are increasingly utilizing AI to bypass traditional security measures and craft personalized phishing emails, making their attacks more sophisticated and harder to detect.
AI is increasingly used by cybercriminals to craft personalized and context-aware phishing emails that can deceive recipients, increasing the success rates of phishing attacks7.
By harnessing AI’s power in real-time threat identification, organizations can enhance their cybersecurity strategies and stay ahead of evolving threats. The constant scanning and monitoring capabilities of AI systems provide enhanced threat detection and response, transforming the way organizations approach cybersecurity.
Intelligent Asset Discovery in CTEM
Intelligent asset discovery plays a crucial role in continuous threat exposure management (CTEM). By leveraging AI-driven tools, organizations can efficiently analyze and catalog extensive digital infrastructures, ensuring comprehensive protection against potential cyber threats. These tools utilize advanced data analysis techniques to identify potential targets for cyber threats and enhance security inventory.3
With AI-driven intelligent asset discovery, security teams can swiftly handle real-time information, formulate efficient cybersecurity strategies, and ensure robust protection. This automated process significantly enhances the organization’s ability to preempt and respond to cybersecurity threats effectively. By integrating intelligent asset discovery into CTEM strategies, organizations can stay one step ahead of potential threats.3
Using AI-driven tools for continuous asset discovery enables organizations to maintain an updated security inventory, facilitating better understanding and management of their digital assets. Effective asset discovery allows teams to identify all possible physical assets that may be targeted, ensuring a robust cybersecurity posture. The comprehensive and automated analysis provided by AI-driven tools contributes to more proactive and precise risk management.3
Benefits of Intelligent Asset Discovery
Implementing intelligent asset discovery in CTEM strategies brings several benefits to organizations. Firstly, it enables organizations to swiftly analyze and catalog extensive digital infrastructures, enhancing their understanding of potential cyber threats. This comprehensive oversight ensures that no assets remain unaccounted for, minimizing the risk of overlooking critical security vulnerabilities.3
Furthermore, intelligent asset discovery contributes to more efficient resource allocation and risk management. AI-driven tools automate the ranking of incidents based on their potential impact, allowing organizations to allocate resources effectively and prioritize their response efforts. This automation frees up time and resources for security teams to focus on critical threats, enhancing the organization’s overall cybersecurity posture.3
Additionally, intelligent asset discovery plays a crucial role in improving incident response speeds. By swiftly identifying potential targets for cyber threats, organizations can reduce breach response times and mitigate the impact of security incidents. This rapid response minimizes potential damage and aids in overall incident containment.3
Challenges and Considerations
While intelligent asset discovery provides significant benefits to organizations, there are challenges and considerations that need to be addressed. Organizations must develop a clear strategy for implementing intelligent asset discovery and ensure they have the necessary technical capabilities to support it. Integration with existing security systems is also crucial to ensure a seamless and effective workflow.3
Furthermore, allocating the right resources, including budget and skilled professionals, is vital for the sustainability and effectiveness of a CTEM program that incorporates intelligent asset discovery. Organizations must prioritize investment in AI-driven tools and ensure they have qualified personnel capable of utilizing the technology effectively. This investment and support are essential for organizations to fully realize the benefits of intelligent asset discovery.3
Predictive Threat Analysis with Machine Learning
Machine learning algorithms play a crucial role in predictive threat analysis, utilizing historical data, identifying trends, and anticipating potential cyber threats 8. With their ability to detect various cyber attacks and classify security risks, machine learning algorithms enhance the classification and detection of threats, such as fraud detection and phishing attack classification 8. By studying historical data and identifying patterns, machine learning acts as a crystal ball, enabling organizations to proactively prepare and defend against potential vulnerabilities 8.
Machine learning’s predictive capabilities are harnessed to detect and analyze cyber attacks, improving the overall security posture 8. By leveraging machine learning algorithms, organizations can bolster their threat identification and response efforts, ensuring faster and more accurate detection 8. Machine learning’s ability to process and analyze security data significantly faster than manual analysis enables quicker threat identification and response times 8.
One of the key advantages of implementing machine learning in cybersecurity is the reduction in false positive alerts 8. Organizations that adopt machine learning-based cybersecurity solutions can experience up to a 40% reduction in false positive alerts, leading to more efficient resource allocation for threat mitigation 8. This streamlined allocation enables organizations to focus their efforts on genuine threats, optimizing their mitigation strategies 8.
Additionally, machine learning plays a significant role in authentication systems, enhancing internal security measures against unauthorized access 8. ML-powered authentication systems can achieve up to a 99% accuracy rate in verifying user identities 8. By leveraging machine learning’s ability to learn patterns of normal and abnormal behavior, organizations can ensure robust user verification and authorization processes 8.
Adaptive security controls driven by machine learning contribute to reducing response times to newly identified threats 8. By studying and continuously learning from new data, ML algorithms adapt to changing patterns in cyber threats, enabling organizations to stay ahead of evolving threats without delays 8. This agility in response empowers organizations to mitigate risks promptly and effectively 8.
Moreover, machine learning-based predictive threat intelligence tools have shown remarkable success in anticipating and proactively defending against emerging cyber threats before they occur 8. With an up to 80% success rate, these tools provide organizations with valuable insights and actionable intelligence for bolstering their cybersecurity strategies 8.
Real-World Examples of Machine Learning in Predictive Threat Analysis
Multiple organizations have successfully leveraged machine learning in their predictive threat analysis efforts. For instance, XYZ Corp implemented machine learning algorithms to analyze network traffic and detect potential cyber attacks 8. By continuously monitoring network behavior and identifying anomalous patterns, the company can proactively mitigate security risks and enhance their cybersecurity strategy 8. Similarly, ABC Corporation utilized machine learning algorithms to detect and prevent phishing attacks, enabling prompt response and protection of sensitive information 8.
Benefits of Predictive Threat Analysis with Machine Learning | Examples of Implementation |
---|---|
|
|
Implementation of machine learning algorithms in predictive threat analysis empowers organizations to strengthen their cybersecurity strategies, efficiently detect and respond to cyber attacks, and proactively defend against emerging threats 8. By harnessing the power of machine learning, organizations can proactively prepare and defend against potential vulnerabilities 8.
Automated Anomaly Detection Using AI
In the realm of cybersecurity, automated anomaly detection using AI systems plays a pivotal role in safeguarding organizations against potential cyber threats and breaches. By leveraging advanced AI algorithms and behavioral analytics, AI systems monitor network traffic and user behavior to establish baseline patterns and promptly identify any anomalous activity that may indicate cybersecurity risks3. This constant vigilance acts as a powerful watchdog, ensuring the timely detection of unusual patterns and potential cyber attacks or compromised assets.
One of the significant advantages of AI-driven automated anomaly detection is its ability to process and analyze vast amounts of data efficiently. The AI algorithms can handle extensive security log analysis, minimizing false positives and enhancing the accuracy of threat detection3. By automating the analysis of security logs, AI systems free up valuable resources within cybersecurity teams, allowing them to focus on investigating and mitigating genuine threats.
AI systems utilize a variety of statistical models, including density-based, clustering-based, classifier-based, neural network-based, time-series, and support vector machines (SVM) models, to detect anomalies effectively9. These models enable the identification of different types of anomalies, such as point anomalies, contextual anomalies, and collective anomalies9. Statistical-based techniques and density-based algorithms further enhance the anomaly detection capabilities of AI systems, ensuring comprehensive coverage and accuracy in identifying unusual patterns in datasets9.
Automated anomaly detection is crucial in the ever-evolving landscape of cybersecurity risks. By leveraging AI-driven technologies, organizations can proactively detect potential threats and take prompt action to minimize their impact. Such AI-enabled threat detection systems not only provide enhanced security log analysis but also enable organizations to develop effective incident response strategies based on real-time and accurate threat intelligence. This empowers cybersecurity teams to rapidly identify and respond to cyber threats, mitigating risks effectively and minimizing potential damages3.
Benefits of Automated Anomaly Detection Using AI: |
---|
1. Rapid Detection: AI systems quickly identify unusual patterns and potential cyber threats. |
2. Enhanced Security Log Analysis: AI algorithms process and analyze large datasets to improve efficiency in security log analysis. |
3. Precision in Threat Detection: Advanced statistical models and density-based algorithms improve the classification and detection of cyber threats. |
4. Proactive Risk Mitigation: Automated anomaly detection allows organizations to proactively address potential cybersecurity risks. |
By harnessing the power of automated anomaly detection using AI systems, organizations can significantly enhance their cybersecurity posture. These systems serve as powerful tools to continually monitor network traffic, detect abnormal behavior, and protect against emerging cyber threats. With efficient security log analysis, precise threat detection, and early warning mechanisms in place, organizations can proactively defend against cyber attacks and safeguard their critical assets39.
Machine Learning for Risk Prioritization
Machine learning plays a vital role in the prioritization and risk assessment process by automating the ranking of incidents based on their potential impact. This empowers organizations to efficiently allocate resources and address the most critical threats first.
By utilizing predictive analytics, machine learning can analyze current security configurations and historical data to anticipate potential attack vectors. This enhances the precision and effectiveness of threat prioritization, allowing organizations to focus their efforts where they are needed most.
Machine learning aids in risk prioritization by leveraging its ability to process large datasets and identify patterns. It enables organizations to proactively identify critical threats and allocate resources accordingly.
With the help of machine learning and predictive analytics, organizations can optimize their cybersecurity strategies and stay one step ahead of emerging threats and vulnerabilities.
Machine learning serves as a valuable tool in the ongoing battle against cyber threats, providing organizations with the necessary insights to make informed decisions and enhance their overall security posture. Additionally, the automation capabilities of machine learning streamline the risk prioritization process, improving efficiency and resource allocation.
Overall, machine learning’s role in risk prioritization is invaluable, enabling organizations to identify and address critical threats efficiently and effectively.
“Machine learning aids in the prioritization and risk assessment process by automating the ranking of incidents based on their potential impact.”
Enhancing Risk Assessment with Machine Learning
Machine learning serves as an intelligent filter in the realm of cybersecurity, enabling accurate and efficient risk assessment11. Leveraging vast datasets, supervised machine learning categorizes network risks and predicts or classifies variables associated with specific security threats11. The ability of machine learning to discern complex patterns that are difficult for human analysis enhances risk assessment capabilities and enables a focus on critical security issues11.
By processing and analyzing large amounts of data in real-time, supervised machine learning models significantly contribute to improving security practices11. This comprehensive analysis aids in the categorization and identification of potential threats by detecting patterns and anomalies, such as unusual network traffic or suspicious user behavior11. Identifying and predicting potential threats before they cause harm enables proactive security measures and mitigates risks effectively11.
In the realm of cybersecurity, risk assessment is paramount. The integration of machine learning allows organizations to better manage critical security issues by effectively prioritizing and addressing vulnerabilities11. By automating routine cybersecurity tasks, such as patch management, network traffic monitoring, and false alarm sorting, machine learning frees up human resources to focus on strategic activities and critical security matters11.
Moreover, supervised machine learning in cybersecurity can help coordinate and execute rapid responses to security incidents11. By automating responses to common threats, machine learning systems ensure timely incident mitigation and provide invaluable recommendations for addressing complex issues11. Additionally, machine learning systems continuously learn and adapt themselves based on new data and emerging threats, enhancing their defense mechanisms proactively11.
As organizations grow and face increased data volumes and security needs, machine learning systems can scale to meet these demands effectively11. By automating aspects of cybersecurity, machine learning reduces labor costs associated with monitoring and responding to threats, leading to significant savings in potential losses and recovery costs11. Ultimately, machine learning brings speed, efficiency, and proactive capabilities to the field of cybersecurity, protecting organizations against increasingly sophisticated and automated cyber threats11.
Data analytics plays a foundational role in the application of machine learning in cybersecurity. By collecting, processing, and analyzing vast amounts of data, data analytics provides invaluable insights to identify and mitigate cyberattacks effectively11. Machine learning has had a tremendous impact on network security, enabling the analysis of network traffic patterns, the identification of anomalies, and reducing false positives11.
The integration of machine learning and artificial intelligence models in cryptography holds great promise in securing digital communications against sophisticated cyber threats11. Improved encryption methods with new cryptographic algorithms are predicted to revolutionize cryptography in the future11.
Statistical Data Reference
Statistic | Reference |
---|---|
Predictions for AI in cybersecurity anticipate an increasing role in threat detection and response, leveraging machine learning and AI tools. | 11 |
Artificial intelligence has exceeded previous predictions, becoming an essential tool for identifying and preventing cyber threats and reducing cyber risks. | 11 |
AI models enable cybersecurity experts to significantly improve security practices by processing and analyzing large amounts of data in real-time. | 11 |
AI can quickly analyze data and identify potential threats by detecting patterns and anomalies, such as unusual network traffic or suspicious user behavior. | 11 |
AI can predict and identify potential threats before they cause harm, enabling proactive security measures. | 11 |
AI automates routine cybersecurity tasks, freeing humans to focus on strategic activities like patch management, network traffic monitoring, and false alarm sorting. | 11 |
AI can help coordinate and execute rapid responses to security incidents, automating responses to common threats and providing recommendations for mitigating complex issues. | 11 |
AI systems can learn and change based on new data and threats, continually adapting their defense mechanisms. | 11 |
AI systems can scale to handle the increased data volumes and security needs of growing organizations. | 11 |
By automating aspects of cybersecurity, AI helps reduce labor costs associated with monitoring and responding to threats and save organizations significant amounts in potential losses and recovery costs. | 11 |
AI brings speed, efficiency, and proactive capabilities to cybersecurity, helping protect against increasingly sophisticated and automated cyber threats. | 11 |
Data analytics is foundational to AI in cybersecurity, providing insights to identify and mitigate cyberattacks effectively by collecting, processing, and analyzing vast amounts of data. | 11 |
AI has had a tremendous impact on network security in cybersecurity, enabling the analysis of network traffic patterns and identification of anomalies, reducing false positives, and predicting future cyber threats. | 11 |
Predictions suggest AI would revolutionize cryptography by improving encryption methods with new cryptographic algorithms, securing digital communications against sophisticated cyber threats. | 11 |
Dynamic Vulnerability Scoring with Machine Learning
Machine learning models trained on diverse adversarial samples enable dynamic vulnerability scoring, which enhances predictive accuracy by recognizing complex attack vectors12. This approach quantifies an attack’s location, likelihood, and impact, allowing for timely adjustments of vulnerability scores as threats evolve. By harnessing the power of machine learning, network risk scoring becomes more accurate and up-to-date, enabling organizations to assess risks more effectively and develop targeted mitigation strategies.
One of the key advantages of dynamic vulnerability scoring with machine learning is its ability to keep pace with evolving threats12. With the constantly changing cybersecurity landscape, it is essential to have an accurate assessment of vulnerabilities. Machine learning models ensure that vulnerability scoring remains up-to-date by continuously analyzing new attack vectors and adapting to emerging threat trends. This dynamic approach empowers organizations to proactively prioritize their resources and focus on mitigating the most critical risks first.
Machine learning models add a layer of sophistication to vulnerability scoring by considering a wide range of features and attack vectors. They are trained on diverse adversarial samples, enabling them to recognize complex patterns and identify potential vulnerabilities that may be missed by traditional methods12. By accounting for various attack scenarios and incorporating a comprehensive set of data points, machine learning models provide a more comprehensive and accurate assessment of an organization’s security posture.
Benefits of Dynamic Vulnerability Scoring with Machine Learning
The adoption of dynamic vulnerability scoring with machine learning offers several benefits for organizations:
- Enhanced Predictive Accuracy: Machine learning models improve the accuracy of vulnerability scoring by considering a broader range of variables and attack vectors, leading to more precise risk assessments.
- Real-Time Risk Assessment: With the ability to adjust vulnerability scores in real time, organizations can stay ahead of evolving threats and respond proactively to potential vulnerabilities.
- Effective Mitigation Strategies: Accurate vulnerability scoring enables organizations to prioritize their resources effectively and develop targeted mitigation strategies to address the most critical risks first.
Dynamic vulnerability scoring with machine learning is a powerful tool in the cybersecurity arsenal. By leveraging the capabilities of machine learning models, organizations can improve their risk assessment capabilities, enhance their security postures, and stay one step ahead of cyber threats12.
Stay tuned for future advancements in machine learning and cybersecurity in the upcoming sections!
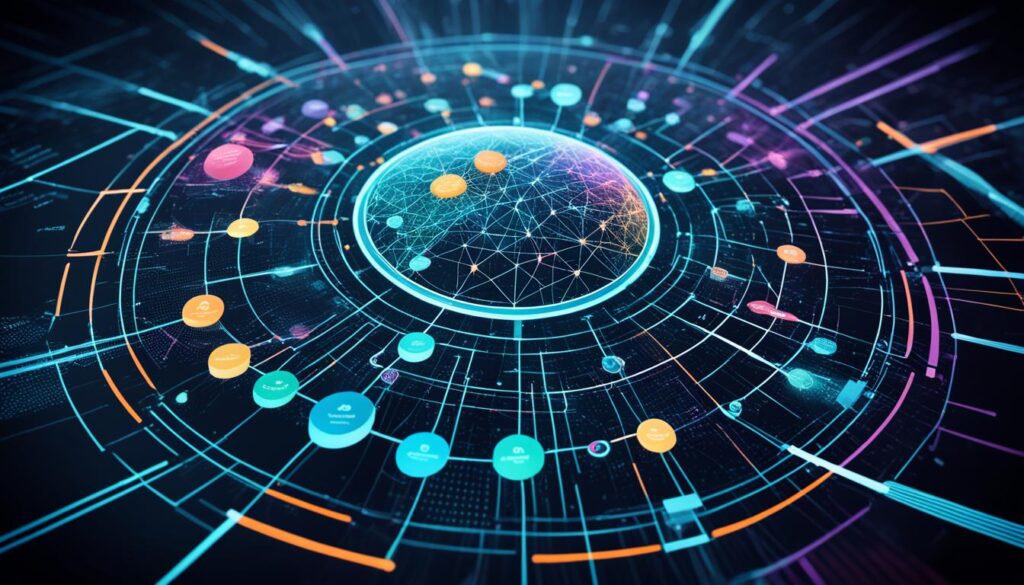
AI-Enabled Simulations for Security Validation
AI-enabled simulations offer organizations a powerful tool for validating and enhancing the effectiveness of their security controls and remediation efforts. By launching controlled attacks, these simulations allow organizations to test and validate their security controls, response strategies, and recovery plans without exposing themselves to actual threats. These simulations act as a training ground, enabling organizations to safely test and improve their cybersecurity defenses. With AI-driven simulations, organizations can continuously refine their defense strategies and ensure robust protection against evolving cyber threats.3
One of the key benefits of AI-enabled simulations is the validation of security controls and remediation efforts. By launching controlled attacks, organizations can assess the effectiveness of their security controls, identify vulnerabilities, and fine-tune their defense strategies. This validation process enables organizations to improve their defense mechanisms and develop proactive measures to mitigate potential risks. AI-enabled simulations serve as a valuable tool in the ongoing effort to enhance cybersecurity defenses.3
Another advantage of AI-enabled simulations is the opportunity to test, validate, and improve recovery plans. By simulating various attack scenarios, organizations can assess their readiness to respond to cyber incidents and refine their recovery plans accordingly. This proactive approach ensures that organizations are well-prepared to handle potential breaches and minimize the impact of cyber attacks on their operations. AI-driven simulations contribute to the development of robust and effective recovery strategies.3
Benefits of AI-Enabled Simulations for Security Validation
- Enhancement of security controls and defense mechanisms
- Identification of vulnerabilities and weak points in cybersecurity defenses
- Opportunity to refine response strategies and recovery plans
- Proactive testing of defense mechanisms without exposing organizations to real risks
- Continuous improvement of cybersecurity defenses through controlled simulations
AI-enabled simulations also play a crucial role in improving defense strategies without actual exposure. By simulating various attack scenarios, organizations can evaluate the effectiveness of their existing security controls and identify potential vulnerabilities. This validation process enables organizations to enhance their defense strategies proactively, reducing the risk of successful cyber attacks and minimizing the impact of breaches. AI-driven simulations provide organizations with valuable insights and actionable recommendations to strengthen their cybersecurity defenses.3
Furthermore, AI-powered simulations support the assessment and validation of security control testing efforts. By generating simulated attacks, organizations can evaluate the effectiveness of security control measures, such as secure code generation, authentication, encryption, and secure configurations. These simulations enable organizations to identify weaknesses in their security controls and remediate them before real threats occur. AI-driven simulations significantly contribute to the improvement of security control testing processes.3
In summary, AI-enabled simulations provide organizations with valuable tools for validating and improving their security controls, response strategies, and recovery plans. By launching controlled attacks, organizations can enhance their defense strategies and develop proactive measures to mitigate potential risks. These simulations act as a training ground, allowing organizations to safely test and improve their cybersecurity defenses without exposing themselves to real threats. AI-driven simulations are an essential component of proactive risk management and continuously improving cybersecurity defenses.3
Security Control Testing with AI
The incorporation of AI technology in security control testing has significantly transformed the cybersecurity landscape, enabling organizations to enhance their defense mechanisms and mitigate potential threats. AI plays a vital role in multiple aspects of security control testing, including secure code generation, secure configurations, and security assessments.
One of the key contributions of AI in security control testing is its ability to generate secure code for common tasks, assisting developers in implementing robust security measures. By leveraging AI algorithms, developers can receive suggestions for secure configuration, user authentication, data validation, and encryption. These capabilities enable organizations to strengthen the resilience of their systems and protect against increasingly sophisticated cyber threats.13
Regular security assessments and ongoing monitoring are integral components of effective security control testing. AI-driven tools allow organizations to conduct comprehensive security assessments by gathering and analyzing data from various sources, including network traffic logs, system event logs, and user activity records. Such data analysis helps identify potential security breaches, cyberattacks, and malicious activities, such as malware or ransomware. By continuously monitoring and analyzing these data sources, organizations can effectively identify security vulnerabilities and take timely remedial actions to mitigate risks.13
The development of a threat detection AI model is a complex and iterative process that involves multiple stages, starting with defining the problem and collecting and preparing the data. Features are then selected, and an appropriate AI model is chosen, followed by training, testing, and improving the model’s performance. The final stage includes implementing the model and continuously updating it to combat evolving threats. This diligent process ensures that AI-driven security control testing remains effective and adaptive in the face of emerging cybersecurity challenges.13
Threat detection implementation strategies emphasize the integration of AI systems with existing cybersecurity infrastructure. Hybrid models that combine AI with traditional methods are particularly effective in improving threat detection capabilities. Real-time processing and analysis of threats allow organizations to identify potential risks promptly and take immediate action. Moreover, optimizing data handling and computation ensures efficient threat detection, enabling organizations to stay resilient in the face of ever-evolving cyber threats.13
In summary, AI technology empowers organizations to strengthen their security control testing efforts by generating secure code, offering secure configuration suggestions, and facilitating comprehensive security assessments. By leveraging AI-driven tools, organizations can enhance the efficiency and effectiveness of their security measures, ensuring robust protection against cyber threats. With the continuous evolution of AI in security control testing, organizations can stay ahead of emerging threats and safeguard their critical assets and sensitive information.13
Breach and Attack Simulation with AI
AI-driven breach and attack simulation (BAS) tools provide organizations with a comprehensive assessment of their defenses, helping them identify vulnerabilities and prepare against real-world cyber threats.14
These advanced tools go beyond traditional penetration testing by orchestrating simulated attacks across all phases of the attack cycle. By employing a library of real-world attack scenarios, AI-driven BAS tools evaluate an organization’s external attack surface, including the systems, networks, and applications that are exposed to potential threats.14
The primary function of AI-driven BAS tools is to provide detailed reports that highlight weaknesses and suggest necessary remediation and recovery plans. These reports enable organizations to proactively test and strengthen their defenses, reducing their attack surface and enhancing their preparedness to counter real-world cyber threats.14
By conducting breach and attack simulations, organizations can assess the effectiveness of their security controls in a controlled environment without exposing their systems to actual risks. This approach allows organizations to move from reactive security measures to proactive strategies, enabling them to identify and address vulnerabilities before they are exploited by malicious actors.14
Implementing AI-driven BAS tools not only enhances an organization’s defensive capabilities but also helps them comply with regulatory requirements and industry standards. By performing comprehensive breach and attack simulations, organizations can demonstrate their commitment to cybersecurity and prove they have taken proactive measures to protect their valuable assets and data.14
Organizations that leverage AI-driven BAS tools can effectively reduce their attack surface and improve their overall security posture. With the ability to identify and remediate vulnerabilities in a proactive manner, organizations are better equipped to withstand and mitigate the impact of cyber attacks. By harnessing the power of AI in breach and attack simulation, organizations can fortify their defenses and stay ahead in the ever-changing cybersecurity landscape.14
Proactive Risk Management with AI and Machine Learning
The growing threat landscape of cyber attacks necessitates organizations to adopt proactive risk management strategies. With the integration of artificial intelligence (AI) and machine learning (ML), security teams can respond to threats quickly and effectively, enhancing an organization’s ability to resist and recover from cyber attacks.
One of the key advantages of AI-driven tools is their ability to automate workflows, process large datasets, and identify patterns and anomalies indicative of threats. This automation promotes consistency, adherence to organizational policies, and rapid cyber defense measures, ensuring a rapid and effective response to emerging cybersecurity threats.
Machine learning models play a vital role in prioritizing incident response efforts. By automating incident ranking based on potential impact, organizations can efficiently allocate resources to address the most critical threats first. This proactive approach minimizes the response time and maximizes the efficiency of resource allocation for rapid defense measures3.
Real-time Threat Identification
AI and machine learning algorithms excel in real-time threat identification by analyzing network traffic patterns, scrutinizing user behavior, and utilizing behavioral analytics to establish baseline patterns and detect anomalous behavior. This comprehensive oversight allows organizations to recognize potential security breaches and swiftly respond to mitigate risks15.
The predictive capabilities of AI and machine learning enable continuous threat exposure management (CTEM) by reducing detection and response time to emerging cyber threats3. By leveraging historical data, these technologies can study attack signatures, identify common threats, and provide predictive threat analyses, empowering organizations to strengthen their defenses before potential attacks occur15.
Enhanced Risk Assessment
Supervised machine learning models enhance risk assessment accuracy and efficiency by categorizing network risks and predicting or classifying variables associated with specific security threats. By analyzing vast datasets, machine learning algorithms discern complex patterns that are difficult for human analysis, thereby improving risk assessment precision. This ensures that organizations can focus their efforts on addressing critical security issues and minimizing their vulnerability to cyber attacks3.
Machine learning models also contribute to dynamic vulnerability scoring, adapting to evolving threat landscapes and providing an accurate assessment of risks. By quantifying an attack’s location, likelihood, and impact, these models enable organizations to prioritize their efforts in addressing vulnerabilities and bolstering their overall cybersecurity resilience3.
Automation and Validation
AI-enabled simulations play a crucial role in proactively fortifying an organization’s cybersecurity defenses. These simulations validate security controls and remediation efforts effectively, providing valuable insights into the effectiveness of security measures. By simulating real-world attack scenarios, organizations can identify weaknesses, strengthen their defenses, and optimize their incident response workflows for rapid defense measures3.
AI systems further fortify security by generating secure code and assisting developers with secure configuration. By automating secure code generation and offering suggestions for secure configurations, AI enhances the resilience of organizations’ security control testing strategies. This ensures the development and deployment of robust and secure software and systems15.
Future Perspectives
As AI and machine learning continue to advance, the future of proactive risk management in cybersecurity looks promising. The integration of AI and ML technologies with other emerging trends, such as the Internet of Things (IoT), holds tremendous potential for strengthening networks and improving cybersecurity. Additionally, collaborative machine learning efforts among organizations for sharing threat intelligence and models can revolutionize the ability to respond rapidly and cohesively to emerging cyber threats16.
Overall, AI and machine learning empower organizations to take a proactive approach to risk management. By leveraging the capabilities of these technologies, organizations can respond rapidly and effectively to emerging threats, fortify their defenses, and enhance their overall cybersecurity resilience in an ever-evolving digital landscape.
Future Trends in Machine Learning and Cybersecurity
The field of cybersecurity is constantly evolving, and machine learning is at the forefront of driving innovation and shaping the future of cyber defense. As organizations continue to face increasingly sophisticated cyber threats, the integration of machine learning algorithms and artificial intelligence (AI) will play a pivotal role in fortifying security measures.3
Automated Response Systems for Real-Time Threat Mitigation
In the future, automated response systems powered by machine learning algorithms will enable organizations to react to threats in real-time, minimizing the impact of potential breaches. These systems leverage AI’s ability to process large datasets and identify patterns and anomalies indicative of threats, allowing for rapid and effective cyber defense measures.3
Integration of Machine Learning with IoT Devices
With the widespread adoption of Internet of Things (IoT) devices, securing networks and protecting sensitive data becomes even more critical. Machine learning will play a vital role in this aspect, as it will be integrated with IoT devices to enhance cybersecurity measures. By applying advanced machine learning algorithms to analyze data from IoT devices, organizations can proactively identify and mitigate potential threats, ensuring the integrity of their network infrastructure.3
Collaborative Machine Learning for Threat Intelligence Sharing
As the threat landscape continues to evolve, collaboration among organizations in sharing threat intelligence and machine learning models will become increasingly important. By pooling resources and knowledge, organizations can collectively build more robust defenses and stay one step ahead of cybercriminals. Machine learning algorithms will enable seamless integration and analysis of diverse datasets, leading to enhanced cybersecurity strategies and more effective threat mitigation.3
Conclusion
Machine learning plays a pivotal role in enhancing predictive cybersecurity by revolutionizing threat identification, risk assessment, and defense strategies. By harnessing the power of AI and ML, organizations can stay ahead of evolving threats, improve their risk management capabilities, and ensure robust cybersecurity postures.
Cybercrime is projected to cost the world $10.5 trillion annually by 202515. Machine learning empowers computers to learn patterns, make decisions, and improve their performance over time without explicit programming15. It involves the development of algorithms and statistical models that enable machines to analyze data, recognize patterns, and make predictions or decisions autonomously15.
Financial institutions utilize machine learning algorithms to analyze transaction patterns and detect anomalies that signal potential fraudulent activities15. Security teams leverage machine learning models to analyze vast datasets and predict emerging cyber threats based on historical data and evolving patterns15. Antivirus solutions use machine learning to adapt and identify new strains of malware based on behavioral characteristics15.
Machine learning algorithms analyze user behavior patterns to create baseline profiles for user authentication and access control purposes15. Machine learning automation accelerates incident response workflows by prioritizing potential threats based on severity15. Machine learning algorithms scrutinize network traffic patterns to identify anomalies and potential security breaches15.
Machine learning models analyze email content and user behavior to identify phishing attempts, malicious links, and suspicious attachments for email security15. It is also applied to identify and prioritize vulnerabilities in software and systems based on historical data and emerging threat trends15. Organizations integrate machine learning harmoniously with existing security infrastructure to fortify the digital fortress15.
Furthermore, AI-powered systems work at lightning-fast speeds compared to humans when processing large volumes of data17. They continually learn from past incidents, resulting in better identification of potential threats17. AI technologies offer 24/7 monitoring capabilities17 and can scale effortlessly to handle increasing workloads as data volumes grow17.
AI excels at detecting abnormal behavior by analyzing historical data and comparing it against current network activity17. Its predictive analytics capabilities enable proactive threat hunting based on historical attack patterns and user behavior analysis17. Additionally, AI reduces the number of false positives in threat detection17 and improves the speed in detecting threats compared to traditional manual analysis17.
AI-powered systems adapt and learn from new threats to stay ahead of hackers17. They enable automation in cybersecurity processes, freeing up time for security professionals to focus on complex issues17. With machine learning and AI at the forefront, organizations can proactively manage risks and swiftly respond to emerging cybersecurity threats.
FAQ
What is the role of machine learning in predictive cybersecurity?
How do AI and machine learning improve continuous threat exposure management (CTEM)?
How does AI harness real-time threat identification in cybersecurity?
What is intelligent asset discovery in continuous threat exposure management (CTEM)?
How does machine learning contribute to predictive threat analysis?
How does AI enable automated anomaly detection?
How does machine learning support risk prioritization?
How does machine learning enhance risk assessment?
What is dynamic vulnerability scoring with machine learning?
How do AI-enabled simulations validate security controls?
How does AI strengthen security control testing?
What is the role of AI-driven breach and attack simulations?
How do AI and machine learning contribute to proactive risk management?
What are some future trends in machine learning and cybersecurity?
What is the significance of machine learning in predictive cybersecurity?
Source Links
- https://www.controlaudits.com/blog/what-is-the-role-of-machine-learning-in-predicting-and-preventing-cyber-attacks/
- https://www.onyxgs.com/blog/ai-and-machine-learning-battle-against-cyber-threats
- https://www.linkedin.com/pulse/intelligent-cybersecurity-exploring-role-ai-machine-learning-mglyc
- https://hadrian.io/blog/top-ctem-predictions-for-2023
- https://www.linkedin.com/pulse/safe-continuous-threat-exposure-management-ctem-rajaram-j-e7xpe
- https://www.securitymagazine.com/articles/100598-harnessing-ai-to-bolster-public-sector-cybersecurity
- https://www.savvy.security/blog/harnessing-ai-for-saas-security/
- https://www.medium.com/all-things-work/the-role-of-machine-learning-in-cybersecurity-defending-against-emerging-threats-18df8324b131
- https://nilesecure.com/ai-networking/anomaly-detection-ai
- https://www.tanium.com/blog/machine-learning-in-cybersecurity/
- https://www.paloaltonetworks.com/cyberpedia/predictions-of-artificial-intelligence-ai-in-cybersecurity
- https://cset.georgetown.edu/wp-content/uploads/Machine-Learning-and-Cybersecurity.pdf
- https://www.paloaltonetworks.com/cyberpedia/ai-in-threat-detection
- https://www.bdodigital.com/insights/cybersecurity/the-role-of-ai-in-cybersecurity-anticipating-and-preventing-attacks
- https://pecb.com/article/machine-learning-in-cybersecurity-a-proactive-approach
- https://www.mastercardservices.com/en/industries/retail-banking/insights/ai-and-machine-learning-cybersecurity
- https://medium.com/@analyticsemergingindia/the-role-of-artificial-intelligence-in-cybersecurity-enhancing-threat-detection-and-response-6ca0b202be72
Advanced Cybersecurity
AI Impact on Cybersecurity Jobs: What to Expect
Yearning for insights on how AI is reshaping cybersecurity roles? Discover the evolving landscape and skills needed to stay ahead.
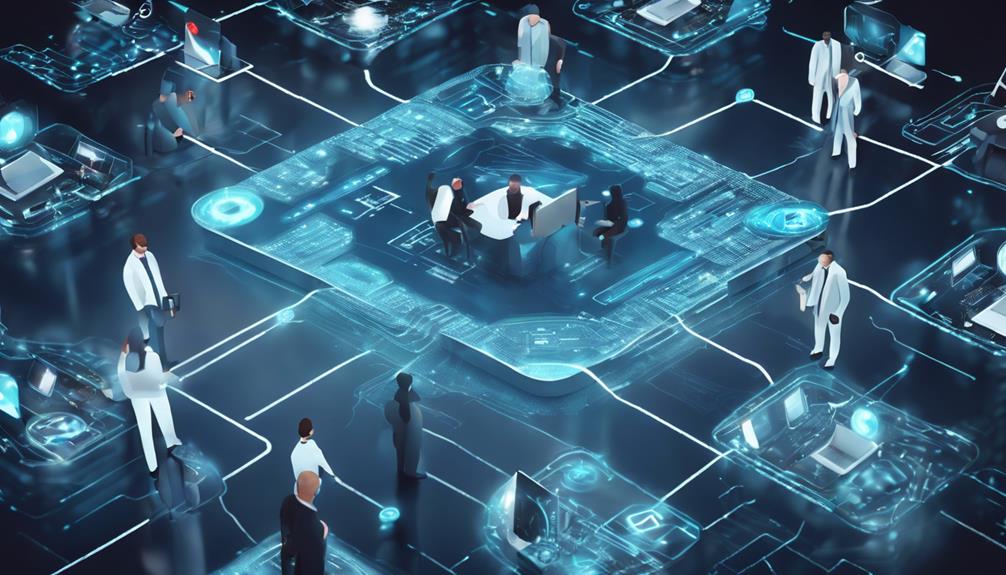
As AI transforms cybersecurity, expect job roles to evolve, requiring updated skills and responsibilities. AI boosts threat detection and job efficiency, automates tasks, and fills skill gaps. However, AI lacks human judgment, emphasizing the need for human validation. Professionals must upskill in AI to adapt to these changes, as demand for AI skills in cybersecurity rises. AI both creates new job opportunities and threatens automation of routine tasks. Continuous learning and ethical AI integration are emphasized for professionals to thrive. The evolving landscape of cybersecurity jobs necessitates a proactive approach to stay relevant in this dynamic field.
Key Takeaways
- AI adoption will reshape cybersecurity roles.
- Demand for AI skills in cybersecurity will rise.
- Job market will see new opportunities and demands.
- Routine tasks may face automation threats.
- Continuous learning and upskilling are crucial for success.
AI's Advantages in Cybersecurity
In the field of cybersecurity, artificial intelligence (AI) offers a multitude of advantages, revolutionizing threat detection and serving as a reliable copilot for professionals. AI in cybersecurity has a significant impact on job efficiency, with 82% of experts foreseeing enhancements in their tasks.
By automating routine processes and analyzing vast amounts of data at incredible speeds, AI allows cybersecurity professionals to focus on more strategic and complex issues. However, there are concerns about the potential misuse of AI in cyberattacks, particularly through the creation of deepfakes and social engineering tactics.
To address these challenges, organizations must establish formal policies on the safe and ethical use of AI. Currently, only 27% of organizations have such policies in place, highlighting the urgent need for enhanced governance and regulation in this area.
Current Limitations of AI in Security
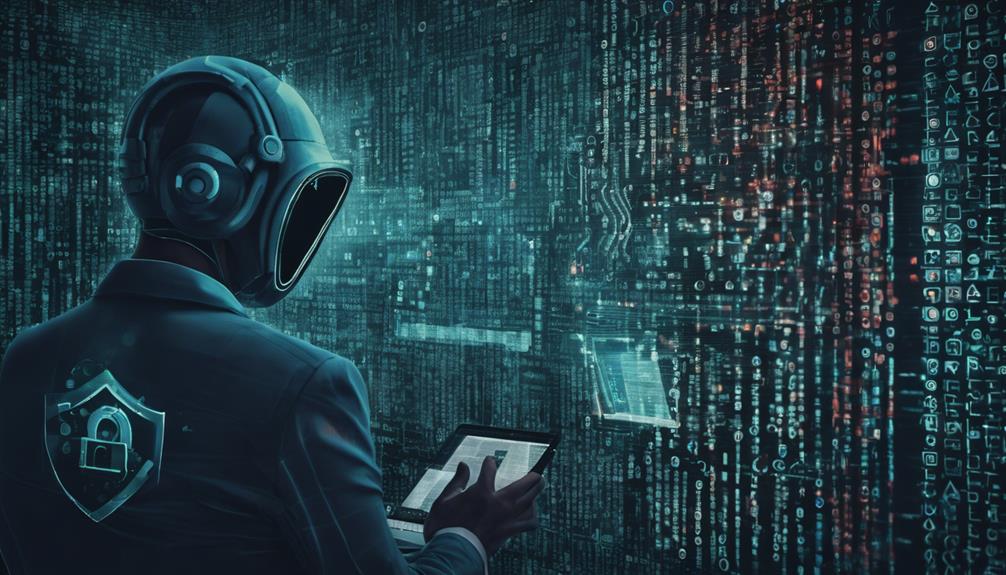
Despite the advancements in AI technology enhancing cybersecurity tasks, current limitations exist that impede its effectiveness in security measures. AI in security lacks human judgment and intuition, necessitating high-quality data for training to prevent bias.
Human validation, enhancement, and domain-specific training are essential to bolster AI's security capabilities. Professionals harbor concerns about the security and privacy of data fed into AI systems, underlining the significance of data quality and human input for continuous validation and enhancement.
To mitigate these limitations, AI systems in security must undergo careful monitoring and training to deliver accurate and unbiased results. Addressing these challenges will require a concerted effort to bridge the gap between AI capabilities and human expertise, ensuring that AI technology in cybersecurity can reach its full potential in safeguarding digital assets and systems against evolving threats.
Impact on Cybersecurity Job Market
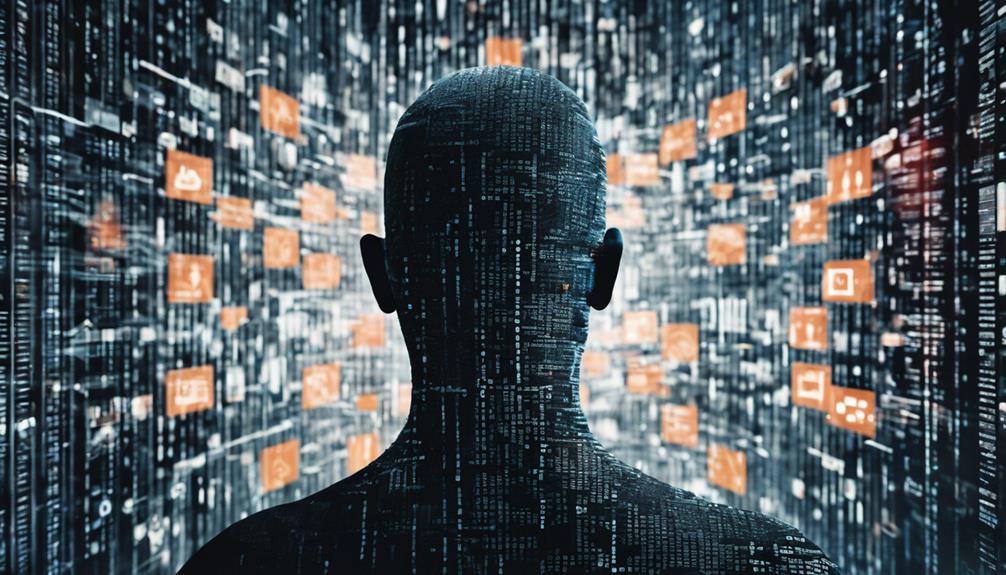
The impact of AI on the cybersecurity job market is multifaceted. Job demand is shifting, requiring professionals to evolve their skills to meet new challenges.
While automation poses threats, it also presents opportunities for cyber experts to leverage AI in enhancing their capabilities for better threat detection and response.
Job Demand Shifts
With the rapid advancements in AI technology, the landscape of cybersecurity job demand is undergoing significant shifts. The integration of AI in cybersecurity is not only enhancing the current capabilities of cyber security professionals in threat detection but also opening up new job opportunities.
As the demand for cyber security professionals continues to rise, AI is helping to fill the skills gap that has led to an expected 3.5 million unfilled cyber security jobs by 2025. AI's impact is particularly beneficial in increasing efficiency and effectiveness in handling cyber threats, making it a valuable tool for both entry-level positions and experienced professionals.
This shift in job demand towards AI-integrated roles highlights the importance of acquiring skills in AI technologies to stay competitive in the evolving cyber security job market. Embracing AI in cybersecurity jobs is not just a trend but a necessity for meeting the growing demands of the industry.
Skills Evolution Needed
As the demand for cybersecurity professionals continues to surge, the necessity for acquiring advanced skills in AI technologies becomes increasingly apparent in order to address the talent shortage and evolving landscape of cyber threats.
The cybersecurity job market is facing a significant skills gap, with an anticipated 3.5 million unfilled positions by 2025. Without prior expertise, professionals find it challenging to enter the field, highlighting the critical need for upskilling in AI tools and technology.
AI's impact on cybersecurity is undeniable, with 88% of professionals expecting significant changes in their roles due to AI in the coming years. Embracing AI tools not only enhances the capabilities of cybersecurity professionals but also paves the way for new job opportunities in the industry.
Automation Threats and Opportunities
In the ever-changing terrain of the cybersecurity job market, professionals must adapt to automation threats and opportunities posed by AI technologies. Cybersecurity professionals believe that AI is impacting their field in substantial ways.
While AI presents opportunities by creating new roles and demands for professionals, it also brings challenges as automation threatens to eliminate routine tasks. As AI continues to advance, it will greatly impact the job market, emphasizing the need for human expertise in ensuring the ethical use of AI in cybersecurity practices.
This shift underscores the evolving role of AI in the industry and highlights the lack of job security for those who do not upskill or specialize.
To navigate these changes successfully, professionals must focus on high-value tasks that require human intervention, emphasizing continuous learning and staying ahead of technological advancements to thrive in the evolving landscape of cybersecurity.
Evolution of AI in Security Roles
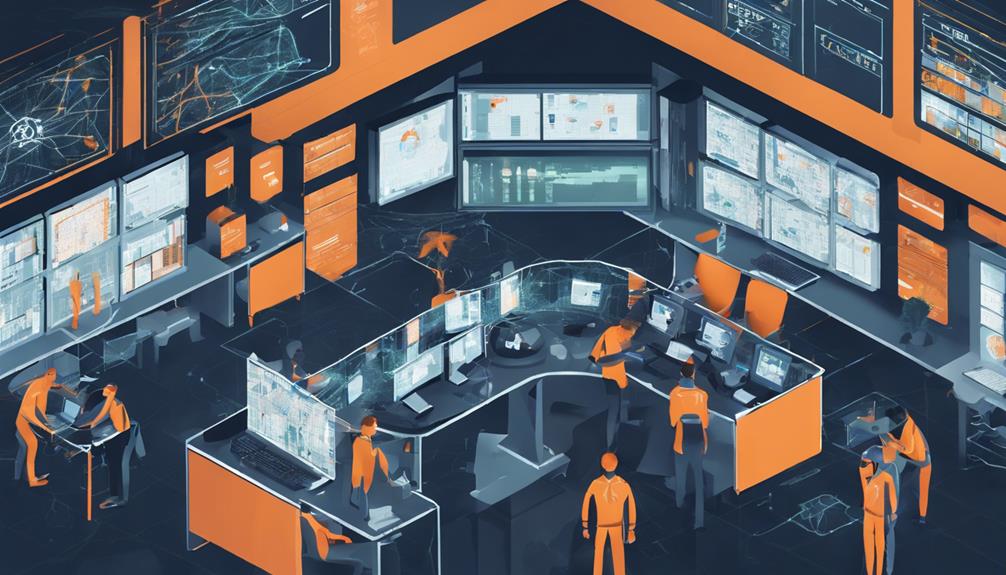
Evolving alongside the rapid advancements in technology, the integration of AI in security roles is reshaping the landscape of cybersecurity professionals' responsibilities. As AI adoption in cybersecurity accelerates, professionals are witnessing significant changes in their daily job functions.
Automation in cybersecurity is becoming more prevalent, with AI handling routine decisions to free up human experts for critical tasks. However, concerns linger about the lack of expertise and awareness within organizations regarding AI integration in security roles.
Cybersecurity jobs are now at a pivotal moment where professionals have the opportunity to lead in implementing secure technology practices through AI. The collaboration between humans and AI is evolving, emphasizing the importance of a symbiotic relationship where each complements the other's strengths.
AI for Good and Bad in Security
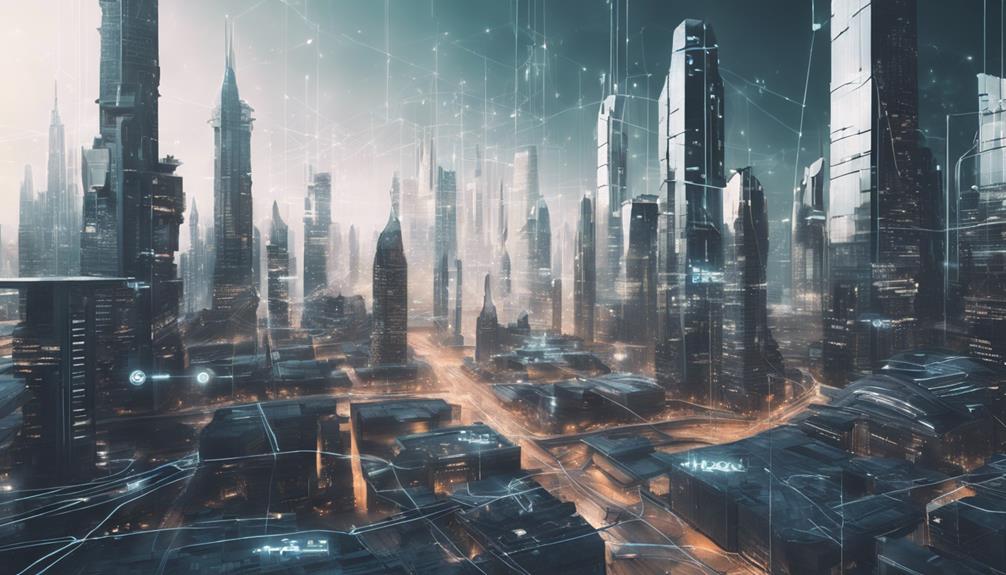
The increasing utilization of AI by malicious actors is dramatically impacting the landscape of cybersecurity, manifesting both beneficial and detrimental implications for security professionals. On one hand, AI tools offer defensive technologies that can analyze vast amounts of data to detect and respond to threats more efficiently.
However, malicious entities are leveraging AI to orchestrate more sophisticated cyber attacks, such as phishing campaigns powered by generative AI to craft convincing messages for social engineering. These advancements enable attackers to produce deep fakes and manipulate voices, escalating the risk of successful infiltrations.
As a result, cybersecurity professionals face the challenge of combating evolving threats that exploit AI capabilities for malicious purposes. Adapting to these changing tactics requires constant vigilance and upskilling to effectively defend against the intricate strategies employed by cybercriminals leveraging AI technology for nefarious activities.
Collaboration Vs. Displacement With AI
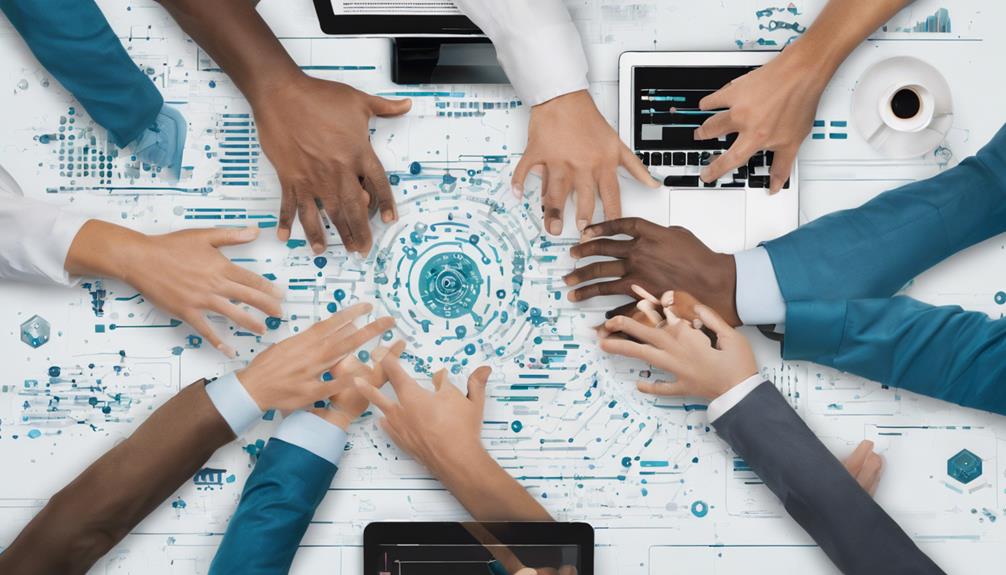
As AI continues to permeate the cybersecurity landscape, professionals are faced with the choice between embracing collaboration or risking displacement in the evolving field. Collaboration among cybersecurity experts is essential to harness the benefits of AI impact on cybersecurity jobs while mitigating the risks of displacement. Organizations must prioritize enhancing expertise and awareness regarding AI integration in cybersecurity to guarantee a smooth shift and secure technology implementation.
To illustrate the importance of collaboration vs. displacement with AI in cybersecurity, consider the following table:
Collaboration Benefits | Displacement Risks |
---|---|
Enhanced threat detection | Job redundancies |
Improved response times | Skill gaps in workforce |
Efficient resource allocation | Resistance to change |
Human Interaction Needs With AI
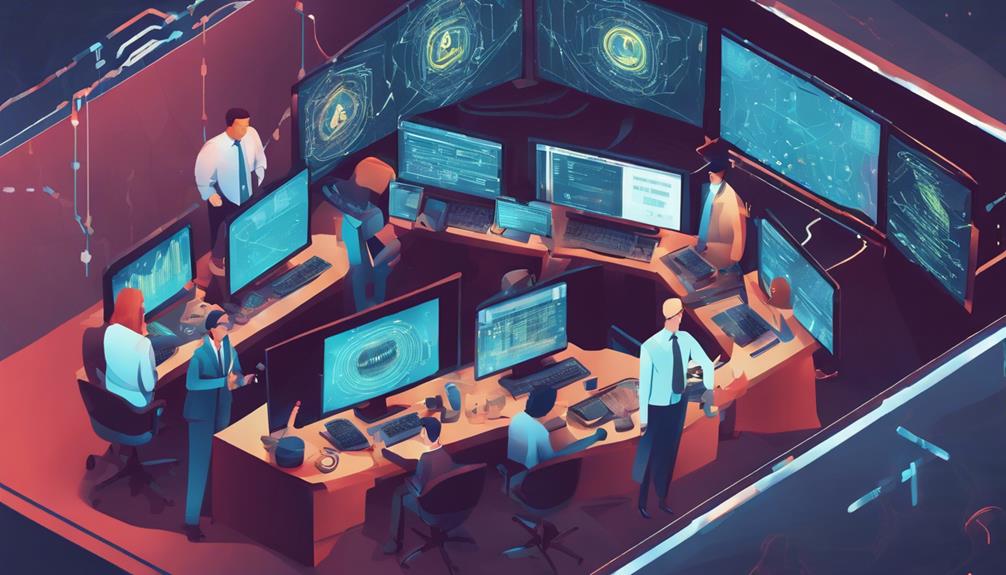
Given the increasing integration of AI in cybersecurity, maintaining effective human interaction with AI systems is essential for cybersecurity professionals to navigate the evolving landscape successfully.
To achieve this, professionals should focus on developing expertise. Continuous learning about AI technologies is vital for cybersecurity professionals to understand their impact on job roles and organizational security.
Enhancing organizational awareness is also crucial. Building a deep understanding within the organization regarding the ethical integration of AI can help in ensuring secure practices and proactive navigation of potential risks.
Moreover, promoting ethical integration is key. Encouraging the ethical use of AI technologies within cybersecurity practices can lead to a more responsible and effective approach, benefiting both professionals and organizations alike.
New Cybersecurity Job Trends
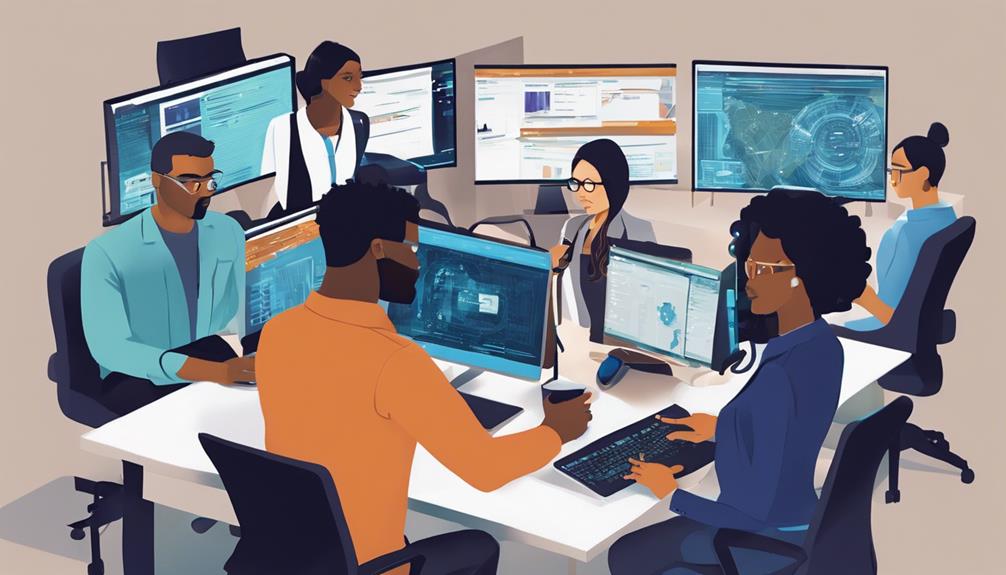
Amidst the evolving landscape of cybersecurity, emerging trends in job opportunities are reshaping the industry's workforce dynamics. With an expected 3.5 million unfilled cybersecurity jobs by 2025, the talent shortage in the industry is glaring.
One significant issue faced is the difficulty in securing entry-level positions without prior experience, exacerbating the skills gap. However, the rise of AI in cybersecurity is seen as a solution to these challenges. AI not only has the potential to fill the skills gap but also to create new job opportunities within the field.
AI adoption in cybersecurity is enhancing the capabilities of professionals, particularly in threat detection, empowering them to combat increasingly sophisticated cyber threats. As organizations integrate AI into their security operations, job growth is anticipated, opening up avenues for individuals to explore new roles and responsibilities in the cybersecurity domain.
This shift towards AI-driven cybersecurity practices is expected to offer a fresh outlook on talent acquisition and skill development, potentially bridging the gap between demand and supply in the industry.
Frequently Asked Questions
How Will AI Affect Cybersecurity Jobs?
AI is poised to revolutionize cybersecurity jobs through automation, threat detection, and response enhancements. As AI technologies evolve, professionals must adapt their skills to leverage these tools effectively, reinforcing the importance of continuous learning and upskilling.
What Is the Main Challenge of Using AI in Cybersecurity?
Amidst the evolving landscape of cybersecurity, the main challenge in utilizing AI lies in the shortage of skilled professionals adept at securing AI technologies. Addressing this expertise gap is imperative for effective AI integration in cybersecurity practices.
How Does AI Contribute to Cyber Security?
AI plays a pivotal role in cybersecurity by enhancing threat detection, incident response, threat intelligence, compliance monitoring, and improving detection quality. It acts as a reliable co-pilot for professionals, integrates with key security platforms, and offers a proactive approach to protection.
Is AI a Risk for Cyber Security?
AI presents both opportunities and risks in cybersecurity. While it enhances defense mechanisms, the misuse of AI by threat actors poses significant risks. Organizations must balance innovation with security measures to mitigate these threats effectively.
Conclusion
To sum up, the impact of AI on cybersecurity jobs is significant and rapidly changing. As AI technology continues to evolve, it offers both advantages and limitations in the field of security.
One interesting statistic to note is that by 2023, it is estimated that there will be a shortage of nearly 3 million cybersecurity professionals worldwide. This highlights the growing need for individuals to adapt to new technologies and skills to meet the demands of the ever-evolving cybersecurity landscape.
Advanced Cybersecurity
AI and Machine Learning in Cybersecurity: A Powerful Combination
Peek into how AI and machine learning revolutionize cybersecurity, enhancing threat detection and incident response with cutting-edge technology.
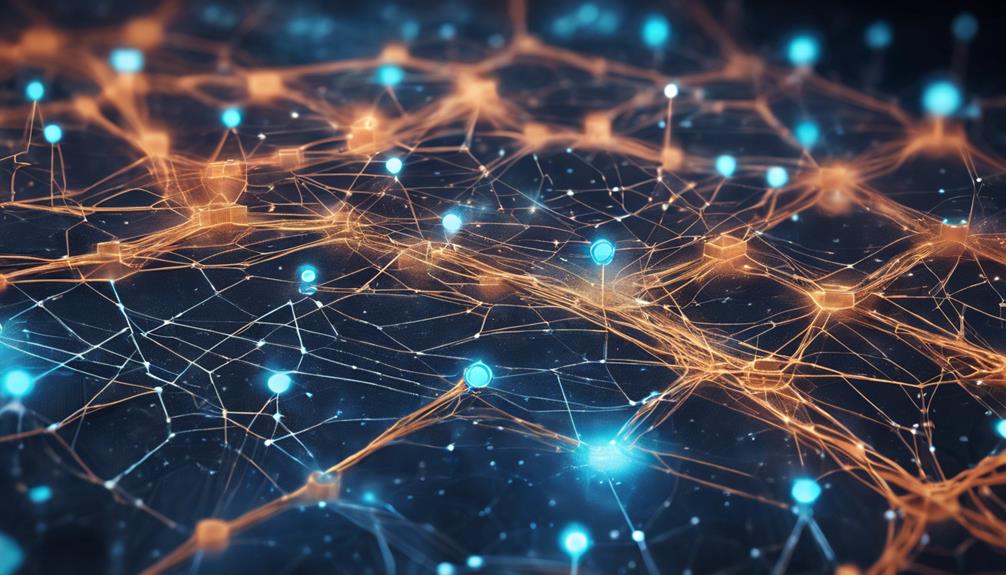
AI and machine learning are transforming cybersecurity, bolstering threat detection, incident response, and security operations. With the evolution from rule-based to deep learning systems, AI now excels in detecting anomalies and patterns. Utilizing historical data, it identifies attack patterns and clusters threats for streamlined response strategies. AI enhances incident response by analyzing data in real-time, automating actions, and providing insightful recommendations. By analyzing vast data sets, it swiftly adapts to dynamic environments, reducing response times. Future trends suggest a deeper integration of AI with security, focusing on user behavior, transparency, and autonomous security operations. The future of cybersecurity is AI-driven.
Key Takeaways
- AI enhances threat detection by analyzing vast data for patterns and anomalies.
- Machine learning identifies known attack patterns for proactive defense.
- Automation of security tasks improves incident response times and efficiency.
- Real-time anomaly detection aids in swift response to evolving threats.
- Integration of AI and ML revolutionizes cybersecurity operations for enhanced protection.
Evolution of AI in Cybersecurity
The evolution of AI in cybersecurity traces back to the mid to late 1980s when early rule-based systems were first utilized for anomaly detection. Since then, the landscape of security measures has been greatly transformed by the integration of machine learning algorithms.
This evolution has been essential in combating advanced cyber threats through enhanced threat detection capabilities. In the late 2000s, supervised learning algorithms played a pivotal role in boosting the accuracy of threat detection systems.
However, it was the advent of deep learning in the 2010s that truly revolutionized cybersecurity. Deep learning enabled the recognition of intricate patterns, providing a more robust defense against evolving threats.
As AI and machine learning technologies continue to progress, they play a crucial role in fortifying security measures across various sectors. The ongoing evolution in anomaly detection, pattern recognition, and overall threat detection capabilities underscores the importance of AI in enhancing cybersecurity protocols.
Machine Learning Techniques for Threat Detection
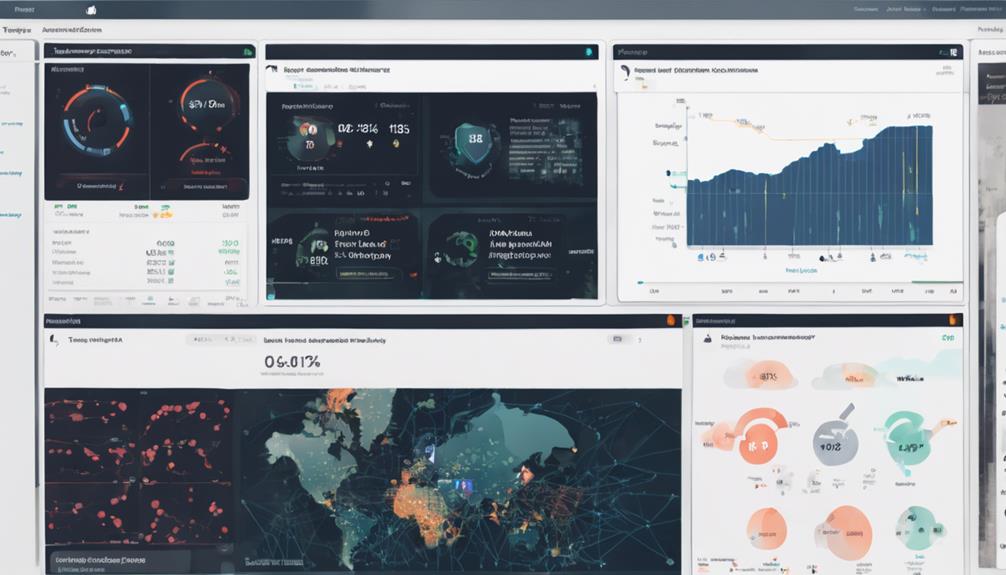
Machine learning techniques employed in cybersecurity play a pivotal role in identifying and mitigating potential threats within network environments. By leveraging AI algorithms to analyze network traffic, cybersecurity systems can proactively detect anomalies and unusual behavior, enhancing threat intelligence for a more secure environment.
These techniques utilize historical data to identify patterns and trends, enabling the detection of known attack patterns and clustering potential threats based on complexity for better risk assessment. Through machine learning, cybersecurity professionals can build a hierarchy of threats, allowing for a more efficient response to cyber threats.
This approach not only aids in threat detection but also helps in improving overall security posture by staying ahead of emerging threats. By embracing machine learning for threat detection, organizations can greatly enhance their cybersecurity defenses and safeguard their digital assets more effectively.
Enhancing Incident Response With AI
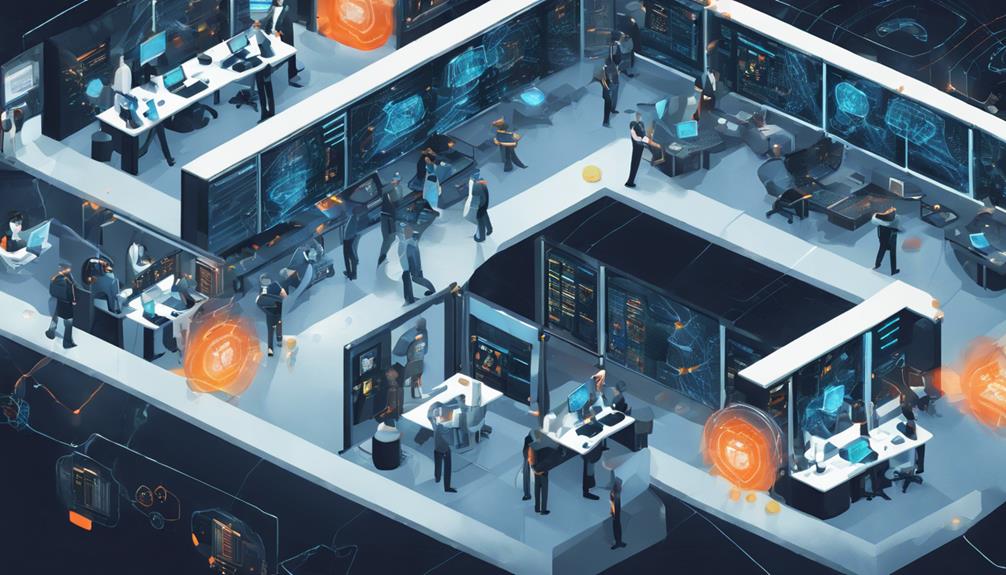
Moreover, utilizing AI technologies in incident response revolutionizes the efficiency and effectiveness of handling security breaches by leveraging advanced data analysis capabilities. Machine learning algorithms embedded in AI-powered systems can detect anomalies in real-time, allowing for swift identification and response to potential threats.
This automation of response actions based on predefined rules streamlines incident resolution processes, ensuring a proactive approach to cybersecurity incidents. Additionally, AI can prioritize alerts and escalate them to the appropriate stakeholders, facilitating efficient incident management by focusing resources where they are most needed.
Moreover, AI enhances decision-making during incident response by providing valuable insights and recommendations drawn from past incidents. By learning from historical data, AI continuously improves incident response strategies, making them more effective and adaptive to evolving cyber threats.
Incorporating AI into incident response not only boosts the speed of resolution but also enhances the overall security posture of organizations.
Leveraging AI in Security Operations
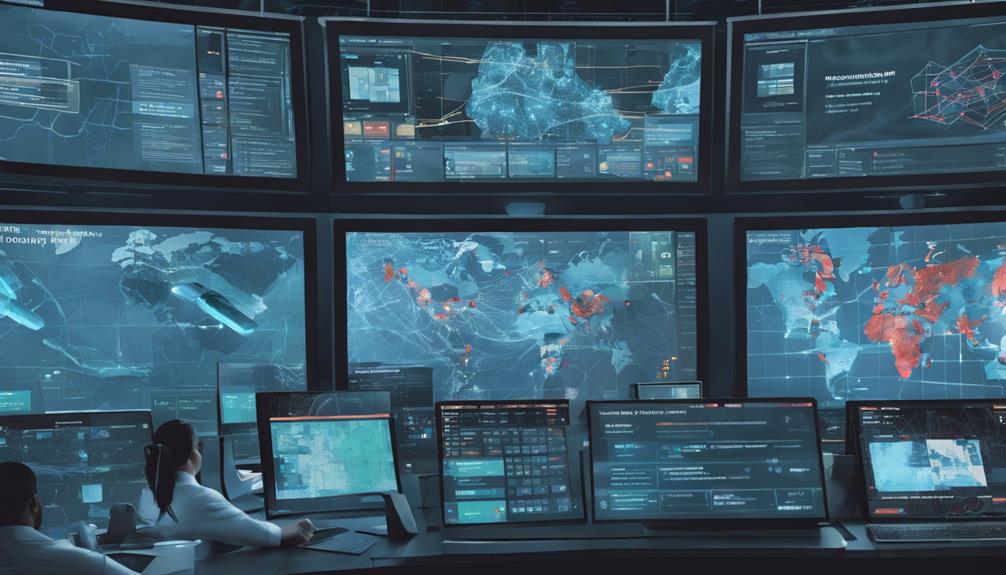
Incorporating artificial intelligence (AI) into security operations revolutionizes threat detection and response capabilities. By leveraging machine learning (ML) algorithms, AI can analyze vast amounts of data to identify patterns of suspicious behavior, enabling proactive threat mitigation. This ability to quickly identify potential threats in networks enhances overall security measures greatly.
Furthermore, AI automates repetitive security tasks, allowing human analysts to focus on more complex threats that require human intervention.
Security operations centers (SOCs) powered by AI can adapt to dynamic environments in real-time, leading to faster incident response times and an improved cybersecurity posture overall.
Future Trends in AI for Cybersecurity
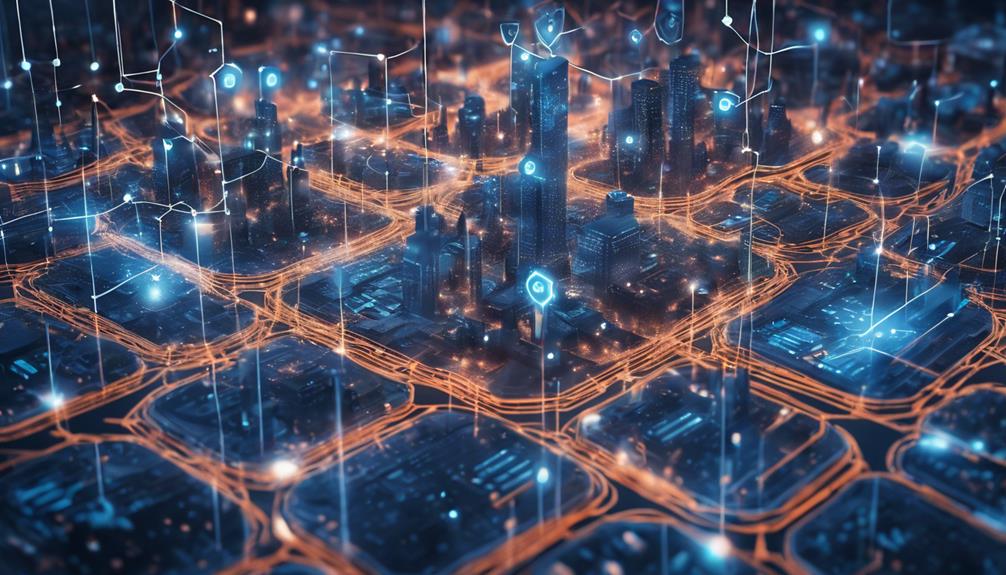
The future trajectory of AI in cybersecurity entails the evolution towards countering advanced cyber threats through innovative technologies and strategies. As the landscape of cybersecurity continues to evolve, here are three key trends shaping the future of AI in cybersecurity:
- Integration with Diverse Security Aspects:
AI and Machine Learning are increasingly being integrated with IoT, cloud security, and endpoint protection to enhance overall cybersecurity defenses and enable proactive threat detection in the face of large volumes of data.
- Significant User Behavior Analysis:
AI plays a significant role in analyzing user behavior patterns to detect anomalies and potential threats, enabling cybersecurity professionals to conduct more effective threat analysis and respond promptly to adversarial attacks.
- Explainable AI and Autonomous Security Operations:
The adoption of Explainable AI is gaining momentum, improving transparency in complex machine learning models. Additionally, AI-driven autonomous security operations centers (ASOCs) are on the rise, automating threat response mechanisms and policy enforcement while facilitating collaboration between AI systems and human experts to develop adaptive cybersecurity strategies.
Frequently Asked Questions
How Is AI and Machine Learning Used in Cyber Security?
AI and machine learning in cybersecurity entail anomaly detection, predictive analytics, phishing detection, automated threat response, and vulnerability management. These technologies enhance threat detection, predict cyber-attacks, and improve overall security by efficiently identifying potential threats and mitigating risks.
Can I Combine AI and Cyber Security?
Yes, the integration of AI in cybersecurity enhances threat detection, response, and mitigation capabilities. Leveraging AI technologies automates tasks, predicts cyber threats, and improves overall security posture. Combining AI with cybersecurity is a recommended approach for robust protection.
Will AI and ML Play a Role in the Future of Cybersecurity?
AI and ML are poised to have a substantial impact on the future of cybersecurity by enhancing defense mechanisms, predicting threats, and improving security postures. These technologies will play a pivotal role in mitigating risks and safeguarding critical infrastructure.
What Is the Intersection of AI and Cybersecurity?
In the intricate dance between artificial intelligence and cybersecurity, AI amplifies threat detection capabilities by unraveling patterns in the digital tapestry, revealing hidden vulnerabilities and fortifying defenses with predictive prowess.
Conclusion
To sum up, the integration of AI and machine learning in cybersecurity is a game-changer, revolutionizing the way organizations detect and respond to threats.
The power of these technologies to analyze vast amounts of data and adapt to evolving threats is unparalleled.
As we move forward, the future of cybersecurity will be shaped by the continued advancements in AI, creating a more secure digital environment for all.
The possibilities are endless, and the impact is immeasurable.
Advanced Cybersecurity
Cybersecurity Using AI: Modern Techniques for Enhanced Protection
Optimize your cybersecurity defenses with cutting-edge AI technology, revolutionizing protection against cyber threats and bolstering your security posture.
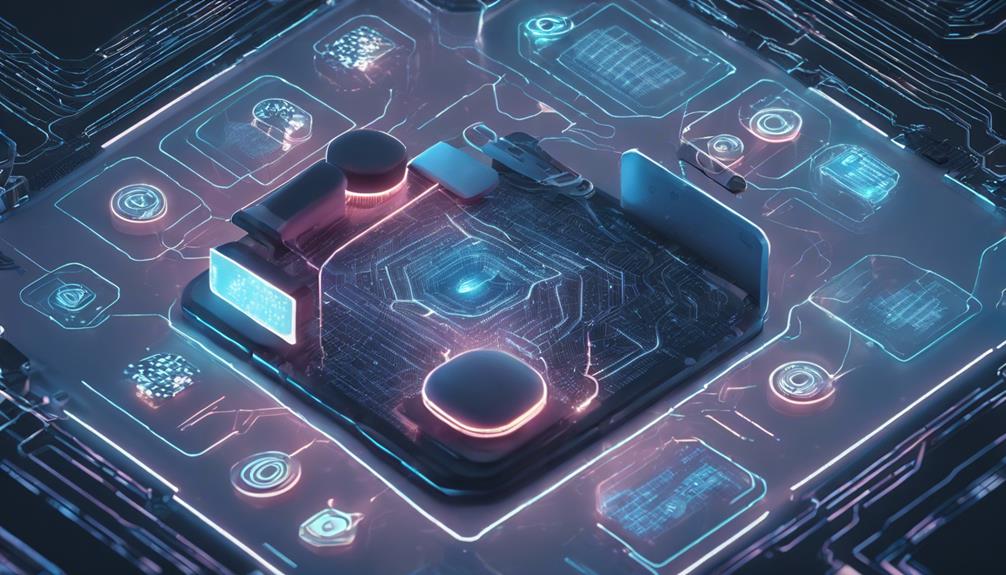
Enhance your cybersecurity with AI, reshaping protection against evolving cyber threats. AI boosts threat detection and response with machine learning technology. Behavioral analysis and anomaly detection heighten security vigilance. Predictive analytics prioritize threat action, automating tasks for efficiency. Proactive defense through AI boosts vulnerability management effectiveness. Swift identification of unknown threats secures systems effectively. Real-time monitoring and adaptive access control fortify cybersecurity posture. Dive deeper into AI's impact on modern protection techniques for thorough cybersecurity enhancement.
Key Takeaways
- AI enhances threat detection with machine learning algorithms.
- Predictive analytics prioritize potential threats for proactive defense.
- Automation streamlines tasks and manages vulnerabilities effectively.
- AI identifies unknown threats swiftly to bolster security measures.
- Integration of AI is crucial in the evolving threat landscape for robust protection.
AI in Cybersecurity Overview
AI in cybersecurity plays a pivotal role in revolutionizing threat detection and incident response through the utilization of machine learning algorithms. By harnessing the power of Artificial Intelligence (AI) and Machine Learning (ML), cybersecurity systems can efficiently detect anomalies and behavioral patterns that signal advanced threats.
Through anomaly detection and behavioral analysis, AI can handle complex security tasks that involve vast amounts of data analysis, enabling proactive defense mechanisms. Predictive analytics further enhances these capabilities by predicting potential threats and prioritizing them for immediate action.
The integration of AI in cybersecurity not only automates tasks but also streamlines incident response, providing real-time threat prevention. By reducing the workload on security teams, AI allows organizations to improve their overall cybersecurity effectiveness.
This modern approach to cybersecurity is essential in combating the ever-evolving landscape of cyber threats, where diverse data sources need to be analyzed thoroughly to ensure robust protection.
Importance of AI in Protection
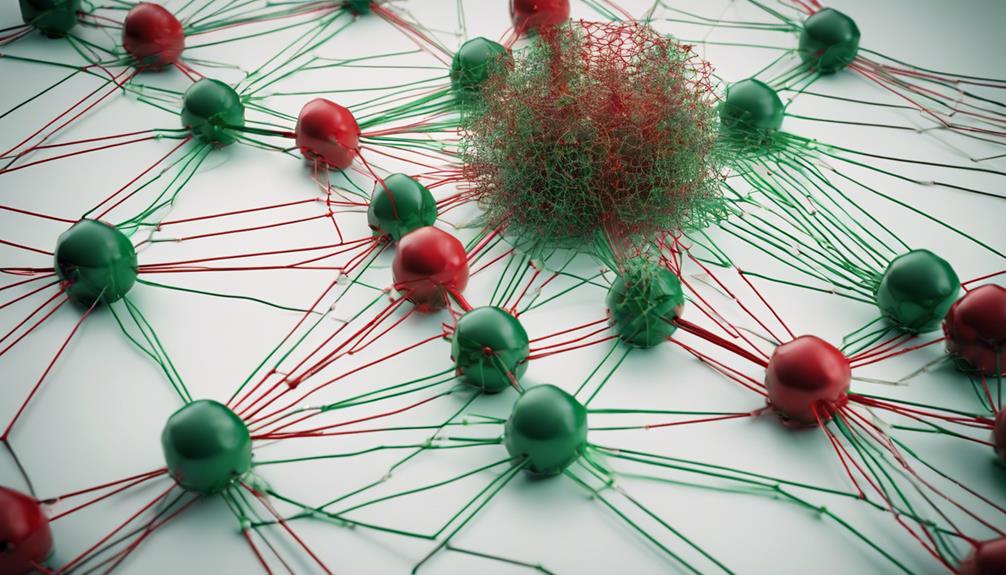
The significance of incorporating Artificial Intelligence technology in cybersecurity for heightened protection measures cannot be overstated. AI plays a pivotal role in cybersecurity by enhancing threat detection capabilities, improving response times, and enabling proactive defense through predictive analytics.
Leveraging AI allows for automating tasks, integrating diverse data sources, and efficiently managing vulnerabilities to prioritize threats effectively. By identifying unknown threats and analyzing large volumes of data swiftly, AI guarantees overall security in the digital landscape.
Implementing AI in cybersecurity not only reduces the workload for security teams but also enhances the ability to address complex cyber threats with precision. In today's ever-evolving threat landscape, where cyberattacks are becoming more sophisticated, the adoption of AI is essential for staying ahead of potential risks and safeguarding sensitive information effectively.
Threat Detection With AI
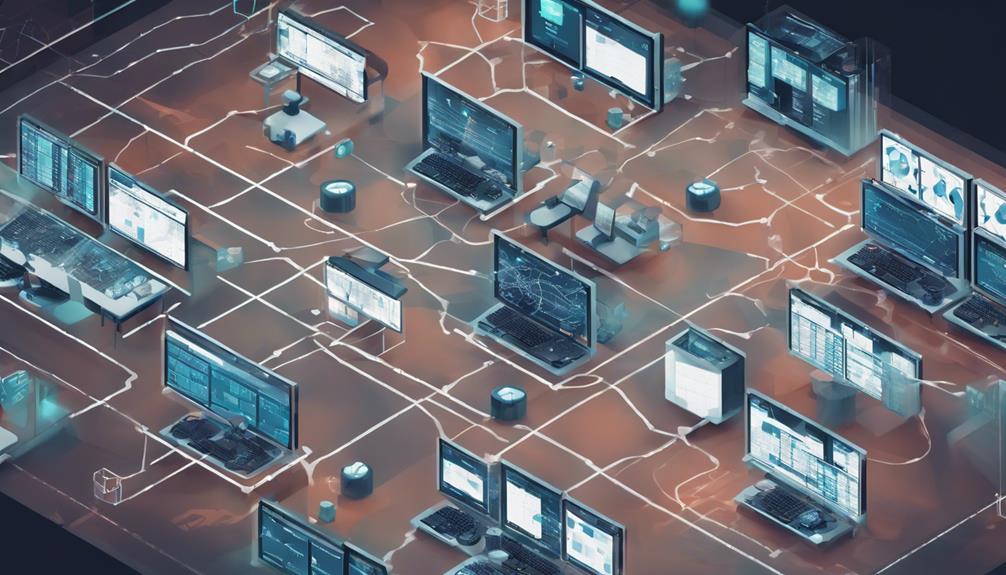
How does artificial intelligence revolutionize threat detection in cybersecurity?
AI plays a pivotal role in enhancing threat detection by leveraging Machine Learning (ML) algorithms to analyze vast amounts of data in real-time. By identifying patterns and anomalies that signify potential threats, AI empowers cybersecurity professionals with automated incident response capabilities, greatly improving response time and accuracy.
Behavioral analysis through AI models enables the proactive identification of suspicious activities, bolstering defense against emerging threats. Additionally, AI solutions continuously monitor and analyze network data, allowing organizations to stay ahead of evolving threats.
AI for Vulnerability Management
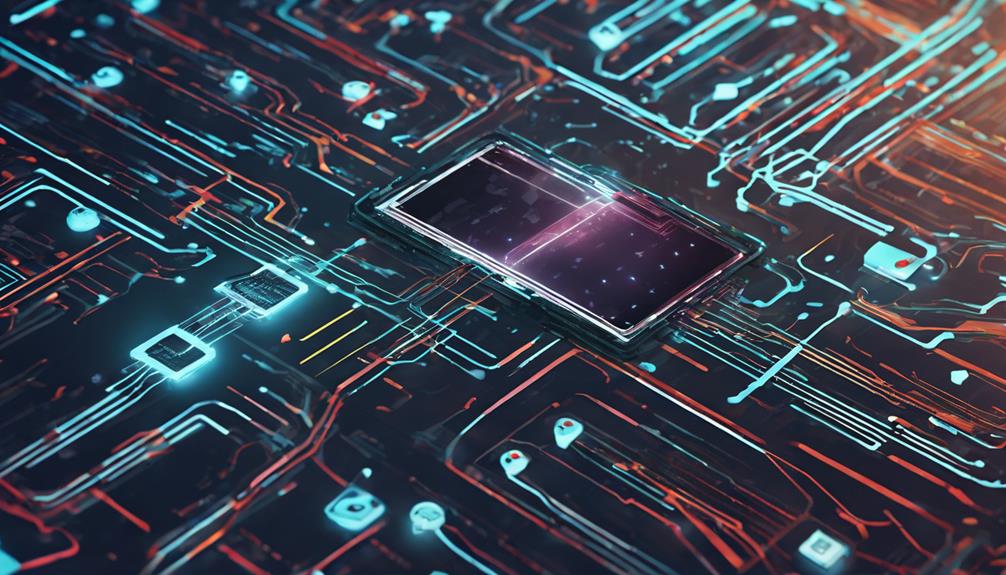
Utilizing advanced algorithms and automation, organizations leverage artificial intelligence to enhance the efficiency of identifying and prioritizing security weaknesses in vulnerability management. Machine learning algorithms play an important role in analyzing vast amounts of data to detect vulnerabilities in systems and networks. AI automates vulnerability scanning and assessment processes, greatly reducing the time required for manual analysis.
This automation not only saves time but also provides real-time insights into potential vulnerabilities, enabling proactive security measures to be implemented promptly. By addressing weaknesses before exploitation, vulnerability management with AI enhances the overall cybersecurity posture of an organization.
In the fast-evolving landscape of cybersecurity threats, utilizing AI for vulnerability management is a proactive approach that can help organizations stay ahead of potential security breaches. By incorporating AI-powered tools into their security strategies, organizations can fortify their defenses and protect against vulnerabilities effectively.
Enhanced Security Measures
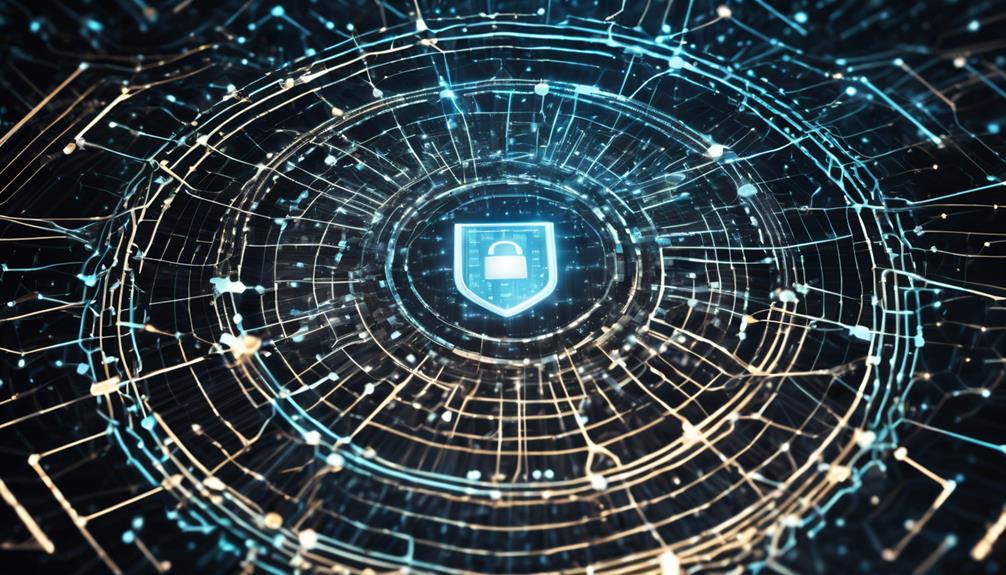
AI's role in cybersecurity extends beyond vulnerability management to encompass enhanced security measures that prioritize critical issues and automate response actions. By leveraging AI, organizations can enhance their defense against emerging threats through proactive threat management.
AI plays an important role in reducing the risk of cyber incidents by prioritizing critical security issues and swiftly fixing them. It automates response actions, enabling quick detection of anomalies and providing actionable intelligence on potential cyber threats.
Additionally, AI's ability to scan systems for risks, suggest security improvements, and support decision-making processes reinforces the least privilege principle, which limits access rights for users to only what are strictly required. This approach noticeably reduces the risk of data breaches and unauthorized access, ultimately enhancing overall cybersecurity posture.
Embracing AI-driven security measures empowers organizations to stay ahead of evolving threats and ensure robust protection against cyberattacks.
Accelerated Response Times
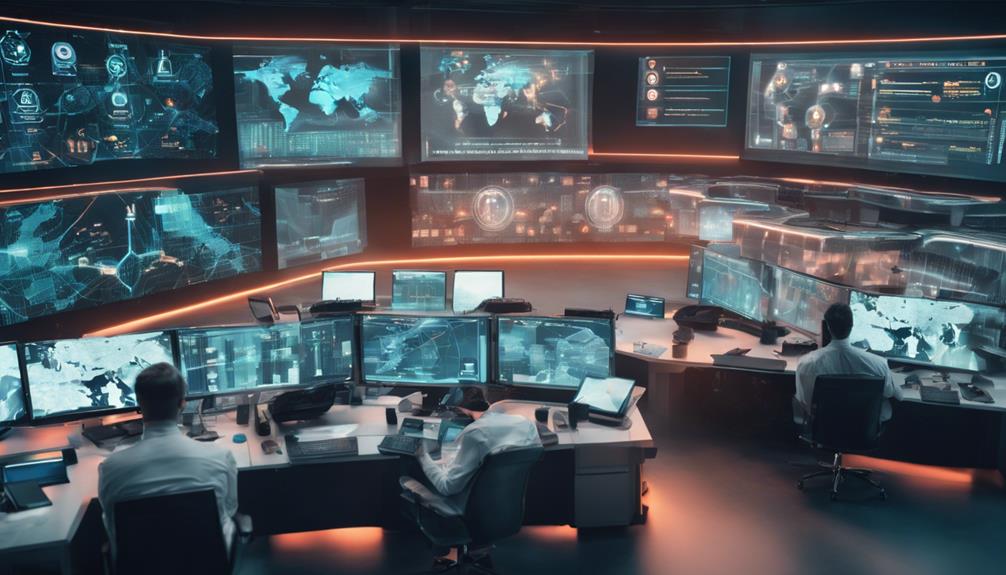
Accelerated response times in cybersecurity using AI offer a significant advantage to organizations.
Real-time threat detection guarantees immediate identification of potential risks, enabling swift action to prevent security breaches.
Automated incident analysis, coupled with instant security alerts, empowers teams to respond effectively, enhancing overall cybersecurity resilience.
Real-Time Threat Detection
Efficiency in identifying and responding to cybersecurity threats is greatly enhanced through the integration of artificial intelligence technologies. AI-powered systems enable real-time threat detection by swiftly analyzing vast amounts of data, leading to immediate threat identification.
This proactive defense mechanism allows for accelerated incident responses, prioritizing critical threats for automated actions. By continuously monitoring networks, endpoints, and applications, AI contributes to overall cybersecurity posture by reducing the time to detect and respond to cyber incidents.
Real-time threat detection with AI not only minimizes potential damages but also empowers organizations to take quick action against emerging threats. The combination of AI's immediate threat detection capabilities and automated responses strengthens the resilience of cybersecurity defenses.
Embracing AI in cybersecurity operations can greatly enhance the security posture of organizations, providing a robust shield against evolving cyber threats.
Automated Incident Analysis
Automated incident analysis plays a pivotal role in enhancing response times in cybersecurity operations. By utilizing AI algorithms, organizations can achieve real-time incident detection and rapid incident analysis, greatly reducing the mean time to detect and respond to cyber threats. This proactive approach enables quicker threat mitigation and enhances overall cybersecurity posture and security effectiveness.
AI-driven automated incident response can efficiently process and prioritize critical threats for immediate action, ensuring that the most pressing issues are addressed promptly. Compared to manual analysis, AI algorithms can process and analyze incidents within seconds, a task that would typically take human analysts hours or even days to complete. This acceleration in response times by up to 65% underscores the importance of automated incident analysis in maintaining a robust cybersecurity defense.
Incorporating automated incident analysis into cybersecurity strategies not only improves response times but also minimizes potential damages by swiftly identifying and addressing threats, ultimately fortifying the organization's security resilience.
Instant Security Alerts
Utilizing AI-powered systems for generating instant security alerts greatly enhances organizations' ability to swiftly respond to potential cyber threats. AI systems can analyze vast amounts of data in real-time, allowing for the immediate detection of suspicious activities that may indicate security incidents. This proactive approach notably reduces response times to cyber threats, sometimes by up to 65%, enabling organizations to take timely action for enhanced protection.
AI-powered tools provide real-time monitoring for swift detection of security incidents.
Instant security alerts offer actionable insights to respond effectively to cyber threats promptly.
Accelerated response times facilitated by AI systems lead to quicker containment and mitigation of potential damages.
Authentication Security With AI
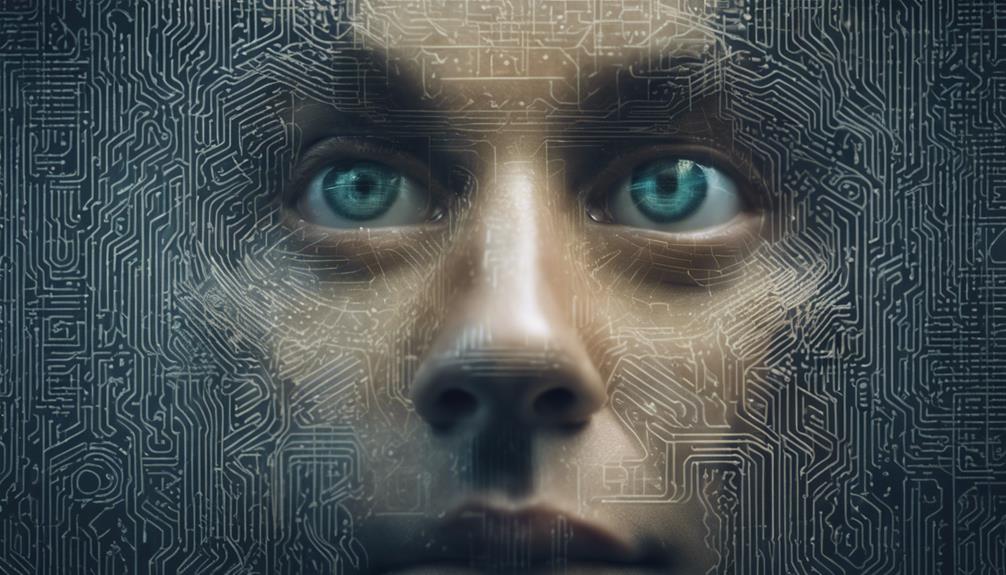
Enhancing authentication security with AI involves utilizing advanced biometric authentication methods. This includes analyzing user behavior for potential threats and implementing adaptive access controls.
By integrating AI-driven solutions, organizations can verify user identities based on unique behavioral traits like typing speed or mouse movements. This helps in bolstering security measures against unauthorized access attempts.
These innovative approaches provide a proactive defense mechanism that adapts to evolving cybersecurity risks. They ensure robust multi-factor authentication protocols for heightened protection.
AI-Driven Biometric Authentication
AI-driven biometric authentication leverages unique biological traits such as fingerprints or facial recognition to provide secure access to systems and data. This innovative approach enhances security measures by reducing the risk of unauthorized access through impersonation.
By utilizing advanced AI algorithms, biometric data is analyzed to accurately and swiftly verify user identities, thereby offering a seamless and efficient authentication process. The integration of AI in biometric authentication guarantees a high level of security, safeguarding against identity theft and fraudulent activities.
Continuous improvements in AI technology further enhance the reliability and precision of biometric authentication systems, reinforcing their efficacy in protecting sensitive information.
- Enhanced Security: Utilizes unique biological traits for secure access.
- Efficient Verification: AI algorithms analyze biometric data to verify identities.
- Protection Against Fraud: Offers a high level of security to prevent identity theft and fraud.
Behavioral Analysis for Security
Behavioral analysis for security, bolstered by artificial intelligence technology, plays a pivotal role in enhancing authentication security measures.
By utilizing AI-driven behavioral analysis, organizations can effectively monitor and detect unusual user behavior, reducing the reliance on traditional authentication methods like passwords. This proactive approach enables the real-time identification of suspicious activities, allowing for immediate response and enhanced security measures.
Through the detection of anomalies in user behavior, AI models can prevent unauthorized access to sensitive information, safeguarding against potential breaches. Additionally, behavioral analysis powered by AI offers a more secure and efficient authentication process, greatly minimizing the risk of fraudulent activities.
Adaptive Access Control
Authentication security in cybersecurity is greatly strengthened by the implementation of adaptive access control, leveraging AI technology to analyze and adjust user behavior in real-time. This advanced approach enhances authentication security by dynamically adapting access permissions based on user behavior, providing a proactive defense against potential security threats.
Key points to note about adaptive access control include:
- AI algorithms assist in identifying unusual user patterns that may indicate security risks.
- Adaptive access control systems continuously monitor user access patterns to detect anomalies and prevent unauthorized access attempts.
- By dynamically adjusting security measures based on real-time user behavior, adaptive access control guarantees a more robust defense against cyber threats.
Through the utilization of AI-driven technologies, organizations can fortify their cybersecurity posture by implementing adaptive access control mechanisms that respond intelligently to evolving user behaviors and potential security challenges.
Automation in Cybersecurity
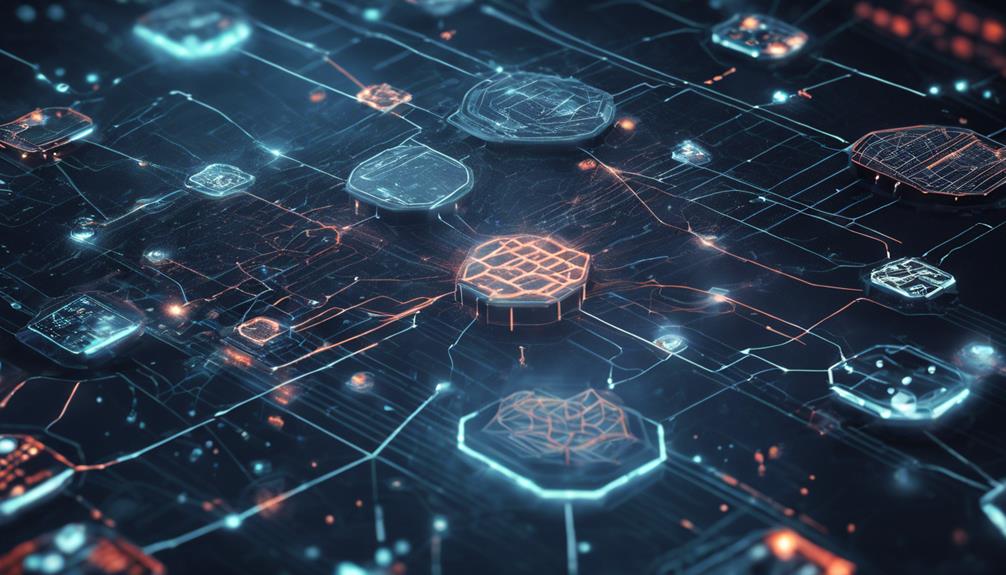
Utilizing automation in cybersecurity processes enhances operational efficiency and response capabilities in addressing cyber threats. AI-driven automation plays an essential role in securing data by enabling real-time monitoring and swift incident response.
With the ability to consolidate and analyze vast amounts of security data efficiently, AI automation streamlines vulnerability assessments and enhances overall security operations' effectiveness. By automating log analysis and vulnerability assessments, security teams can minimize manual tasks, allowing them to focus on more complex cybersecurity challenges.
Additionally, AI automation in cybersecurity not only improves efficiency but also contributes to cost reduction by optimizing the resources required for monitoring and incident response. Embracing AI-driven automation in cybersecurity operations is vital for staying ahead of evolving cyber threats, ensuring robust protection for sensitive data and critical systems.
Frequently Asked Questions
How Does AI Enhance Cybersecurity?
AI enhances cybersecurity by leveraging advanced algorithms to analyze data, detect threats efficiently, automate incident responses, and predict potential risks. It enables proactive defense by staying ahead of evolving threats and streamlining security operations for professionals.
What Is an Example of Cyber Security in Ai?
An example of cybersecurity in AI involves utilizing machine learning algorithms to analyze network data for threat detection. By identifying patterns and anomalies, AI can proactively prevent unknown threats, continuously improving security measures to mitigate cyber risks effectively.
How Can AI Help in Data Protection?
AI aids data protection by analyzing vast data sets to detect anomalies and threats. Machine learning algorithms identify patterns indicating security risks. Real-time monitoring tracks user behavior for unauthorized access. AI automates security tasks, prioritizes alerts, and enhances data protection measures.
Can Cyber Security Be Replaced by Ai?
While AI can bolster cybersecurity defenses, it cannot entirely replace human expertise. Just as a ship needs both its compass and captain, cybersecurity requires the synergy of AI's efficiency with human decision-making for effective threat mitigation and strategic defense.
Conclusion
To sum up, AI technology in cybersecurity provides advanced protection against threats, enhances vulnerability management, and ensures accelerated response times. By implementing AI-driven security measures, organizations can strengthen their defenses and safeguard sensitive data.
It is ironic that the very technology used to create cyber threats can also be leveraged to combat them effectively. Embracing AI in cybersecurity is not just a trend but a necessary step towards securing digital assets in the modern age.
-
Hackathons1 month ago
How to Find Hackathons: Discover Upcoming Hackathon Opportunities Through Community Forums and Listing Platforms.
-
Hackathons1 month ago
Do Hackathons Look Good on Resume: Participating in Hackathons Is an Impressive Accomplishment to Highlight on Resumes.
-
Hackathons1 month ago
Remote Hackathons: Virtual Hackathons Enable Global Teams to Collaborate Remotely.
-
Hackathons1 month ago
MIT Hackathons: the Prestigious MIT Hosts Innovative Student-Led Hackathons in Boston.
-
Hackathons1 month ago
Internal Company Hackathons: Corporations Leverage Internal Hackathons to Crowdsource Ideas and Drive Innovation.
-
Hackathons1 month ago
2024 Hackathons: Get Ready for the Next Wave of Cutting-Edge Hackathon Events in 2024.
-
Hackathons1 month ago
Internal Hackathons: Companies Host Internal Hackathons to Drive Innovation From Within.
-
Hackathons1 month ago
Hackathons in Usa: Don't Miss the Major National and Regional Hackathons Across the United States.