Advanced Cybersecurity
Cybersecurity AI Projects: Innovations and Best Practices
Journey through groundbreaking cybersecurity AI projects, from threat detection to risk analysis, for a glimpse into the future of digital defense.
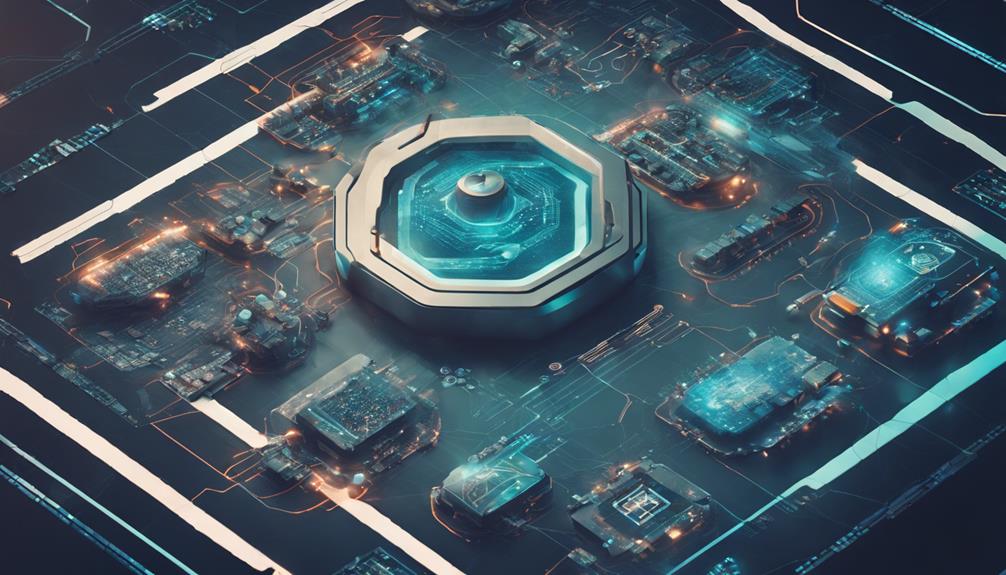
Explore innovative cybersecurity AI projects such as AI-Powered Threat Detection utilizing machine learning for robust defense. Implement Machine Learning for Risk Analysis to predict and preempt cyber risks effectively. AI-driven Incident Response Systems streamline threat management with automated responses. Enhance Security Operations Centers with AI tools for real-time analysis and proactive threat mitigation. Adaptive Authentication Solutions guarantee secure user verification and anomaly detection. Embrace cutting-edge Behavioral Analytics for anomaly detection and incident response efficiency. Secure Data Encryption Techniques fortify data protection against cyber threats. Discover more on regulatory compliance automation and advanced cybersecurity practices for a fortified digital defense.
Key Takeaways
- AI-powered threat detection enhances security defenses.
- Machine learning predicts and proactively addresses risks.
- Advanced security techniques include anomaly detection and encryption.
- Automated incident response systems minimize breach impact.
- AI strengthens security operations with real-time analysis.
AI-Powered Threat Detection
AI-Powered Threat Detection plays an essential role in modern cybersecurity defense strategies by utilizing advanced machine learning algorithms to analyze data and identify potential security risks.
These AI-powered systems can analyze vast amounts of data in real-time, using machine learning models to detect anomalies and patterns indicative of cyber threats.
By automating threat detection, organizations can greatly enhance the speed and accuracy of identifying and responding to security incidents.
One key advantage of AI-powered threat detection is the ability of AI models to continuously learn and adapt to evolving cyber threats.
This adaptability improves the overall effectiveness of cybersecurity defenses by staying ahead of sophisticated attacks.
Implementing AI-powered threat detection tools can help organizations protect sensitive data and maintain a proactive stance against cyber threats.
Machine Learning for Risk Analysis
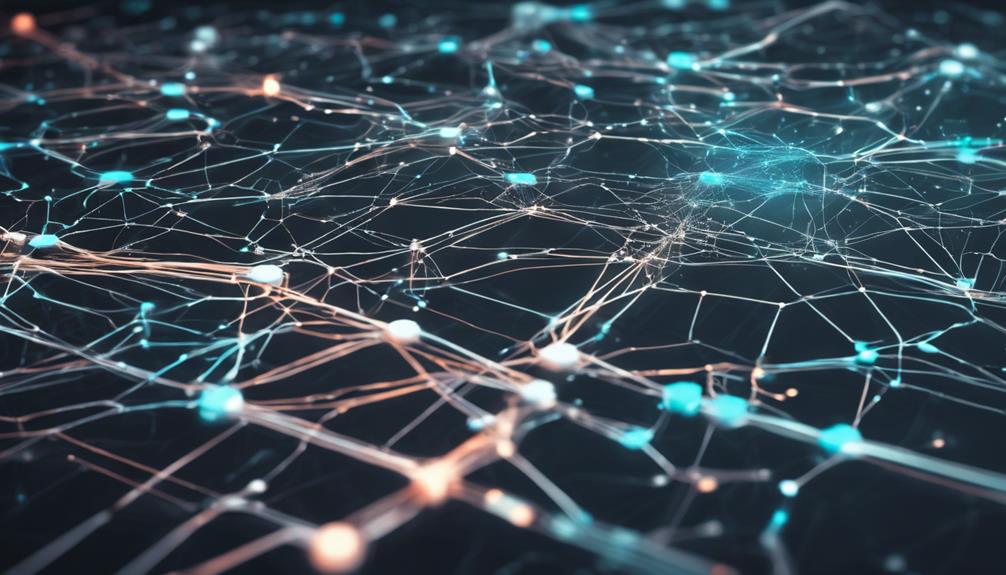
Machine learning plays a vital role in analyzing cybersecurity risks by utilizing advanced algorithms to detect anomalies in network traffic, system behavior, and user activity. These algorithms not only identify potential threats but also predict cybersecurity risks, helping organizations to proactively strengthen their defenses.
Risk Detection Algorithms
Utilizing advanced risk detection algorithms powered by machine learning, organizations can proactively identify and thwart potential cyber threats. These algorithms leverage the capabilities of machine learning to analyze patterns and anomalies in data, enhancing threat detection accuracy.
Here are key points on the significance of risk detection algorithms in bolstering cybersecurity posture:
- Critical Threat Identification: Risk detection algorithms excel in spotting potential cyber threats like malware, phishing attacks, and unauthorized access attempts swiftly and accurately.
- Continuous Learning: Machine learning models used in risk analysis continuously learn from new data, improving threat detection capabilities and adapting to the dynamic cybersecurity landscape.
- Proactive Risk Mitigation: By integrating AI-driven risk detection algorithms, organizations can preemptively identify and address security risks before they escalate into significant incidents, ultimately fortifying their cybersecurity posture and response efficiency.
Incorporating machine learning for risk analysis ensures automated, real-time monitoring of security events, which is essential for maintaining a robust cybersecurity posture in today's digital landscape.
Threat Prediction Models
Risk prediction models leverage machine learning algorithms to analyze historical data and patterns for anticipating cybersecurity threats. These AI models play a vital role in enhancing security measures by identifying potential risks and vulnerabilities in real-time. By continuously learning from new data, these models can adapt to evolving cyber threats, providing accurate risk assessments to prevent security breaches effectively.
Here is a table summarizing key aspects of threat prediction models:
Key Aspect | Description | Importance |
---|---|---|
Real-time Detection | Identifying threats as they occur enables proactive responses. | High |
Anomaly Detection | Detecting unusual activities that may indicate a cyber attack. | Critical |
Adaptability | Ability to learn from new data and evolve to counter emerging threats. | Essential |
Accuracy | Providing precise risk assessments to prevent security breaches effectively. | Vital |
Ethical Guidelines | Ensuring the use of AI models aligns with ethical standards in cybersecurity. | Fundamental |
Considering the importance of these aspects and adherence to ethical guidelines, implementing threat prediction models can significantly enhance an organization's cybersecurity posture.
Anomaly Detection Techniques
Anomaly detection techniques in cybersecurity AI projects leverage advanced machine learning algorithms to identify irregular patterns or behaviors within data. These techniques play a pivotal role in risk analysis by detecting potential security breaches, fraudulent activities, or abnormal occurrences within a system.
Key points regarding anomaly detection techniques in cybersecurity AI projects include:
- Machine learning algorithms like neural networks, support vector machines, and clustering methods are commonly employed to detect anomalies effectively.
- By analyzing historical data and establishing normal behavior patterns, AI systems can swiftly identify deviations that may signal security threats.
- The implementation of anomaly detection techniques fortifies cybersecurity defenses by facilitating proactive monitoring and real-time threat detection, thereby enhancing overall system security.
Through the integration of these sophisticated machine learning algorithms, cybersecurity AI projects can enhance their ability to detect and mitigate potential risks, ensuring a more secure digital environment.
Automated Incident Response Systems
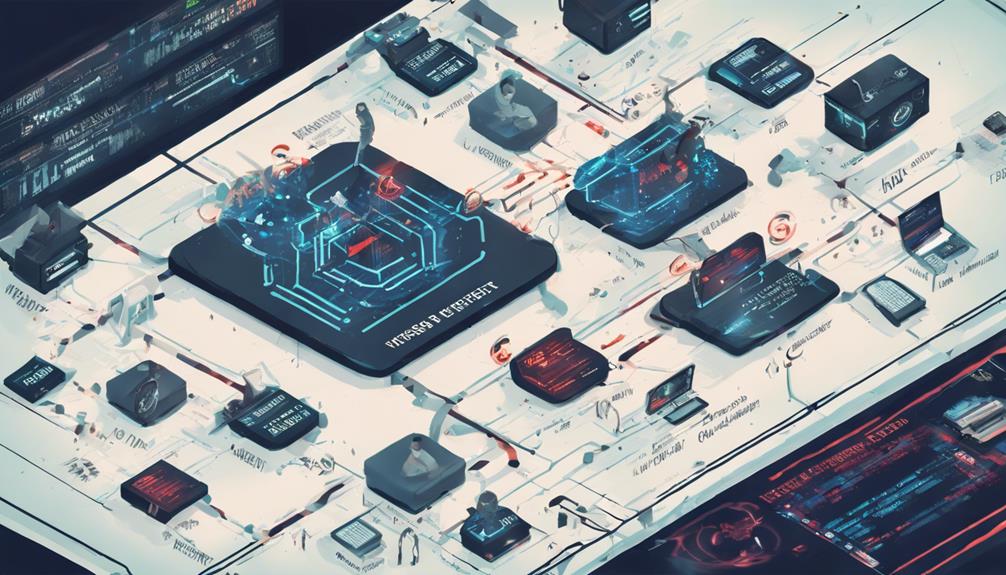
Automated Incident Response Systems leverage AI technology to swiftly identify and neutralize cybersecurity threats as they arise.
By automating the detection and response process, organizations can enhance their security posture and minimize the impact of potential breaches.
Through the use of AI-driven systems, companies can achieve greater efficiency in threat management, allowing for proactive measures to be taken promptly.
AI in Incident Response
Utilizing advanced AI algorithms, Incident Response systems leverage real-time detection and response capabilities in cybersecurity operations. AI in cybersecurity has revolutionized incident response by introducing automated systems that enhance security measures.
Here are key aspects highlighting the significance of AI in Incident Response:
- Efficient Threat Detection: Automated incident response systems can swiftly analyze vast amounts of data, enabling the identification of potential threats and vulnerabilities in real-time. This proactive approach helps in mitigating risks before they escalate.
- Streamlined Incident Handling: By automating repetitive tasks such as data analysis and initial response actions, AI systems enable security analysts to focus on complex threats and strategic decision-making. This streamlining of processes reduces response times and minimizes the impact of cybersecurity incidents.
- Enhanced Cybersecurity Posture: The integration of AI in Incident Response not only improves response efficiency but also strengthens overall cybersecurity posture by facilitating proactive threat detection and response strategies. This proactive stance is essential in combating evolving cyber threats effectively.
Threat Detection Automation
AI-powered systems for automated incident response play a pivotal role in enhancing cybersecurity defenses by rapidly detecting and responding to potential threats in real-time. These AI systems employ sophisticated algorithms to sift through vast amounts of data, pinpointing patterns and anomalies that could signify security breaches.
By automating incident response, organizations can greatly reduce response times, swiftly mitigating the impact of cyber attacks. Continuous monitoring for security threats using AI-driven tools further bolsters the overall cybersecurity posture of a system.
Implementing Automated Incident Response Systems not only enhances security but also improves efficiency and accuracy in responding to security incidents. These systems act as proactive guardians, tirelessly scanning and analyzing for any signs of security threats, providing a robust layer of defense in the digital domain.
In today's dynamic threat landscape, leveraging AI for automated incident response is a strategic move towards fortifying cybersecurity defenses.
Efficiency Through AI
Enhancing operational efficiency through the integration of advanced incident response systems driven by artificial intelligence technology is paramount in modern cybersecurity strategies. AI applications in incident response offer a range of benefits, including:
- Reduced Response Time: Automated incident response systems powered by AI can decrease the time to address cyber threats by up to 65%, enabling organizations to react swiftly to potential breaches.
- Real-Time Analysis: These AI-driven systems have the capability to analyze large volumes of security data in real-time, facilitating quick detection and response to cyber attacks before they escalate.
- Enhanced Security Posture: By automating routine tasks and prioritizing alerts, AI incident response tools help organizations allocate resources efficiently, strengthening their overall security posture and data protection measures.
Implementing AI technologies in incident response not only streamlines processes but also empowers cybersecurity professionals to address more complex security issues, ultimately fortifying an organization's resilience against evolving threats.
Enhancing Security Operations Centers
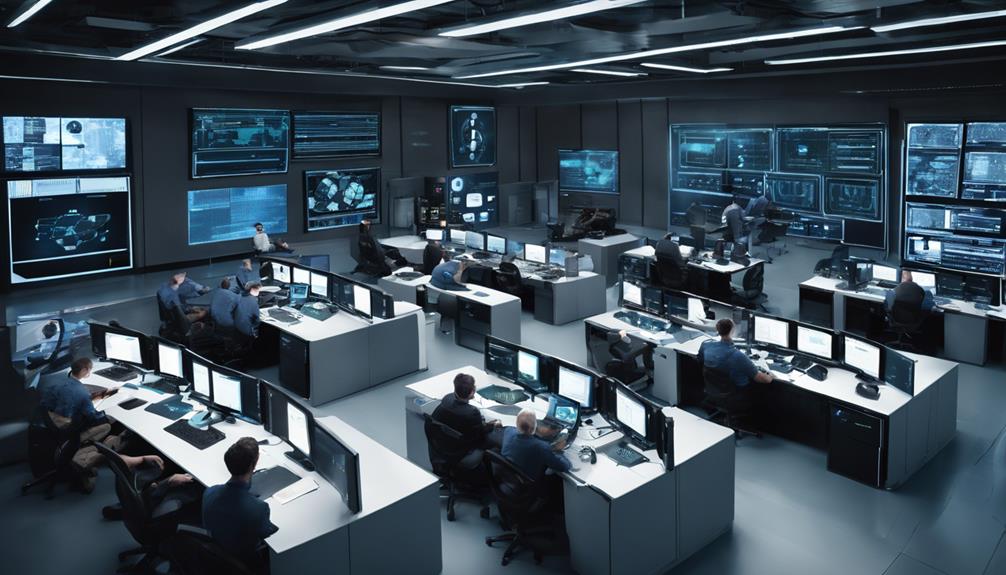
Security Operations Centers leverage advanced artificial intelligence technologies to bolster their capabilities in detecting and responding to cyber threats. By integrating AI tools, SOCs can enhance their cyber defense mechanisms through real-time analysis of vast amounts of data. Machine learning algorithms play an essential role in improving detection accuracy and reducing response times to potential security breaches. These AI-powered SOCs enable proactive threat hunting and mitigation strategies, thereby fortifying the organization's cybersecurity posture.
The incorporation of artificial intelligence in SOCs not only enhances threat detection but also elevates overall security operations efficiency and effectiveness. With AI-driven systems in place, security teams can swiftly identify anomalies, predict potential threats, and take preemptive actions to safeguard the organization's digital assets. This blend of human expertise with machine intelligence equips SOCs with the necessary tools to combat evolving cyber threats effectively, ensuring a robust defense mechanism against sophisticated adversaries.
Adaptive Authentication Solutions
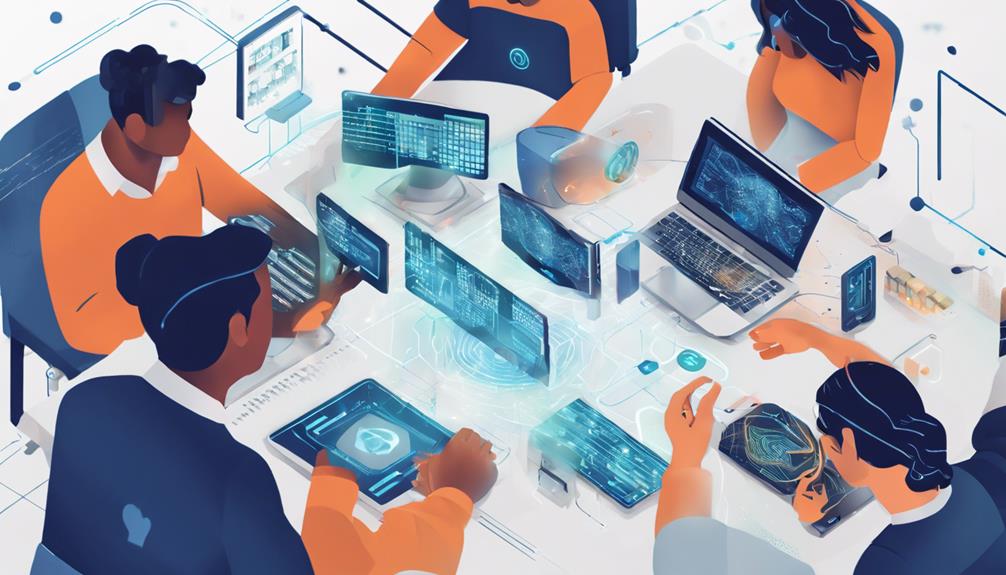
In the field of cybersecurity technology, adaptive authentication solutions revolutionize user verification processes by leveraging AI algorithms to dynamically adjust security measures based on behavioral patterns and risk assessments. These AI-driven adaptive authentication systems offer a proactive approach to cybersecurity defenses, enhancing user security through real-time analysis of user behavior.
Here are key points highlighting the importance of adaptive authentication solutions:
- Essential Security Measures: By analyzing patterns, locations, devices, and other user behavior factors, adaptive authentication solutions verify user identity more accurately, reducing the risk of unauthorized access.
- Anomaly Detection: AI-driven adaptive authentication can detect anomalies and potential threats in real-time, providing organizations with advanced warning to prevent security breaches.
- Improved User Experience: Organizations benefit from reduced false positives and improved user experience with adaptive authentication technologies, ensuring a seamless and secure authentication process.
These continuous monitoring and learning capabilities strengthen cybersecurity defenses against evolving threats, making adaptive authentication solutions an essential component of a robust cybersecurity strategy.
Behavioral Analytics for Anomaly Detection
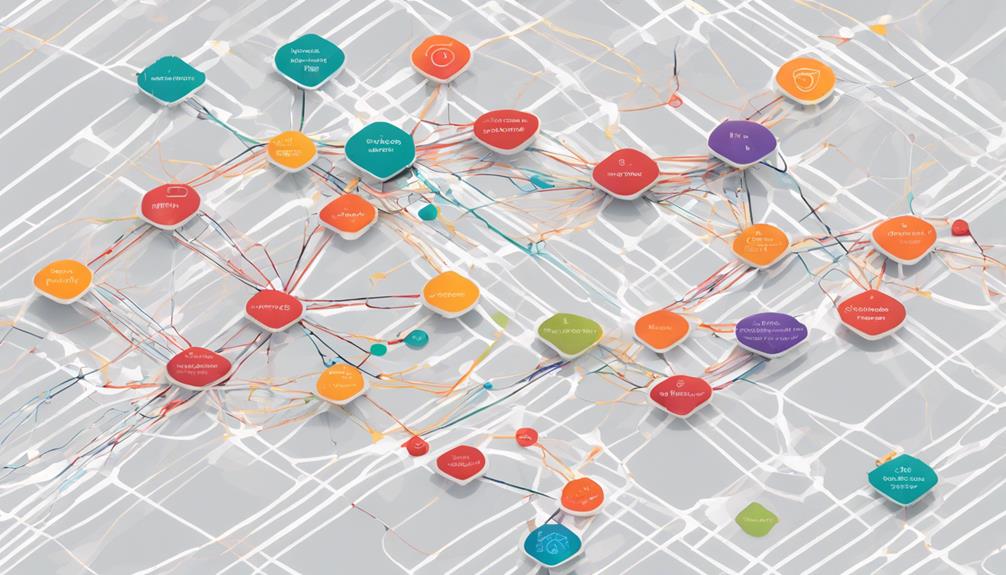
Utilizing advanced artificial intelligence algorithms, behavioral analytics for anomaly detection plays an essential role in fortifying cybersecurity defenses by identifying deviations from typical user behavior patterns. By leveraging AI algorithms, this approach can effectively detect anomalous activities that may signify insider threats, advanced persistent threats, or other malicious behaviors.
The continuous learning capability of AI algorithms in behavioral analytics allows for improved detection accuracy over time, adapting to evolving cybersecurity threats.
Monitoring user activities in real-time enables organizations to proactively respond to potential security breaches by swiftly identifying behavior-based indicators of compromise. This proactive stance minimizes false positives and enhances incident response efficiency, focusing on the most critical threats.
Secure Data Encryption Techniques
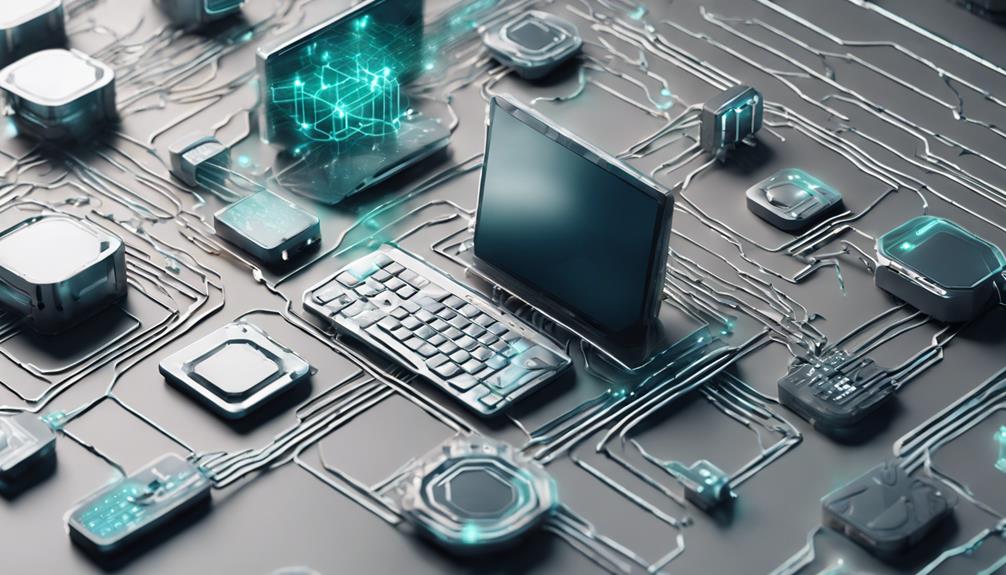
Secure data encryption techniques play a pivotal role in safeguarding sensitive information within AI projects. When implementing encryption strategies, consider the following key aspects:
- Encryption Keys: Encryption keys are fundamental in securing data by allowing only authorized parties to decrypt it. Managing and protecting these keys is essential to maintaining the integrity of the encryption process.
- Advanced Encryption Standards: Utilizing advanced encryption standards such as SHA-256 and AES-256 enhances the security of AI systems against unauthorized access. These robust encryption algorithms provide a high level of protection for sensitive data.
- End-to-End Encryption: Implementing end-to-end encryption methodologies ensures that data remains secure during transmission and storage within AI systems. This thorough approach to encryption minimizes the risk of interception or tampering, safeguarding critical information effectively.
Regulatory Compliance Automation
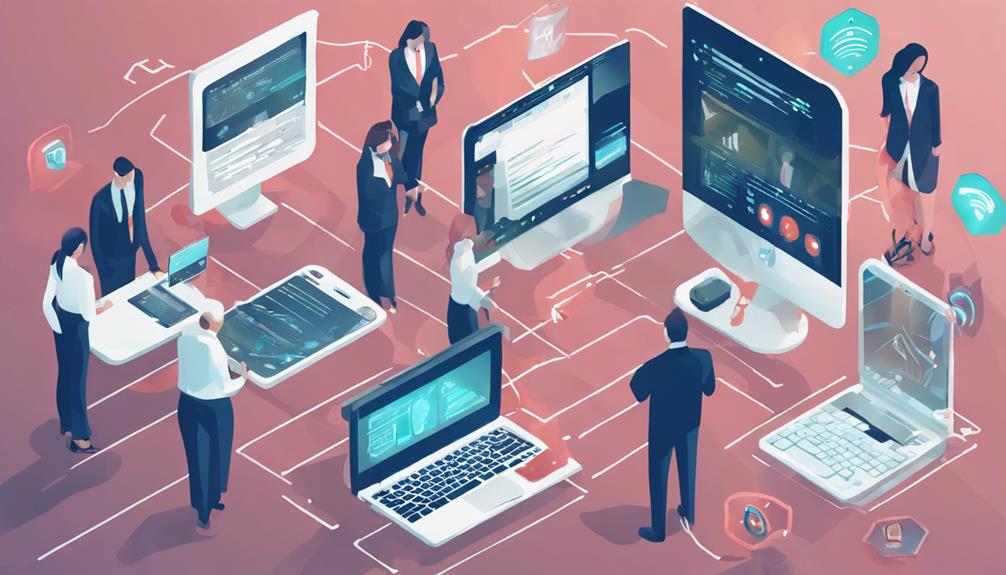
Data encryption techniques are essential for safeguarding sensitive information within AI projects, and regulatory compliance automation further enhances security measures by utilizing AI algorithms to guarantee adherence to industry regulations like GDPR.
In cybersecurity AI projects, regulatory compliance automation plays a pivotal role in ensuring that organizations meet the necessary standards set by regulatory bodies. By automating compliance checks through AI algorithms, potential violations can be identified and addressed with increased efficiency and accuracy. This not only helps in reducing human error but also streamlines the auditing process for companies, leading to enhanced overall security posture.
Automated compliance tools continuously monitor AI systems to ensure they adhere to industry regulations, thereby minimizing legal risks and maintaining data protection. Implementing regulatory compliance automation is essential for organizations looking to stay compliant with evolving industry regulations while leveraging the capabilities of AI technology to enhance cybersecurity measures.
Frequently Asked Questions
What Are Some Examples of AI in Cyber Security?
In cybersecurity, AI is utilized for anomaly detection in network traffic, predictive analysis of security breaches, autonomous threat response with intrusion detection systems, identification of insider threats through behavioral analytics, and proactive threat monitoring with AI-driven intelligence platforms.
How Can AI Be Implemented in Cyber Security?
In the field of cybersecurity, AI serves as a vigilant sentinel, fortifying defenses through threat detection, anomaly analysis, and automated response mechanisms. By leveraging machine learning algorithms and deep learning models, AI bolsters security operations with enhanced efficiency and accuracy.
How Is Cybersecurity AI Being Improved?
Cybersecurity AI is enhanced through algorithm and model updates to address vulnerabilities, adherence to data protection regulations like GDPR, robust access controls, authentication mechanisms, security audits, adversarial testing, and transparency in AI models to identify biases and vulnerabilities.
How Are AI Systems Typically Used in the Cyber Security Industry?
AI systems are instrumental in cyber security for threat detection, anomaly identification, and automation of tasks like log analysis. By leveraging machine learning and deep learning algorithms, these systems can enhance security monitoring, detect malicious activities, and predict vulnerabilities to fortify defense mechanisms.
Conclusion
In summary, the implementation of cybersecurity AI projects is essential in today's digital landscape.
By leveraging AI-powered threat detection, machine learning for risk analysis, and automated incident response systems, organizations can enhance their security operations and protect sensitive data.
Behavioral analytics, secure encryption techniques, and regulatory compliance automation further strengthen defenses against cyber threats.
Embracing these innovations and best practices will guarantee a proactive approach to cybersecurity, safeguarding against potential breaches and maintaining data integrity.
Remember, in the world of cybersecurity, prevention is always better than cure.
Advanced Cybersecurity
AI Impact on Cybersecurity Jobs: What to Expect
Yearning for insights on how AI is reshaping cybersecurity roles? Discover the evolving landscape and skills needed to stay ahead.
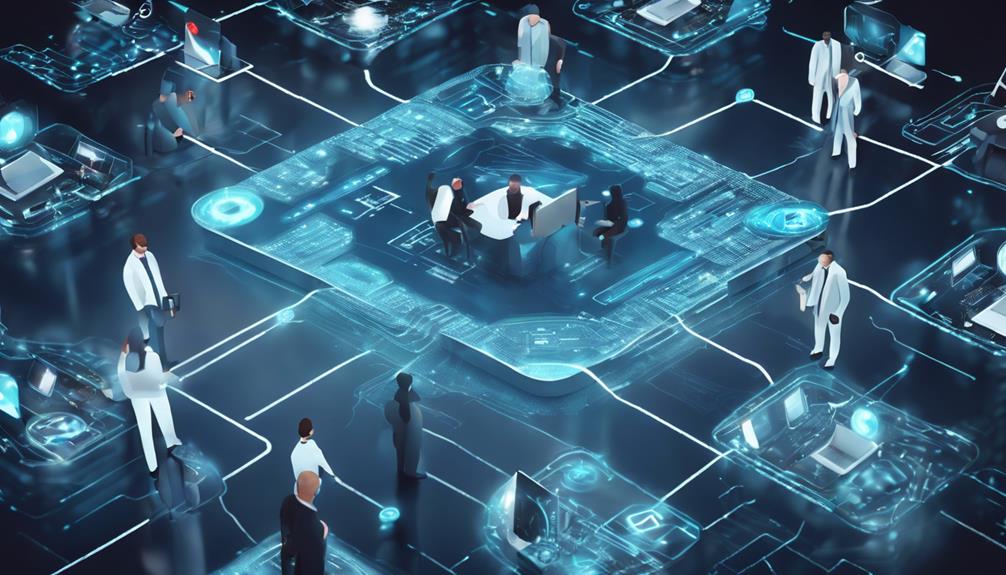
As AI transforms cybersecurity, expect job roles to evolve, requiring updated skills and responsibilities. AI boosts threat detection and job efficiency, automates tasks, and fills skill gaps. However, AI lacks human judgment, emphasizing the need for human validation. Professionals must upskill in AI to adapt to these changes, as demand for AI skills in cybersecurity rises. AI both creates new job opportunities and threatens automation of routine tasks. Continuous learning and ethical AI integration are emphasized for professionals to thrive. The evolving landscape of cybersecurity jobs necessitates a proactive approach to stay relevant in this dynamic field.
Key Takeaways
- AI adoption will reshape cybersecurity roles.
- Demand for AI skills in cybersecurity will rise.
- Job market will see new opportunities and demands.
- Routine tasks may face automation threats.
- Continuous learning and upskilling are crucial for success.
AI's Advantages in Cybersecurity
In the field of cybersecurity, artificial intelligence (AI) offers a multitude of advantages, revolutionizing threat detection and serving as a reliable copilot for professionals. AI in cybersecurity has a significant impact on job efficiency, with 82% of experts foreseeing enhancements in their tasks.
By automating routine processes and analyzing vast amounts of data at incredible speeds, AI allows cybersecurity professionals to focus on more strategic and complex issues. However, there are concerns about the potential misuse of AI in cyberattacks, particularly through the creation of deepfakes and social engineering tactics.
To address these challenges, organizations must establish formal policies on the safe and ethical use of AI. Currently, only 27% of organizations have such policies in place, highlighting the urgent need for enhanced governance and regulation in this area.
Current Limitations of AI in Security
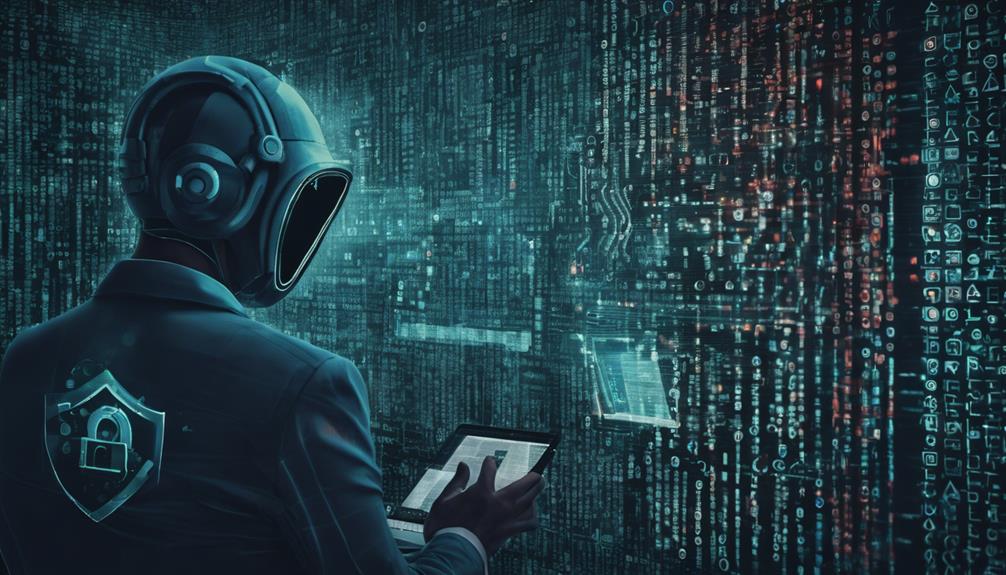
Despite the advancements in AI technology enhancing cybersecurity tasks, current limitations exist that impede its effectiveness in security measures. AI in security lacks human judgment and intuition, necessitating high-quality data for training to prevent bias.
Human validation, enhancement, and domain-specific training are essential to bolster AI's security capabilities. Professionals harbor concerns about the security and privacy of data fed into AI systems, underlining the significance of data quality and human input for continuous validation and enhancement.
To mitigate these limitations, AI systems in security must undergo careful monitoring and training to deliver accurate and unbiased results. Addressing these challenges will require a concerted effort to bridge the gap between AI capabilities and human expertise, ensuring that AI technology in cybersecurity can reach its full potential in safeguarding digital assets and systems against evolving threats.
Impact on Cybersecurity Job Market
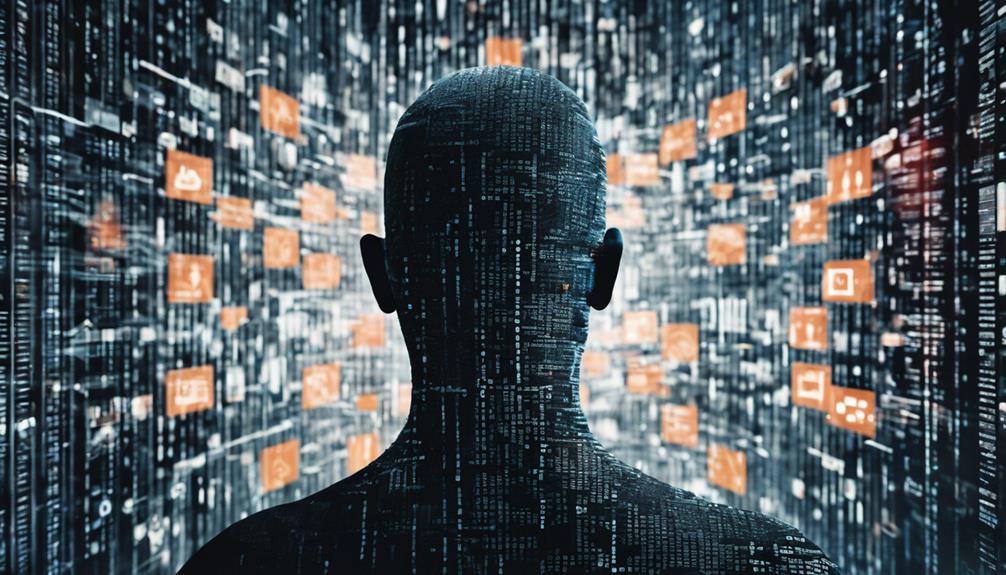
The impact of AI on the cybersecurity job market is multifaceted. Job demand is shifting, requiring professionals to evolve their skills to meet new challenges.
While automation poses threats, it also presents opportunities for cyber experts to leverage AI in enhancing their capabilities for better threat detection and response.
Job Demand Shifts
With the rapid advancements in AI technology, the landscape of cybersecurity job demand is undergoing significant shifts. The integration of AI in cybersecurity is not only enhancing the current capabilities of cyber security professionals in threat detection but also opening up new job opportunities.
As the demand for cyber security professionals continues to rise, AI is helping to fill the skills gap that has led to an expected 3.5 million unfilled cyber security jobs by 2025. AI's impact is particularly beneficial in increasing efficiency and effectiveness in handling cyber threats, making it a valuable tool for both entry-level positions and experienced professionals.
This shift in job demand towards AI-integrated roles highlights the importance of acquiring skills in AI technologies to stay competitive in the evolving cyber security job market. Embracing AI in cybersecurity jobs is not just a trend but a necessity for meeting the growing demands of the industry.
Skills Evolution Needed
As the demand for cybersecurity professionals continues to surge, the necessity for acquiring advanced skills in AI technologies becomes increasingly apparent in order to address the talent shortage and evolving landscape of cyber threats.
The cybersecurity job market is facing a significant skills gap, with an anticipated 3.5 million unfilled positions by 2025. Without prior expertise, professionals find it challenging to enter the field, highlighting the critical need for upskilling in AI tools and technology.
AI's impact on cybersecurity is undeniable, with 88% of professionals expecting significant changes in their roles due to AI in the coming years. Embracing AI tools not only enhances the capabilities of cybersecurity professionals but also paves the way for new job opportunities in the industry.
Automation Threats and Opportunities
In the ever-changing terrain of the cybersecurity job market, professionals must adapt to automation threats and opportunities posed by AI technologies. Cybersecurity professionals believe that AI is impacting their field in substantial ways.
While AI presents opportunities by creating new roles and demands for professionals, it also brings challenges as automation threatens to eliminate routine tasks. As AI continues to advance, it will greatly impact the job market, emphasizing the need for human expertise in ensuring the ethical use of AI in cybersecurity practices.
This shift underscores the evolving role of AI in the industry and highlights the lack of job security for those who do not upskill or specialize.
To navigate these changes successfully, professionals must focus on high-value tasks that require human intervention, emphasizing continuous learning and staying ahead of technological advancements to thrive in the evolving landscape of cybersecurity.
Evolution of AI in Security Roles
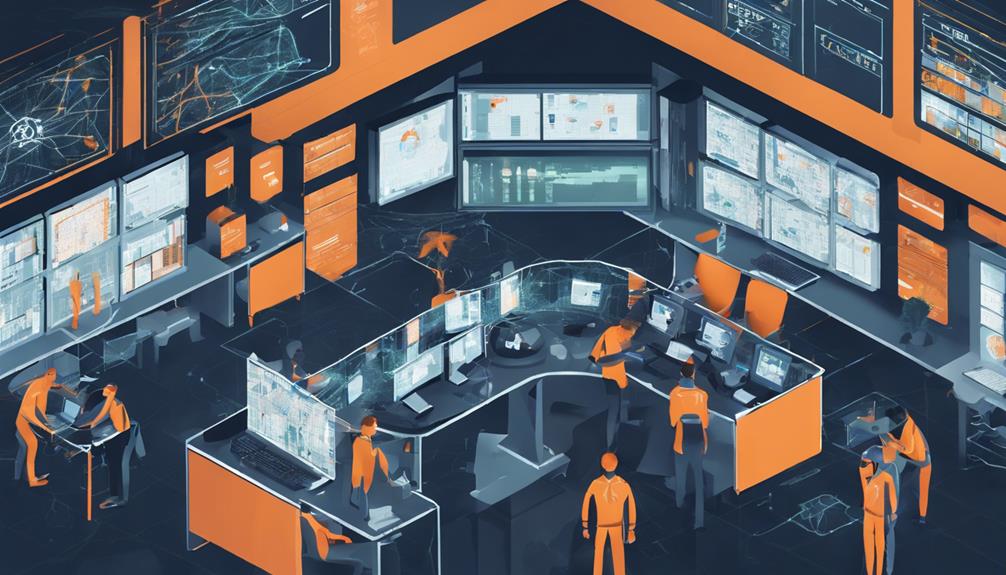
Evolving alongside the rapid advancements in technology, the integration of AI in security roles is reshaping the landscape of cybersecurity professionals' responsibilities. As AI adoption in cybersecurity accelerates, professionals are witnessing significant changes in their daily job functions.
Automation in cybersecurity is becoming more prevalent, with AI handling routine decisions to free up human experts for critical tasks. However, concerns linger about the lack of expertise and awareness within organizations regarding AI integration in security roles.
Cybersecurity jobs are now at a pivotal moment where professionals have the opportunity to lead in implementing secure technology practices through AI. The collaboration between humans and AI is evolving, emphasizing the importance of a symbiotic relationship where each complements the other's strengths.
AI for Good and Bad in Security
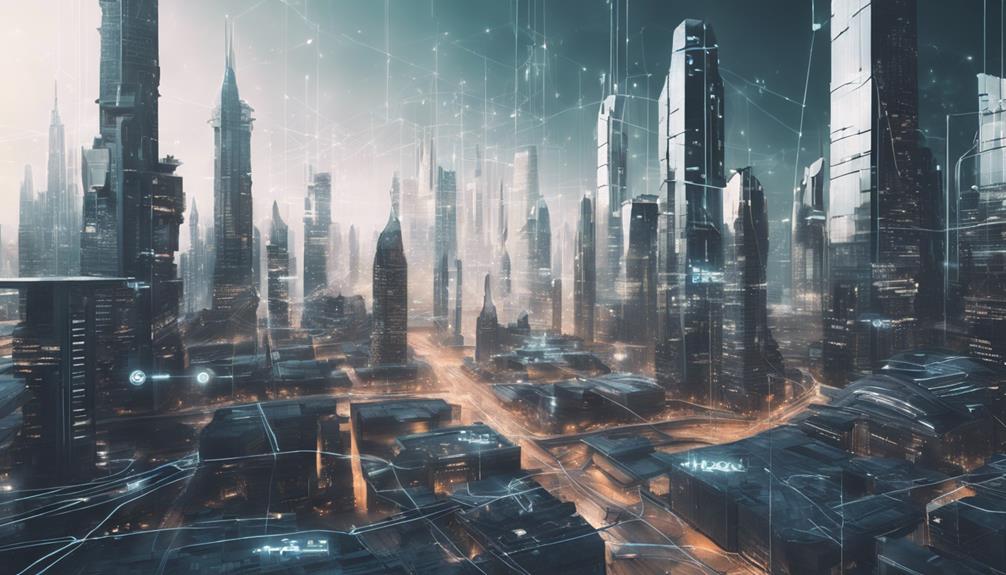
The increasing utilization of AI by malicious actors is dramatically impacting the landscape of cybersecurity, manifesting both beneficial and detrimental implications for security professionals. On one hand, AI tools offer defensive technologies that can analyze vast amounts of data to detect and respond to threats more efficiently.
However, malicious entities are leveraging AI to orchestrate more sophisticated cyber attacks, such as phishing campaigns powered by generative AI to craft convincing messages for social engineering. These advancements enable attackers to produce deep fakes and manipulate voices, escalating the risk of successful infiltrations.
As a result, cybersecurity professionals face the challenge of combating evolving threats that exploit AI capabilities for malicious purposes. Adapting to these changing tactics requires constant vigilance and upskilling to effectively defend against the intricate strategies employed by cybercriminals leveraging AI technology for nefarious activities.
Collaboration Vs. Displacement With AI
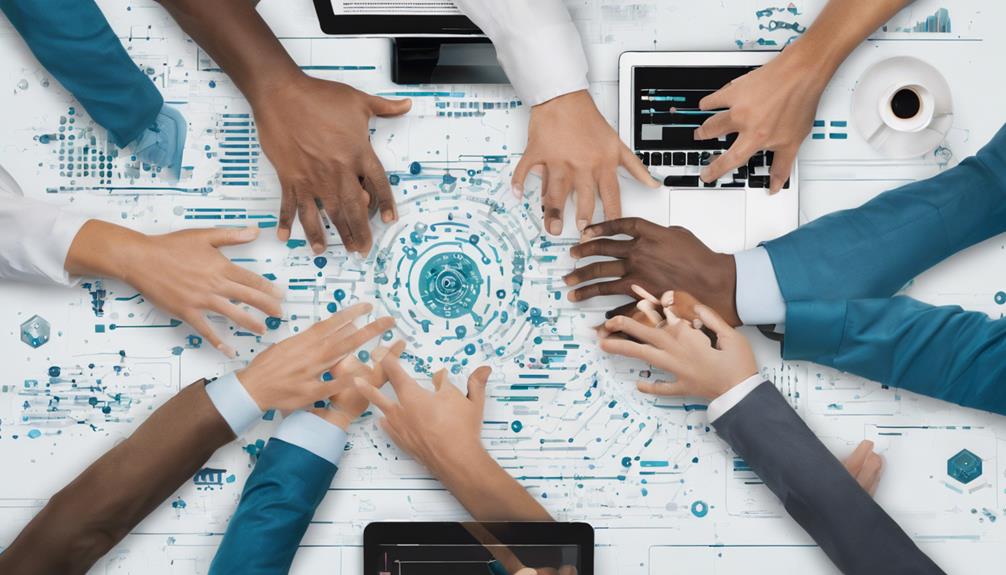
As AI continues to permeate the cybersecurity landscape, professionals are faced with the choice between embracing collaboration or risking displacement in the evolving field. Collaboration among cybersecurity experts is essential to harness the benefits of AI impact on cybersecurity jobs while mitigating the risks of displacement. Organizations must prioritize enhancing expertise and awareness regarding AI integration in cybersecurity to guarantee a smooth shift and secure technology implementation.
To illustrate the importance of collaboration vs. displacement with AI in cybersecurity, consider the following table:
Collaboration Benefits | Displacement Risks |
---|---|
Enhanced threat detection | Job redundancies |
Improved response times | Skill gaps in workforce |
Efficient resource allocation | Resistance to change |
Human Interaction Needs With AI
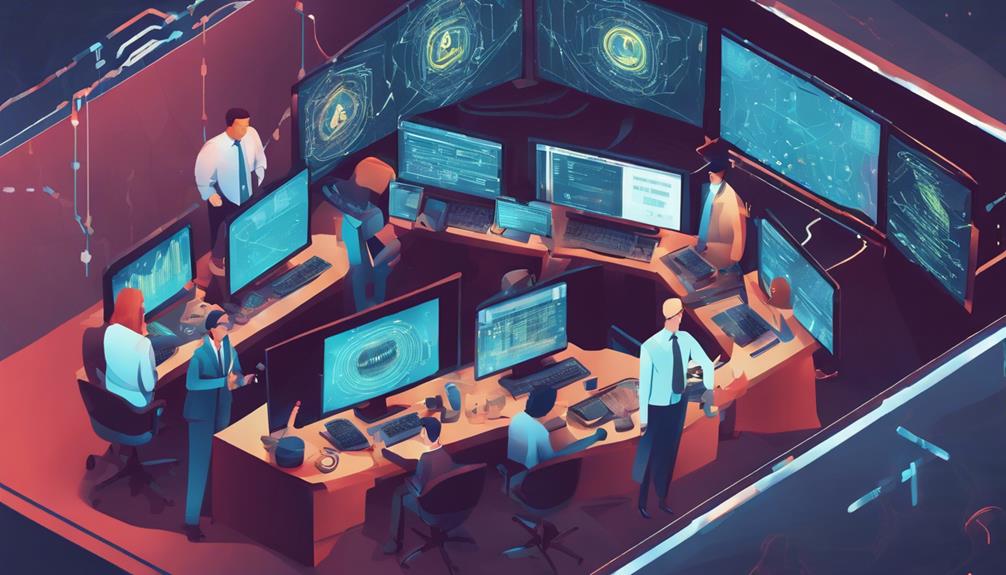
Given the increasing integration of AI in cybersecurity, maintaining effective human interaction with AI systems is essential for cybersecurity professionals to navigate the evolving landscape successfully.
To achieve this, professionals should focus on developing expertise. Continuous learning about AI technologies is vital for cybersecurity professionals to understand their impact on job roles and organizational security.
Enhancing organizational awareness is also crucial. Building a deep understanding within the organization regarding the ethical integration of AI can help in ensuring secure practices and proactive navigation of potential risks.
Moreover, promoting ethical integration is key. Encouraging the ethical use of AI technologies within cybersecurity practices can lead to a more responsible and effective approach, benefiting both professionals and organizations alike.
New Cybersecurity Job Trends
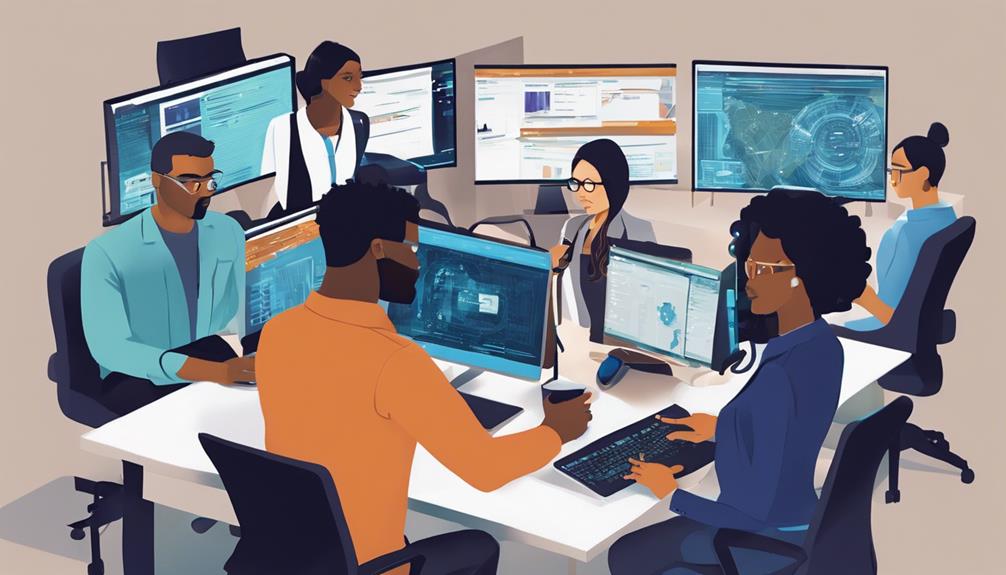
Amidst the evolving landscape of cybersecurity, emerging trends in job opportunities are reshaping the industry's workforce dynamics. With an expected 3.5 million unfilled cybersecurity jobs by 2025, the talent shortage in the industry is glaring.
One significant issue faced is the difficulty in securing entry-level positions without prior experience, exacerbating the skills gap. However, the rise of AI in cybersecurity is seen as a solution to these challenges. AI not only has the potential to fill the skills gap but also to create new job opportunities within the field.
AI adoption in cybersecurity is enhancing the capabilities of professionals, particularly in threat detection, empowering them to combat increasingly sophisticated cyber threats. As organizations integrate AI into their security operations, job growth is anticipated, opening up avenues for individuals to explore new roles and responsibilities in the cybersecurity domain.
This shift towards AI-driven cybersecurity practices is expected to offer a fresh outlook on talent acquisition and skill development, potentially bridging the gap between demand and supply in the industry.
Frequently Asked Questions
How Will AI Affect Cybersecurity Jobs?
AI is poised to revolutionize cybersecurity jobs through automation, threat detection, and response enhancements. As AI technologies evolve, professionals must adapt their skills to leverage these tools effectively, reinforcing the importance of continuous learning and upskilling.
What Is the Main Challenge of Using AI in Cybersecurity?
Amidst the evolving landscape of cybersecurity, the main challenge in utilizing AI lies in the shortage of skilled professionals adept at securing AI technologies. Addressing this expertise gap is imperative for effective AI integration in cybersecurity practices.
How Does AI Contribute to Cyber Security?
AI plays a pivotal role in cybersecurity by enhancing threat detection, incident response, threat intelligence, compliance monitoring, and improving detection quality. It acts as a reliable co-pilot for professionals, integrates with key security platforms, and offers a proactive approach to protection.
Is AI a Risk for Cyber Security?
AI presents both opportunities and risks in cybersecurity. While it enhances defense mechanisms, the misuse of AI by threat actors poses significant risks. Organizations must balance innovation with security measures to mitigate these threats effectively.
Conclusion
To sum up, the impact of AI on cybersecurity jobs is significant and rapidly changing. As AI technology continues to evolve, it offers both advantages and limitations in the field of security.
One interesting statistic to note is that by 2023, it is estimated that there will be a shortage of nearly 3 million cybersecurity professionals worldwide. This highlights the growing need for individuals to adapt to new technologies and skills to meet the demands of the ever-evolving cybersecurity landscape.
Advanced Cybersecurity
AI and Machine Learning in Cybersecurity: A Powerful Combination
Peek into how AI and machine learning revolutionize cybersecurity, enhancing threat detection and incident response with cutting-edge technology.
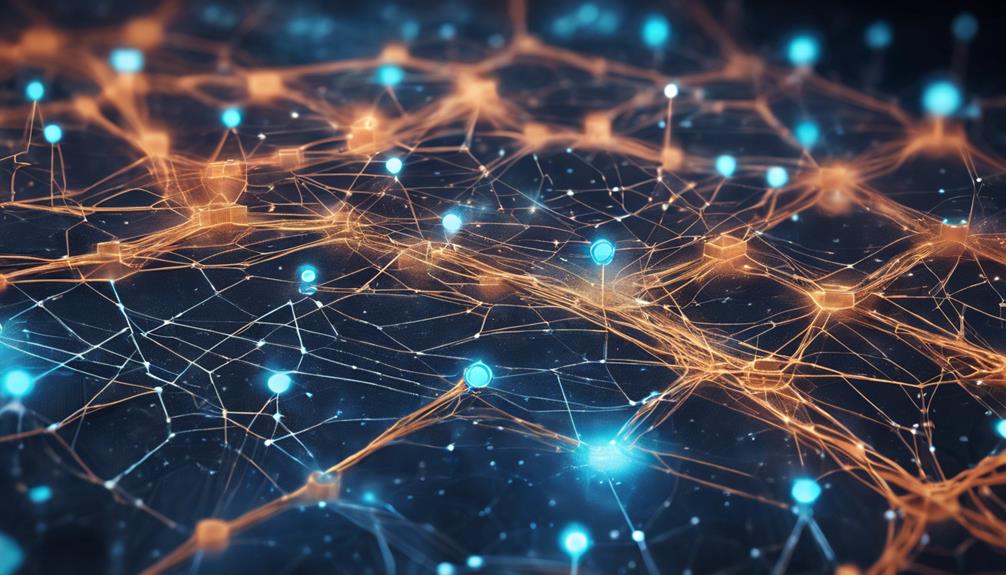
AI and machine learning are transforming cybersecurity, bolstering threat detection, incident response, and security operations. With the evolution from rule-based to deep learning systems, AI now excels in detecting anomalies and patterns. Utilizing historical data, it identifies attack patterns and clusters threats for streamlined response strategies. AI enhances incident response by analyzing data in real-time, automating actions, and providing insightful recommendations. By analyzing vast data sets, it swiftly adapts to dynamic environments, reducing response times. Future trends suggest a deeper integration of AI with security, focusing on user behavior, transparency, and autonomous security operations. The future of cybersecurity is AI-driven.
Key Takeaways
- AI enhances threat detection by analyzing vast data for patterns and anomalies.
- Machine learning identifies known attack patterns for proactive defense.
- Automation of security tasks improves incident response times and efficiency.
- Real-time anomaly detection aids in swift response to evolving threats.
- Integration of AI and ML revolutionizes cybersecurity operations for enhanced protection.
Evolution of AI in Cybersecurity
The evolution of AI in cybersecurity traces back to the mid to late 1980s when early rule-based systems were first utilized for anomaly detection. Since then, the landscape of security measures has been greatly transformed by the integration of machine learning algorithms.
This evolution has been essential in combating advanced cyber threats through enhanced threat detection capabilities. In the late 2000s, supervised learning algorithms played a pivotal role in boosting the accuracy of threat detection systems.
However, it was the advent of deep learning in the 2010s that truly revolutionized cybersecurity. Deep learning enabled the recognition of intricate patterns, providing a more robust defense against evolving threats.
As AI and machine learning technologies continue to progress, they play a crucial role in fortifying security measures across various sectors. The ongoing evolution in anomaly detection, pattern recognition, and overall threat detection capabilities underscores the importance of AI in enhancing cybersecurity protocols.
Machine Learning Techniques for Threat Detection
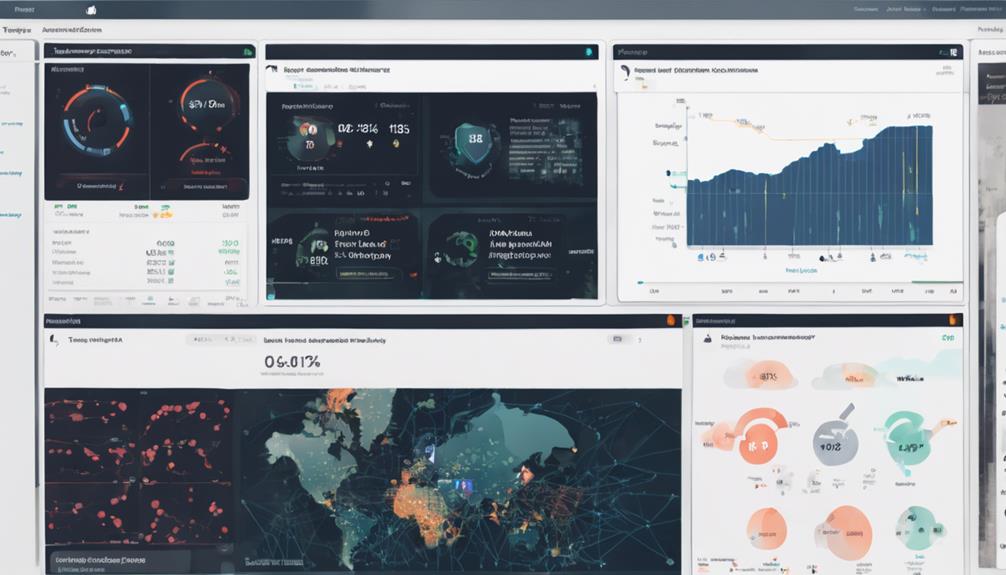
Machine learning techniques employed in cybersecurity play a pivotal role in identifying and mitigating potential threats within network environments. By leveraging AI algorithms to analyze network traffic, cybersecurity systems can proactively detect anomalies and unusual behavior, enhancing threat intelligence for a more secure environment.
These techniques utilize historical data to identify patterns and trends, enabling the detection of known attack patterns and clustering potential threats based on complexity for better risk assessment. Through machine learning, cybersecurity professionals can build a hierarchy of threats, allowing for a more efficient response to cyber threats.
This approach not only aids in threat detection but also helps in improving overall security posture by staying ahead of emerging threats. By embracing machine learning for threat detection, organizations can greatly enhance their cybersecurity defenses and safeguard their digital assets more effectively.
Enhancing Incident Response With AI
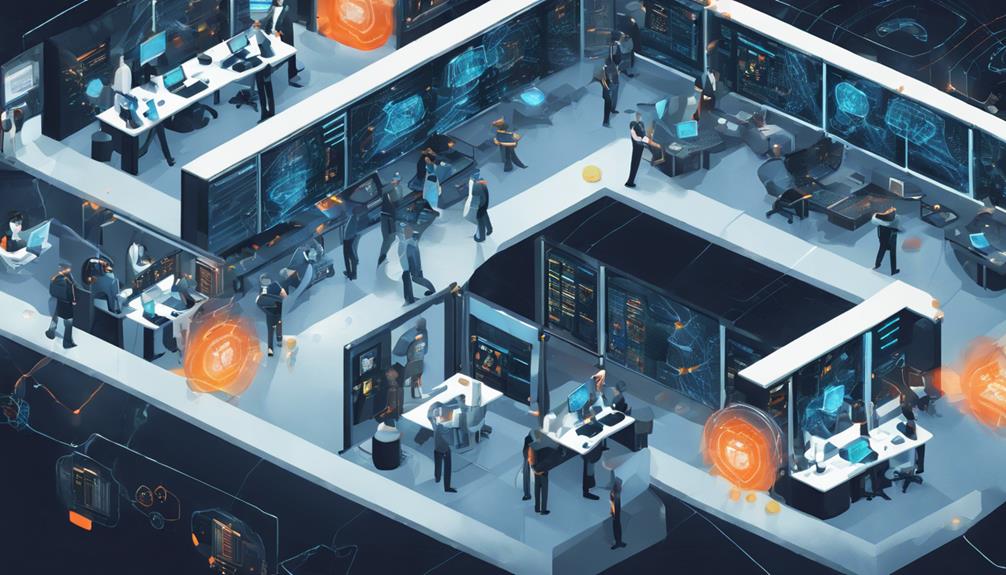
Moreover, utilizing AI technologies in incident response revolutionizes the efficiency and effectiveness of handling security breaches by leveraging advanced data analysis capabilities. Machine learning algorithms embedded in AI-powered systems can detect anomalies in real-time, allowing for swift identification and response to potential threats.
This automation of response actions based on predefined rules streamlines incident resolution processes, ensuring a proactive approach to cybersecurity incidents. Additionally, AI can prioritize alerts and escalate them to the appropriate stakeholders, facilitating efficient incident management by focusing resources where they are most needed.
Moreover, AI enhances decision-making during incident response by providing valuable insights and recommendations drawn from past incidents. By learning from historical data, AI continuously improves incident response strategies, making them more effective and adaptive to evolving cyber threats.
Incorporating AI into incident response not only boosts the speed of resolution but also enhances the overall security posture of organizations.
Leveraging AI in Security Operations
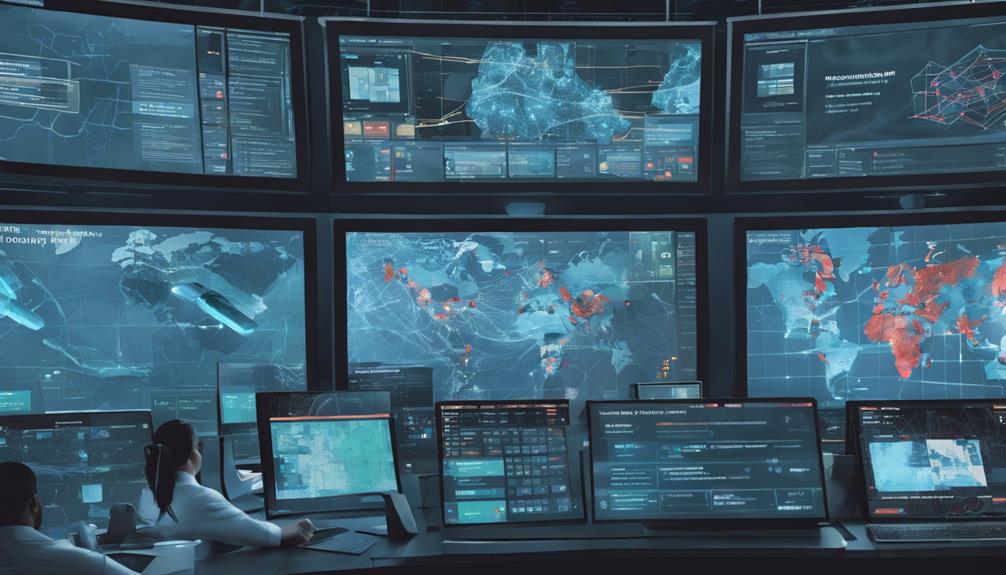
Incorporating artificial intelligence (AI) into security operations revolutionizes threat detection and response capabilities. By leveraging machine learning (ML) algorithms, AI can analyze vast amounts of data to identify patterns of suspicious behavior, enabling proactive threat mitigation. This ability to quickly identify potential threats in networks enhances overall security measures greatly.
Furthermore, AI automates repetitive security tasks, allowing human analysts to focus on more complex threats that require human intervention.
Security operations centers (SOCs) powered by AI can adapt to dynamic environments in real-time, leading to faster incident response times and an improved cybersecurity posture overall.
Future Trends in AI for Cybersecurity
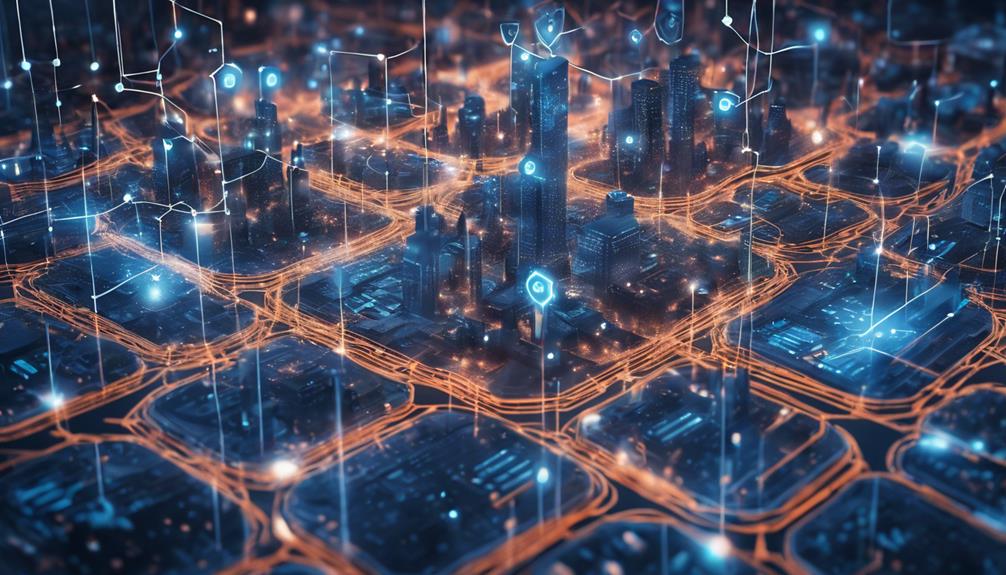
The future trajectory of AI in cybersecurity entails the evolution towards countering advanced cyber threats through innovative technologies and strategies. As the landscape of cybersecurity continues to evolve, here are three key trends shaping the future of AI in cybersecurity:
- Integration with Diverse Security Aspects:
AI and Machine Learning are increasingly being integrated with IoT, cloud security, and endpoint protection to enhance overall cybersecurity defenses and enable proactive threat detection in the face of large volumes of data.
- Significant User Behavior Analysis:
AI plays a significant role in analyzing user behavior patterns to detect anomalies and potential threats, enabling cybersecurity professionals to conduct more effective threat analysis and respond promptly to adversarial attacks.
- Explainable AI and Autonomous Security Operations:
The adoption of Explainable AI is gaining momentum, improving transparency in complex machine learning models. Additionally, AI-driven autonomous security operations centers (ASOCs) are on the rise, automating threat response mechanisms and policy enforcement while facilitating collaboration between AI systems and human experts to develop adaptive cybersecurity strategies.
Frequently Asked Questions
How Is AI and Machine Learning Used in Cyber Security?
AI and machine learning in cybersecurity entail anomaly detection, predictive analytics, phishing detection, automated threat response, and vulnerability management. These technologies enhance threat detection, predict cyber-attacks, and improve overall security by efficiently identifying potential threats and mitigating risks.
Can I Combine AI and Cyber Security?
Yes, the integration of AI in cybersecurity enhances threat detection, response, and mitigation capabilities. Leveraging AI technologies automates tasks, predicts cyber threats, and improves overall security posture. Combining AI with cybersecurity is a recommended approach for robust protection.
Will AI and ML Play a Role in the Future of Cybersecurity?
AI and ML are poised to have a substantial impact on the future of cybersecurity by enhancing defense mechanisms, predicting threats, and improving security postures. These technologies will play a pivotal role in mitigating risks and safeguarding critical infrastructure.
What Is the Intersection of AI and Cybersecurity?
In the intricate dance between artificial intelligence and cybersecurity, AI amplifies threat detection capabilities by unraveling patterns in the digital tapestry, revealing hidden vulnerabilities and fortifying defenses with predictive prowess.
Conclusion
To sum up, the integration of AI and machine learning in cybersecurity is a game-changer, revolutionizing the way organizations detect and respond to threats.
The power of these technologies to analyze vast amounts of data and adapt to evolving threats is unparalleled.
As we move forward, the future of cybersecurity will be shaped by the continued advancements in AI, creating a more secure digital environment for all.
The possibilities are endless, and the impact is immeasurable.
Advanced Cybersecurity
Cybersecurity Using AI: Modern Techniques for Enhanced Protection
Optimize your cybersecurity defenses with cutting-edge AI technology, revolutionizing protection against cyber threats and bolstering your security posture.
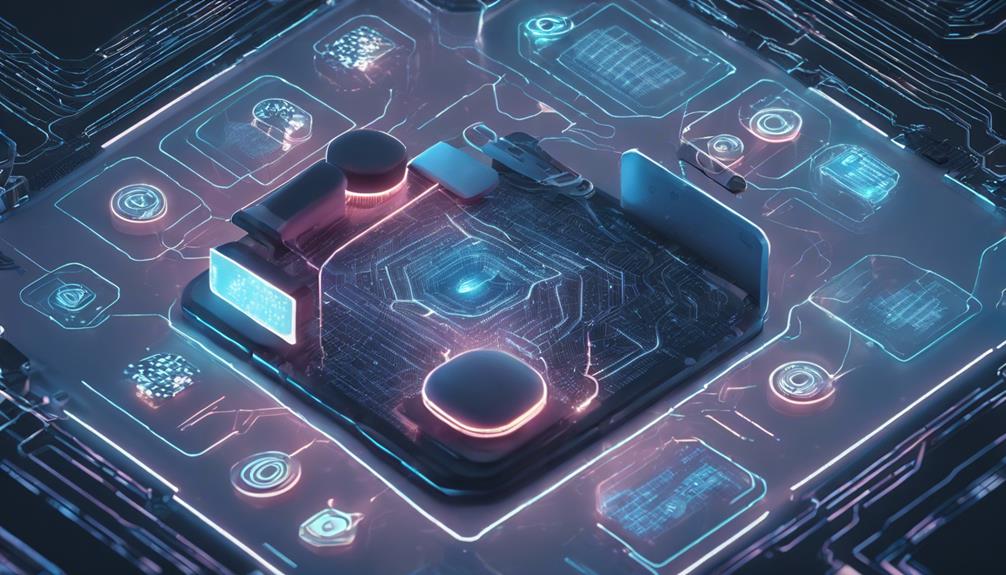
Enhance your cybersecurity with AI, reshaping protection against evolving cyber threats. AI boosts threat detection and response with machine learning technology. Behavioral analysis and anomaly detection heighten security vigilance. Predictive analytics prioritize threat action, automating tasks for efficiency. Proactive defense through AI boosts vulnerability management effectiveness. Swift identification of unknown threats secures systems effectively. Real-time monitoring and adaptive access control fortify cybersecurity posture. Dive deeper into AI's impact on modern protection techniques for thorough cybersecurity enhancement.
Key Takeaways
- AI enhances threat detection with machine learning algorithms.
- Predictive analytics prioritize potential threats for proactive defense.
- Automation streamlines tasks and manages vulnerabilities effectively.
- AI identifies unknown threats swiftly to bolster security measures.
- Integration of AI is crucial in the evolving threat landscape for robust protection.
AI in Cybersecurity Overview
AI in cybersecurity plays a pivotal role in revolutionizing threat detection and incident response through the utilization of machine learning algorithms. By harnessing the power of Artificial Intelligence (AI) and Machine Learning (ML), cybersecurity systems can efficiently detect anomalies and behavioral patterns that signal advanced threats.
Through anomaly detection and behavioral analysis, AI can handle complex security tasks that involve vast amounts of data analysis, enabling proactive defense mechanisms. Predictive analytics further enhances these capabilities by predicting potential threats and prioritizing them for immediate action.
The integration of AI in cybersecurity not only automates tasks but also streamlines incident response, providing real-time threat prevention. By reducing the workload on security teams, AI allows organizations to improve their overall cybersecurity effectiveness.
This modern approach to cybersecurity is essential in combating the ever-evolving landscape of cyber threats, where diverse data sources need to be analyzed thoroughly to ensure robust protection.
Importance of AI in Protection
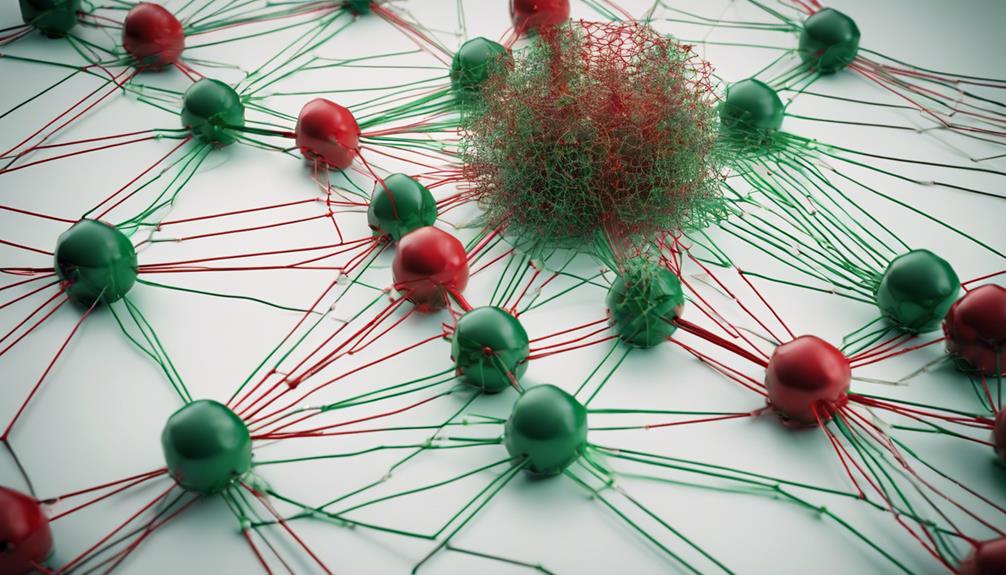
The significance of incorporating Artificial Intelligence technology in cybersecurity for heightened protection measures cannot be overstated. AI plays a pivotal role in cybersecurity by enhancing threat detection capabilities, improving response times, and enabling proactive defense through predictive analytics.
Leveraging AI allows for automating tasks, integrating diverse data sources, and efficiently managing vulnerabilities to prioritize threats effectively. By identifying unknown threats and analyzing large volumes of data swiftly, AI guarantees overall security in the digital landscape.
Implementing AI in cybersecurity not only reduces the workload for security teams but also enhances the ability to address complex cyber threats with precision. In today's ever-evolving threat landscape, where cyberattacks are becoming more sophisticated, the adoption of AI is essential for staying ahead of potential risks and safeguarding sensitive information effectively.
Threat Detection With AI
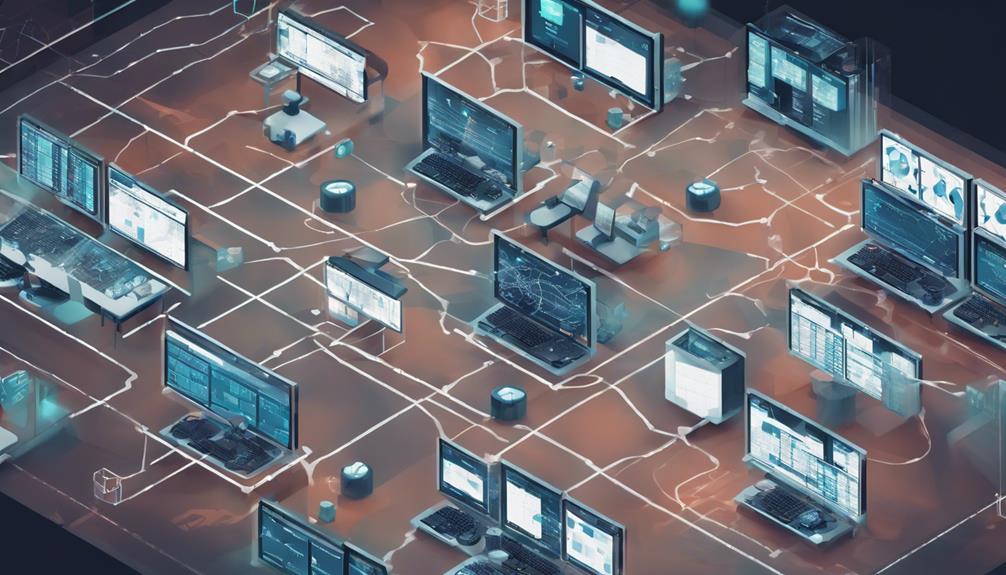
How does artificial intelligence revolutionize threat detection in cybersecurity?
AI plays a pivotal role in enhancing threat detection by leveraging Machine Learning (ML) algorithms to analyze vast amounts of data in real-time. By identifying patterns and anomalies that signify potential threats, AI empowers cybersecurity professionals with automated incident response capabilities, greatly improving response time and accuracy.
Behavioral analysis through AI models enables the proactive identification of suspicious activities, bolstering defense against emerging threats. Additionally, AI solutions continuously monitor and analyze network data, allowing organizations to stay ahead of evolving threats.
AI for Vulnerability Management
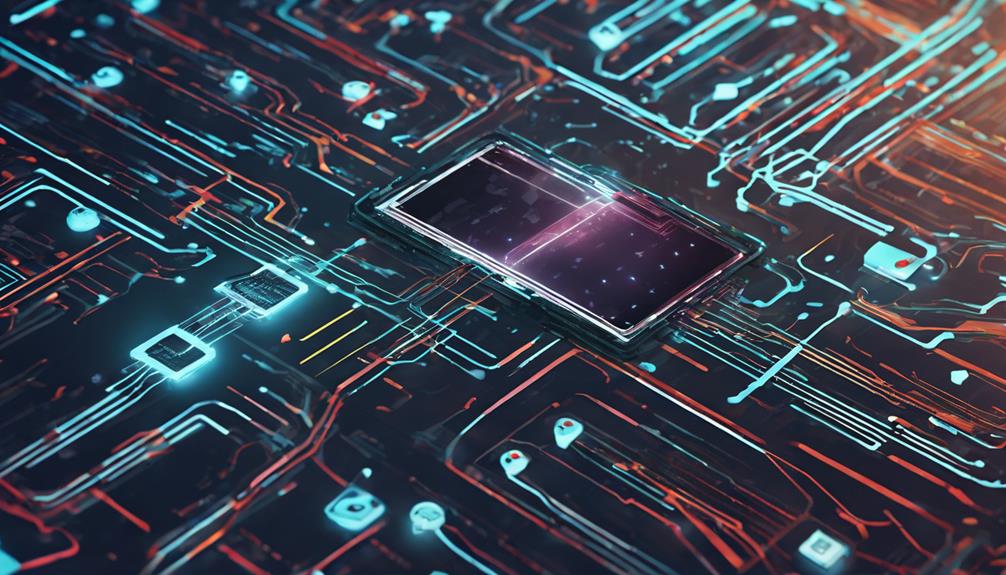
Utilizing advanced algorithms and automation, organizations leverage artificial intelligence to enhance the efficiency of identifying and prioritizing security weaknesses in vulnerability management. Machine learning algorithms play an important role in analyzing vast amounts of data to detect vulnerabilities in systems and networks. AI automates vulnerability scanning and assessment processes, greatly reducing the time required for manual analysis.
This automation not only saves time but also provides real-time insights into potential vulnerabilities, enabling proactive security measures to be implemented promptly. By addressing weaknesses before exploitation, vulnerability management with AI enhances the overall cybersecurity posture of an organization.
In the fast-evolving landscape of cybersecurity threats, utilizing AI for vulnerability management is a proactive approach that can help organizations stay ahead of potential security breaches. By incorporating AI-powered tools into their security strategies, organizations can fortify their defenses and protect against vulnerabilities effectively.
Enhanced Security Measures
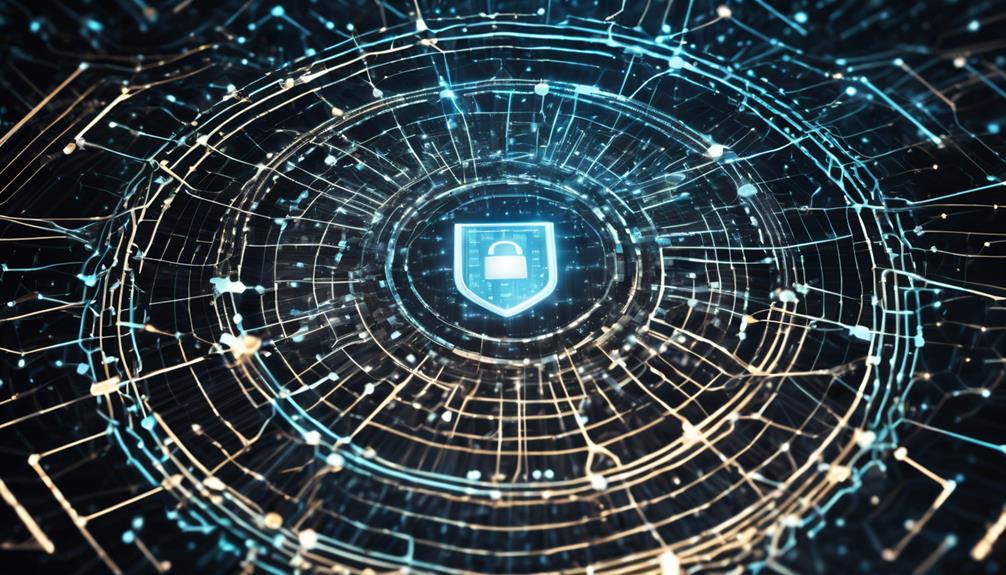
AI's role in cybersecurity extends beyond vulnerability management to encompass enhanced security measures that prioritize critical issues and automate response actions. By leveraging AI, organizations can enhance their defense against emerging threats through proactive threat management.
AI plays an important role in reducing the risk of cyber incidents by prioritizing critical security issues and swiftly fixing them. It automates response actions, enabling quick detection of anomalies and providing actionable intelligence on potential cyber threats.
Additionally, AI's ability to scan systems for risks, suggest security improvements, and support decision-making processes reinforces the least privilege principle, which limits access rights for users to only what are strictly required. This approach noticeably reduces the risk of data breaches and unauthorized access, ultimately enhancing overall cybersecurity posture.
Embracing AI-driven security measures empowers organizations to stay ahead of evolving threats and ensure robust protection against cyberattacks.
Accelerated Response Times
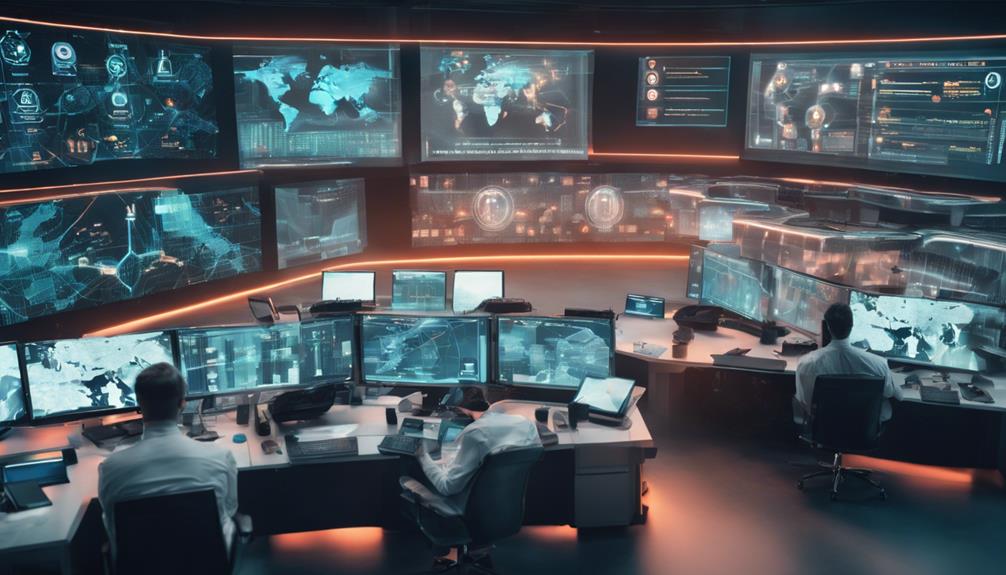
Accelerated response times in cybersecurity using AI offer a significant advantage to organizations.
Real-time threat detection guarantees immediate identification of potential risks, enabling swift action to prevent security breaches.
Automated incident analysis, coupled with instant security alerts, empowers teams to respond effectively, enhancing overall cybersecurity resilience.
Real-Time Threat Detection
Efficiency in identifying and responding to cybersecurity threats is greatly enhanced through the integration of artificial intelligence technologies. AI-powered systems enable real-time threat detection by swiftly analyzing vast amounts of data, leading to immediate threat identification.
This proactive defense mechanism allows for accelerated incident responses, prioritizing critical threats for automated actions. By continuously monitoring networks, endpoints, and applications, AI contributes to overall cybersecurity posture by reducing the time to detect and respond to cyber incidents.
Real-time threat detection with AI not only minimizes potential damages but also empowers organizations to take quick action against emerging threats. The combination of AI's immediate threat detection capabilities and automated responses strengthens the resilience of cybersecurity defenses.
Embracing AI in cybersecurity operations can greatly enhance the security posture of organizations, providing a robust shield against evolving cyber threats.
Automated Incident Analysis
Automated incident analysis plays a pivotal role in enhancing response times in cybersecurity operations. By utilizing AI algorithms, organizations can achieve real-time incident detection and rapid incident analysis, greatly reducing the mean time to detect and respond to cyber threats. This proactive approach enables quicker threat mitigation and enhances overall cybersecurity posture and security effectiveness.
AI-driven automated incident response can efficiently process and prioritize critical threats for immediate action, ensuring that the most pressing issues are addressed promptly. Compared to manual analysis, AI algorithms can process and analyze incidents within seconds, a task that would typically take human analysts hours or even days to complete. This acceleration in response times by up to 65% underscores the importance of automated incident analysis in maintaining a robust cybersecurity defense.
Incorporating automated incident analysis into cybersecurity strategies not only improves response times but also minimizes potential damages by swiftly identifying and addressing threats, ultimately fortifying the organization's security resilience.
Instant Security Alerts
Utilizing AI-powered systems for generating instant security alerts greatly enhances organizations' ability to swiftly respond to potential cyber threats. AI systems can analyze vast amounts of data in real-time, allowing for the immediate detection of suspicious activities that may indicate security incidents. This proactive approach notably reduces response times to cyber threats, sometimes by up to 65%, enabling organizations to take timely action for enhanced protection.
AI-powered tools provide real-time monitoring for swift detection of security incidents.
Instant security alerts offer actionable insights to respond effectively to cyber threats promptly.
Accelerated response times facilitated by AI systems lead to quicker containment and mitigation of potential damages.
Authentication Security With AI
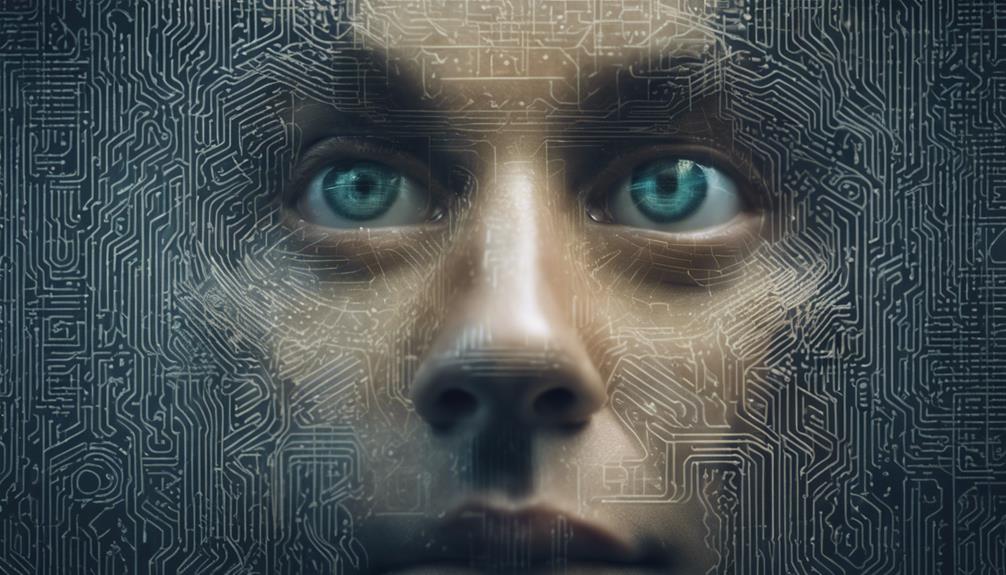
Enhancing authentication security with AI involves utilizing advanced biometric authentication methods. This includes analyzing user behavior for potential threats and implementing adaptive access controls.
By integrating AI-driven solutions, organizations can verify user identities based on unique behavioral traits like typing speed or mouse movements. This helps in bolstering security measures against unauthorized access attempts.
These innovative approaches provide a proactive defense mechanism that adapts to evolving cybersecurity risks. They ensure robust multi-factor authentication protocols for heightened protection.
AI-Driven Biometric Authentication
AI-driven biometric authentication leverages unique biological traits such as fingerprints or facial recognition to provide secure access to systems and data. This innovative approach enhances security measures by reducing the risk of unauthorized access through impersonation.
By utilizing advanced AI algorithms, biometric data is analyzed to accurately and swiftly verify user identities, thereby offering a seamless and efficient authentication process. The integration of AI in biometric authentication guarantees a high level of security, safeguarding against identity theft and fraudulent activities.
Continuous improvements in AI technology further enhance the reliability and precision of biometric authentication systems, reinforcing their efficacy in protecting sensitive information.
- Enhanced Security: Utilizes unique biological traits for secure access.
- Efficient Verification: AI algorithms analyze biometric data to verify identities.
- Protection Against Fraud: Offers a high level of security to prevent identity theft and fraud.
Behavioral Analysis for Security
Behavioral analysis for security, bolstered by artificial intelligence technology, plays a pivotal role in enhancing authentication security measures.
By utilizing AI-driven behavioral analysis, organizations can effectively monitor and detect unusual user behavior, reducing the reliance on traditional authentication methods like passwords. This proactive approach enables the real-time identification of suspicious activities, allowing for immediate response and enhanced security measures.
Through the detection of anomalies in user behavior, AI models can prevent unauthorized access to sensitive information, safeguarding against potential breaches. Additionally, behavioral analysis powered by AI offers a more secure and efficient authentication process, greatly minimizing the risk of fraudulent activities.
Adaptive Access Control
Authentication security in cybersecurity is greatly strengthened by the implementation of adaptive access control, leveraging AI technology to analyze and adjust user behavior in real-time. This advanced approach enhances authentication security by dynamically adapting access permissions based on user behavior, providing a proactive defense against potential security threats.
Key points to note about adaptive access control include:
- AI algorithms assist in identifying unusual user patterns that may indicate security risks.
- Adaptive access control systems continuously monitor user access patterns to detect anomalies and prevent unauthorized access attempts.
- By dynamically adjusting security measures based on real-time user behavior, adaptive access control guarantees a more robust defense against cyber threats.
Through the utilization of AI-driven technologies, organizations can fortify their cybersecurity posture by implementing adaptive access control mechanisms that respond intelligently to evolving user behaviors and potential security challenges.
Automation in Cybersecurity
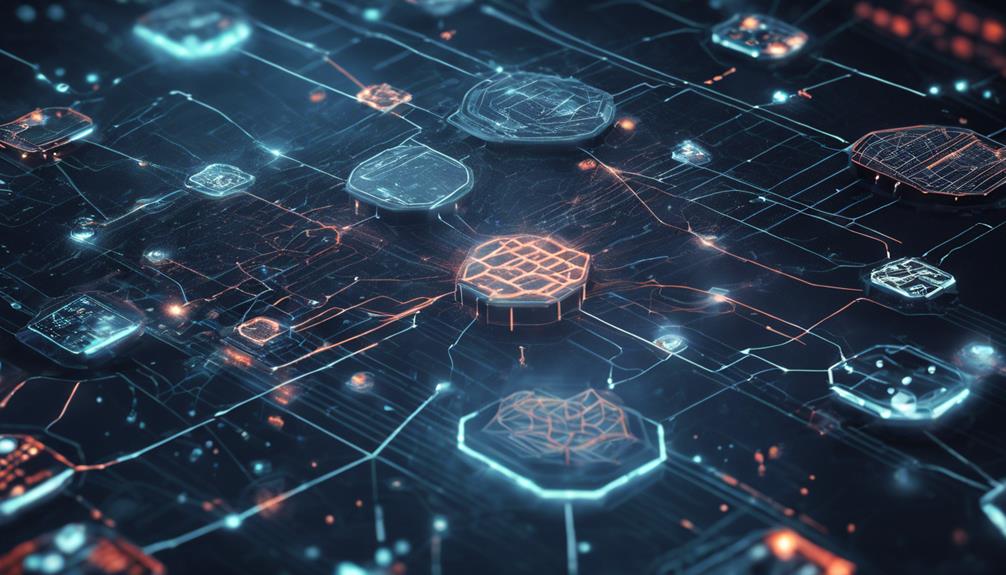
Utilizing automation in cybersecurity processes enhances operational efficiency and response capabilities in addressing cyber threats. AI-driven automation plays an essential role in securing data by enabling real-time monitoring and swift incident response.
With the ability to consolidate and analyze vast amounts of security data efficiently, AI automation streamlines vulnerability assessments and enhances overall security operations' effectiveness. By automating log analysis and vulnerability assessments, security teams can minimize manual tasks, allowing them to focus on more complex cybersecurity challenges.
Additionally, AI automation in cybersecurity not only improves efficiency but also contributes to cost reduction by optimizing the resources required for monitoring and incident response. Embracing AI-driven automation in cybersecurity operations is vital for staying ahead of evolving cyber threats, ensuring robust protection for sensitive data and critical systems.
Frequently Asked Questions
How Does AI Enhance Cybersecurity?
AI enhances cybersecurity by leveraging advanced algorithms to analyze data, detect threats efficiently, automate incident responses, and predict potential risks. It enables proactive defense by staying ahead of evolving threats and streamlining security operations for professionals.
What Is an Example of Cyber Security in Ai?
An example of cybersecurity in AI involves utilizing machine learning algorithms to analyze network data for threat detection. By identifying patterns and anomalies, AI can proactively prevent unknown threats, continuously improving security measures to mitigate cyber risks effectively.
How Can AI Help in Data Protection?
AI aids data protection by analyzing vast data sets to detect anomalies and threats. Machine learning algorithms identify patterns indicating security risks. Real-time monitoring tracks user behavior for unauthorized access. AI automates security tasks, prioritizes alerts, and enhances data protection measures.
Can Cyber Security Be Replaced by Ai?
While AI can bolster cybersecurity defenses, it cannot entirely replace human expertise. Just as a ship needs both its compass and captain, cybersecurity requires the synergy of AI's efficiency with human decision-making for effective threat mitigation and strategic defense.
Conclusion
To sum up, AI technology in cybersecurity provides advanced protection against threats, enhances vulnerability management, and ensures accelerated response times. By implementing AI-driven security measures, organizations can strengthen their defenses and safeguard sensitive data.
It is ironic that the very technology used to create cyber threats can also be leveraged to combat them effectively. Embracing AI in cybersecurity is not just a trend but a necessary step towards securing digital assets in the modern age.
-
Hackathons5 months ago
Do Hackathons Look Good on Resume: Participating in Hackathons Is an Impressive Accomplishment to Highlight on Resumes.
-
Hackathons5 months ago
Remote Hackathons: Virtual Hackathons Enable Global Teams to Collaborate Remotely.
-
Hackathons5 months ago
MIT Hackathons: the Prestigious MIT Hosts Innovative Student-Led Hackathons in Boston.
-
Ethical Hacking4 months ago
Which Is Best: Cyber Security or Ethical Hacking?
-
Ethical Hacking4 months ago
What Is Better: Computer Science or Ethical Hacking?
-
Hackathons5 months ago
How to Find Hackathons: Discover Upcoming Hackathon Opportunities Through Community Forums and Listing Platforms.
-
Technology3 months ago
Global Shutdown: Drastic Microsoft Outage Impact
-
Hackathons5 months ago
Internal Company Hackathons: Corporations Leverage Internal Hackathons to Crowdsource Ideas and Drive Innovation.