Advanced Cybersecurity
AI/ML in Cybersecurity: Leveraging Machine Learning for Better Defense
AI and machine learning revolutionize cybersecurity, offering proactive defense strategies and real-time threat detection – discover how organizations stay ahead of cyber threats.
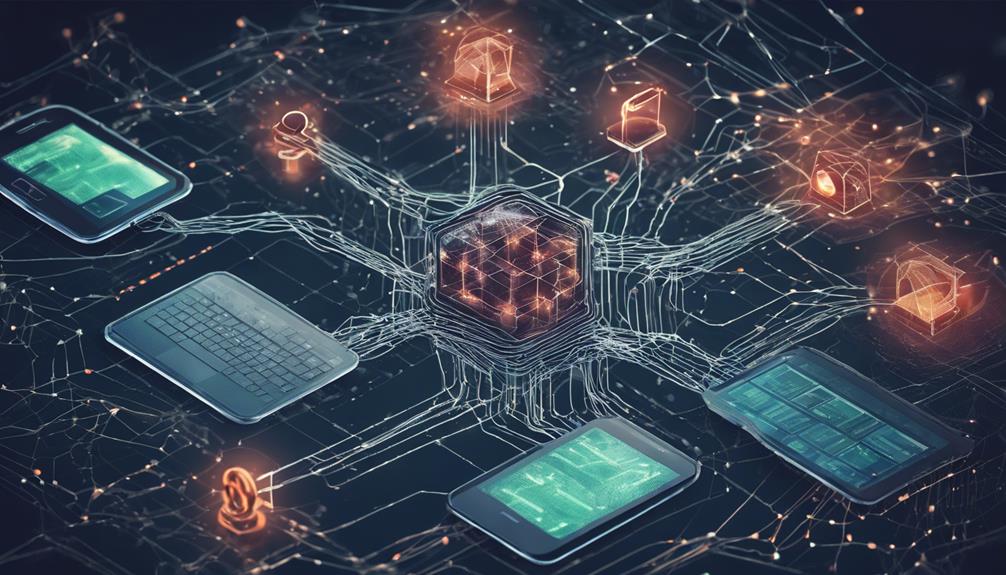
AI and machine learning are transforming cybersecurity, enhancing defense mechanisms through real-time threat detection and automating tasks for better operational efficiency. By analyzing vast datasets, proactive defense strategies are devised, with a focus on pattern recognition and anomaly classification. This transformative impact is seen in modern defense practices, with anomaly detection techniques, behavior analysis for threat detection, and predictive analytics reinforcing security measures. Leveraging these technologies offers a proactive approach to cybersecurity, empowering organizations to anticipate and mitigate potential threats effectively. Embracing AI/ML in cybersecurity guarantees a robust defense against evolving cyber threats. Learn more about these cutting-edge advancements.
Key Takeaways
- AI enhances defense with real-time threat detection.
- ML automates tasks for operational efficiency.
- Analyzing vast datasets for proactive defense.
- ML plays a key role in anomaly classification.
- Transforming modern defense practices with AI/ML.
Importance of Ai/Ml in Cybersecurity
The significance of AI and ML in cybersecurity lies in their ability to bolster defense mechanisms through real-time threat detection and response capabilities. By harnessing the power of AI and ML algorithms, organizations can enhance their cybersecurity posture by automating tasks, improving operational efficiency, and strengthening overall security strategies.
These technologies excel in analyzing vast datasets to identify potential risks, contributing to more proactive defense strategies against evolving cyber threats. AI and ML play an important role in pattern recognition, anomaly classification, and predicting cyber threats based on historical data, enabling security teams to stay ahead of malicious actors.
Leveraging these technologies not only enhances the effectiveness of security operations centers (SOCs) but also helps in automating mundane tasks, allowing cybersecurity professionals to focus on more strategic initiatives. Ultimately, the value of AI and ML in cybersecurity is demonstrated through achieving good security outcomes with limited resources, highlighting the transformative impact these technologies have on modern defense practices.
Anomaly Detection Using Machine Learning
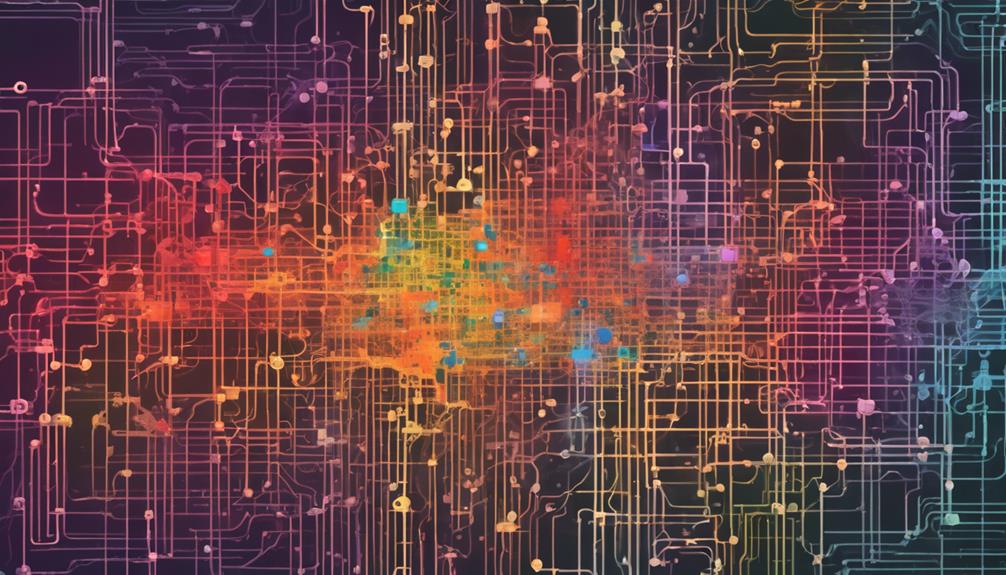
Utilizing machine learning techniques allows for the identification of abnormal patterns in systems or networks, enhancing cybersecurity defenses through anomaly detection. By leveraging machine learning algorithms trained on substantial datasets, organizations can effectively distinguish between benign deviations and potential threats.
AI-driven anomaly detection continuously improves by learning from data, refining detection capabilities over time. Monitoring data access and transfer patterns in cloud storage platforms enables prompt flagging of potential data breach attempts, bolstering overall security measures.
In contrast, traditional systems may inundate security teams with numerous false alarms when detecting any deviation, underscoring the necessity for AI-driven anomaly detection solutions. These advanced technologies not only reduce the burden of false alarms but also enhance the accuracy and efficiency of threat detection processes.
Behavior Analysis for Threat Detection
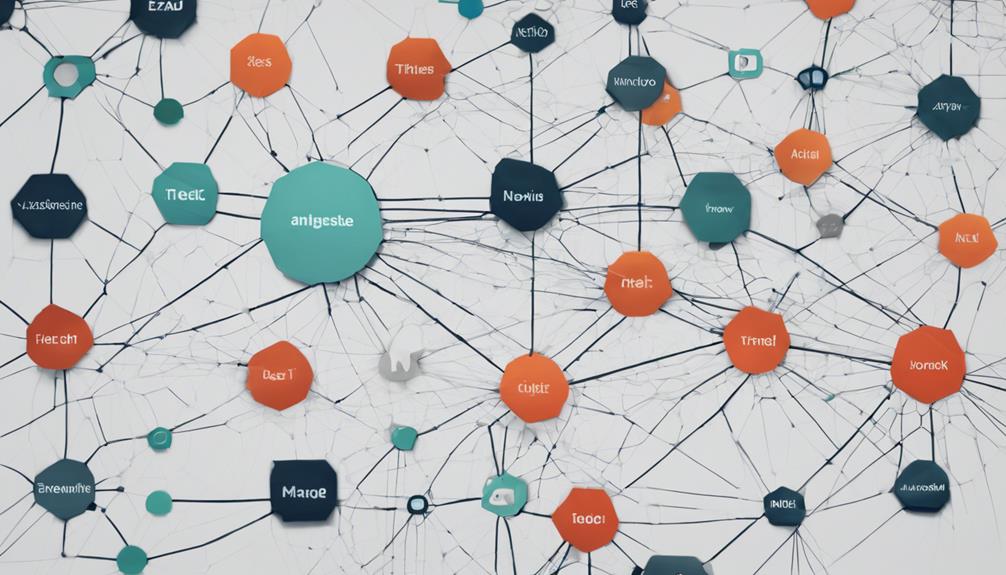
Behavior analysis in cybersecurity involves profiling user interactions to detect abnormal patterns, such as odd-hour logins or unauthorized data access.
AI algorithms create unique digital fingerprints for each user, enabling the identification of anomalies like high-level executives accessing sensitive data late at night.
Anomaly Detection Techniques
An essential component of modern cybersecurity strategies involves employing advanced anomaly detection techniques to identify abnormal patterns within systems or networks. Behavior analysis, utilizing AI-driven anomaly detection, plays a critical role in this process. By monitoring data access and transfer patterns, anomalies such as odd-hour logins or unusual data access can be swiftly identified.
Machine learning algorithms, particularly when trained on substantial datasets, excel at discerning benign deviations from potential security threats, thereby enhancing anomaly detection in cybersecurity. Over time, AI-driven anomaly detection becomes more effective as it learns from data, continuously refining its capabilities to detect threats proactively.
Cloud storage platforms, in particular, benefit from behavior analysis, promptly flagging potential data breach attempts by analyzing user interactions. The synergy between anomaly detection and behavior analysis underlines the importance of leveraging cutting-edge technologies to bolster cyber defense mechanisms and protect crucial digital assets.
Predictive Modeling Applications
Predictive modeling applications play a pivotal role in cybersecurity by leveraging AI algorithms to proactively analyze user behaviors for threat detection and prevention. Utilizing predictive analytics and ML models, behavior analysis focuses on detecting anomalies in user behavior that could indicate potential security threats. By employing AI-driven behavior analysis, cybersecurity systems can swiftly identify deviations such as odd-hour logins or unusual data access patterns.
These AI systems create digital fingerprints that help in spotting abnormal user behaviors, like accessing sensitive data at irregular times. Promptly flagging potential security breaches, such as high-level executives accessing critical data late at night, is essential in preventing account takeovers and other cyber threats.
The ability to detect anomalies in user behavior efficiently enables cybersecurity teams to understand how legitimate users typically interact with systems and respond effectively to abnormal activities. This proactive approach to threat detection enhances overall cybersecurity defenses and reduces the risk of potential breaches.
User Behavior Profiling
Utilizing advanced AI algorithms, user behavior profiling in cybersecurity plays a critical role in proactively identifying potential security threats through the analysis of digital interactions and deviations.
By creating digital fingerprints for users, behavior analysis tracks interactions and detects deviations, such as odd-hour logins or accessing unfamiliar data segments, promptly flagging unusual behavior.
The aim is to prevent security breaches by profiling how legitimate users typically interact with systems and identifying abnormal activities using AI algorithms. For instance, spotting a high-level executive accessing sensitive data late at night could signal a potential account takeover attempt.
Furthermore, behavior analysis is instrumental in detecting insider threats and unauthorized access by continuously monitoring user behavior patterns.
Predictive Analytics in Cybersecurity Defense
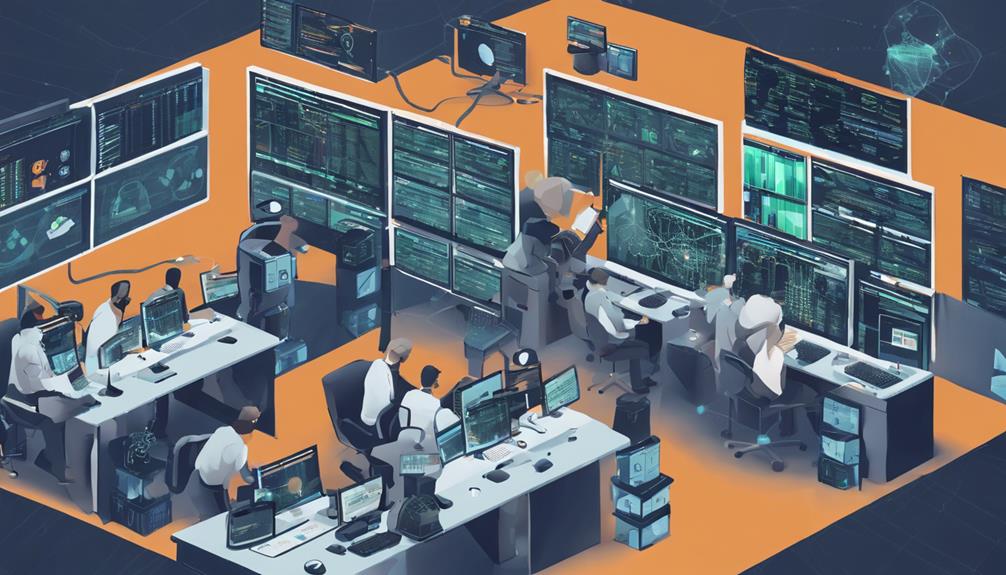
Predictive analytics in cybersecurity defense offers a proactive approach to strengthening security measures.
By utilizing threat detection models, anomaly detection techniques, and behavioral analysis applications, organizations can anticipate and mitigate potential cyber threats before they materialize.
These tools leverage historical data to forecast future attack trends and patterns, empowering companies to stay ahead of evolving cybersecurity challenges.
Threat Detection Models
Implementing advanced AI and ML technologies in cybersecurity defense enables organizations to enhance threat detection models through the analysis of historical data. By utilizing machine learning and predictive analytics, companies can identify patterns and trends from past security incidents to predict potential future threats.
Insights gained from this predictive analytics process empower organizations to proactively strengthen their cybersecurity defenses against evolving cyber threats.
These threat detection models leverage historical data to predict potential future attack types, allowing for the implementation of proactive defense measures. By staying ahead of cyber threats through the use of predictive analytics, organizations can better prepare themselves and mitigate potential risks before they escalate.
This proactive approach not only enhances overall cybersecurity posture but also helps in safeguarding sensitive data and critical systems from malicious actors. Leveraging threat detection models powered by machine learning and predictive analytics is a pivotal step towards bolstering cybersecurity defenses in today's constantly evolving threat landscape.
Anomaly Detection Techniques
Anomaly detection techniques in cybersecurity defense leverage machine learning to identify deviations from normal operation patterns in systems. By employing machine learning algorithms, cybersecurity experts can effectively distinguish between benign deviations and potential threats, greatly enhancing detection accuracy.
Predictive analytics plays a vital role in anomaly detection, utilizing historical data to predict and prevent future cyber threats before they materialize. Monitoring data access and transfer patterns in cloud storage platforms is a key strategy in promptly flagging potential data breach attempts, enabling proactive cybersecurity measures.
Moreover, AI-driven anomaly detection continuously improves over time by learning from data and refining detection capabilities. This iterative process enhances the effectiveness of anomaly detection systems, making them more adept at identifying and mitigating evolving cyber threats.
Behavioral Analysis Applications
Utilizing AI algorithms, behavioral analysis in cybersecurity involves creating digital fingerprints to track user interactions and identify deviations for enhanced threat detection capabilities. By detecting abnormal behavior, such as odd-hour logins or unusual data access patterns, AI-driven behavioral analysis can promptly flag anomalies for potential security threats.
This essential defense approach is vital for preventing security breaches, especially when it comes to identifying abnormal user activities like high-level executives accessing sensitive data at irregular times.
Predictive analytics plays a significant role in cybersecurity by utilizing AI and ML to analyze past incidents, predict future threats, and enhance proactive defense strategies. AI-powered behavioral analysis tools are designed to prevent security breaches effectively by swiftly identifying and responding to abnormal user activities.
Enhancing Phishing Detection With AI
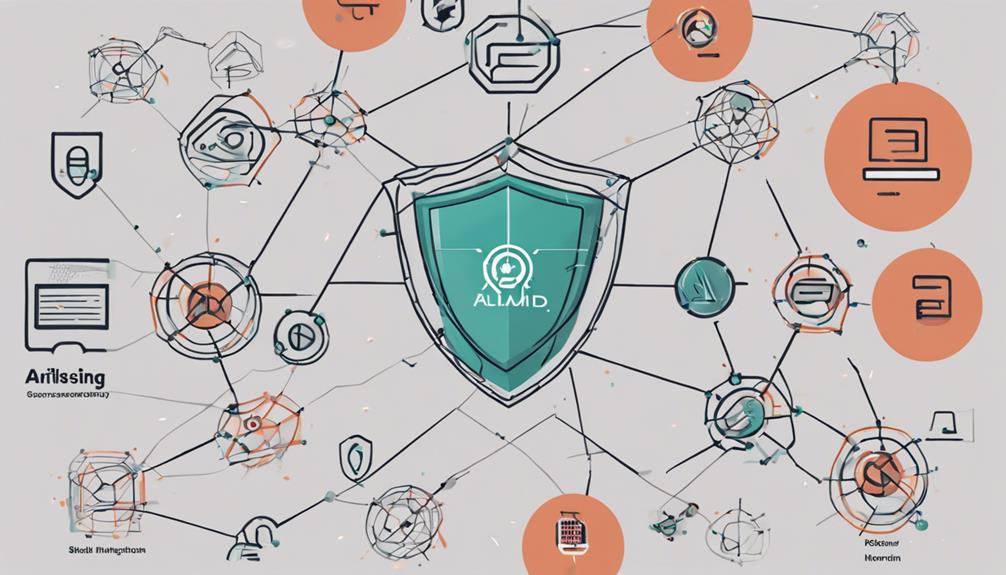
AI technology plays an essential role in enhancing the detection of phishing attempts, particularly in emails. Machine learning algorithms, a subset of AI techniques, have revolutionized cybersecurity by swiftly identifying and flagging suspicious emails. These algorithms are trained on extensive datasets to distinguish between legitimate and phishing emails, enabling them to detect subtle indicators of phishing attempts that might go unnoticed by traditional methods. By analyzing email structures for inconsistencies, AI systems can efficiently identify potential phishing emails, even those designed to evade detection through sophisticated techniques. This capability is important in preventing costly mistakes and safeguarding organizations against cyber threats. Below is a table highlighting the key benefits of using AI in phishing detection:
Benefits of AI in Phishing Detection |
---|
Efficiently detects subtle indicators of phishing attempts |
Trained on vast datasets to differentiate between legitimate and phishing emails |
Identifies inconsistencies in email structures to flag potential phishing emails |
Detects sophisticated phishing attempts through AI-driven solutions |
Swiftly identifies and flags suspicious emails to prevent cybersecurity breaches |
Automated Threat Hunting Techniques
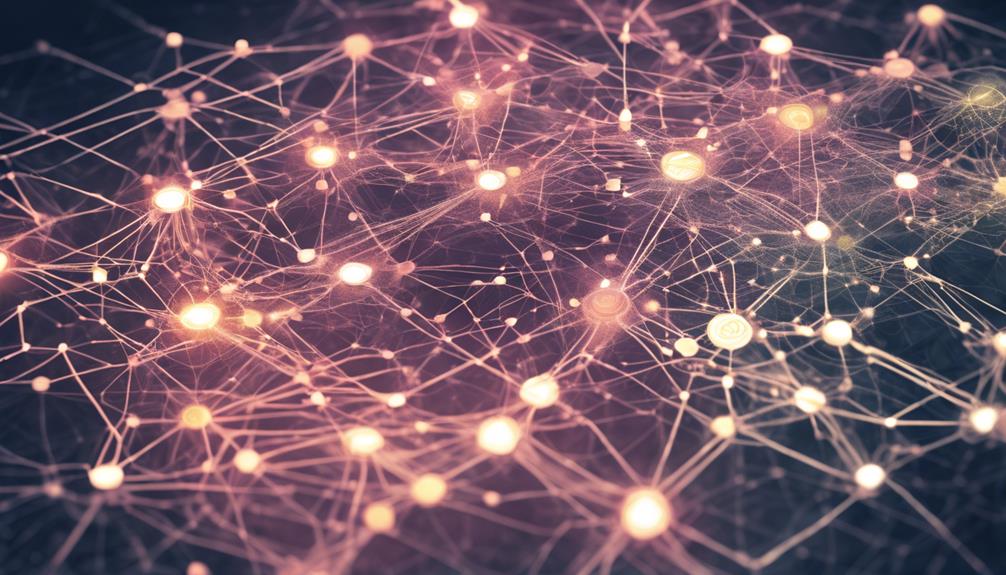
Enhancing cybersecurity defenses, automated threat hunting techniques involve actively scanning networks to detect patterns, anomalies, or behaviors indicating potential threats.
By leveraging AI-driven systems, organizations can achieve continuous monitoring capabilities, enabling the swift detection and neutralization of threats at their earliest stages.
Proactive threat hunting tools, powered by AI, can identify patterns resembling past attacks, facilitating early isolation and investigation to prevent potential breaches.
Constant vigilance through AI-powered threat hunting greatly enhances the cybersecurity posture by detecting and neutralizing threats before they cause damage.
Automating the process of seeking out potential threats, AI-driven threat hunting shifts the focus from reactive to proactive defense strategies, ensuring a more robust security framework.
Frequently Asked Questions
How Are AI and Machine Learning Improving Cybersecurity?
AI and machine learning are enhancing cybersecurity by efficiently analyzing data, automating incident responses, improving response times, and enabling proactive defense. These technologies play a crucial role in identifying unknown threats, managing vulnerabilities, and predicting and countering threats in cyber defense.
How AI Can Be Used in Cyber Security Defences?
In the domain of cybersecurity defenses, AI plays a pivotal role by enhancing anomaly detection, behavior analysis, predictive analytics, automated threat hunting, and phishing detection. Leveraging machine learning, AI fortifies networks against evolving cyber threats.
What Is Leveraging AI in Cybersecurity?
Leveraging AI in cybersecurity involves harnessing advanced technologies to analyze data, detect patterns, and identify potential threats in real-time. This proactive approach enables organizations to enhance their defense strategies, improve response times, and bolster overall security posture.
What Is Aiml in Cyber Security?
AI/ML in cybersecurity refers to utilizing artificial intelligence and machine learning technologies to bolster defense mechanisms against cyber threats. By applying data analytics and pattern recognition, AI/ML aids in identifying anomalies and potential security breaches for enhanced protection.
Conclusion
To summarize, the integration of AI and ML in cybersecurity is essential for enhancing defense mechanisms against evolving threats. By leveraging machine learning for anomaly detection, behavior analysis, predictive analytics, phishing detection, and automated threat hunting, organizations can strengthen their security posture and stay ahead of malicious actors.
The potential for AI and ML to revolutionize cybersecurity is nothing short of extraordinary, offering a powerful toolset for protecting sensitive data and mitigating risks in the digital landscape.
Advanced Cybersecurity
AI Impact on Cybersecurity Jobs: What to Expect
Yearning for insights on how AI is reshaping cybersecurity roles? Discover the evolving landscape and skills needed to stay ahead.
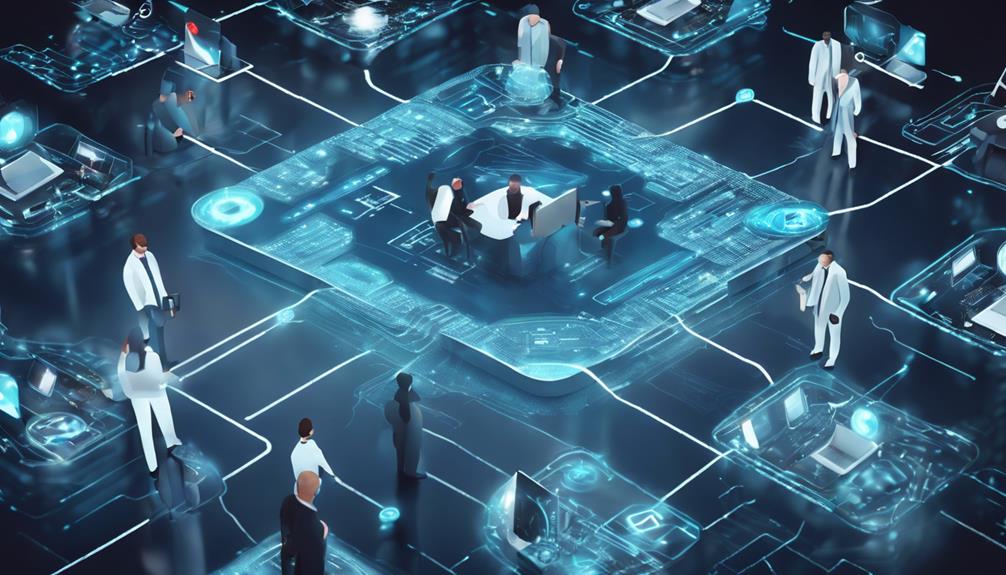
As AI transforms cybersecurity, expect job roles to evolve, requiring updated skills and responsibilities. AI boosts threat detection and job efficiency, automates tasks, and fills skill gaps. However, AI lacks human judgment, emphasizing the need for human validation. Professionals must upskill in AI to adapt to these changes, as demand for AI skills in cybersecurity rises. AI both creates new job opportunities and threatens automation of routine tasks. Continuous learning and ethical AI integration are emphasized for professionals to thrive. The evolving landscape of cybersecurity jobs necessitates a proactive approach to stay relevant in this dynamic field.
Key Takeaways
- AI adoption will reshape cybersecurity roles.
- Demand for AI skills in cybersecurity will rise.
- Job market will see new opportunities and demands.
- Routine tasks may face automation threats.
- Continuous learning and upskilling are crucial for success.
AI's Advantages in Cybersecurity
In the field of cybersecurity, artificial intelligence (AI) offers a multitude of advantages, revolutionizing threat detection and serving as a reliable copilot for professionals. AI in cybersecurity has a significant impact on job efficiency, with 82% of experts foreseeing enhancements in their tasks.
By automating routine processes and analyzing vast amounts of data at incredible speeds, AI allows cybersecurity professionals to focus on more strategic and complex issues. However, there are concerns about the potential misuse of AI in cyberattacks, particularly through the creation of deepfakes and social engineering tactics.
To address these challenges, organizations must establish formal policies on the safe and ethical use of AI. Currently, only 27% of organizations have such policies in place, highlighting the urgent need for enhanced governance and regulation in this area.
Current Limitations of AI in Security
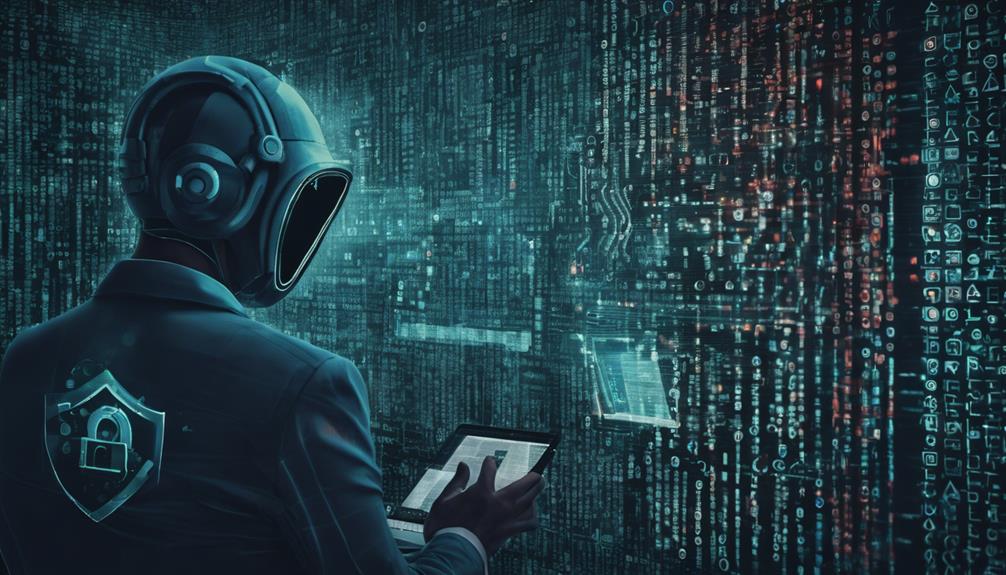
Despite the advancements in AI technology enhancing cybersecurity tasks, current limitations exist that impede its effectiveness in security measures. AI in security lacks human judgment and intuition, necessitating high-quality data for training to prevent bias.
Human validation, enhancement, and domain-specific training are essential to bolster AI's security capabilities. Professionals harbor concerns about the security and privacy of data fed into AI systems, underlining the significance of data quality and human input for continuous validation and enhancement.
To mitigate these limitations, AI systems in security must undergo careful monitoring and training to deliver accurate and unbiased results. Addressing these challenges will require a concerted effort to bridge the gap between AI capabilities and human expertise, ensuring that AI technology in cybersecurity can reach its full potential in safeguarding digital assets and systems against evolving threats.
Impact on Cybersecurity Job Market
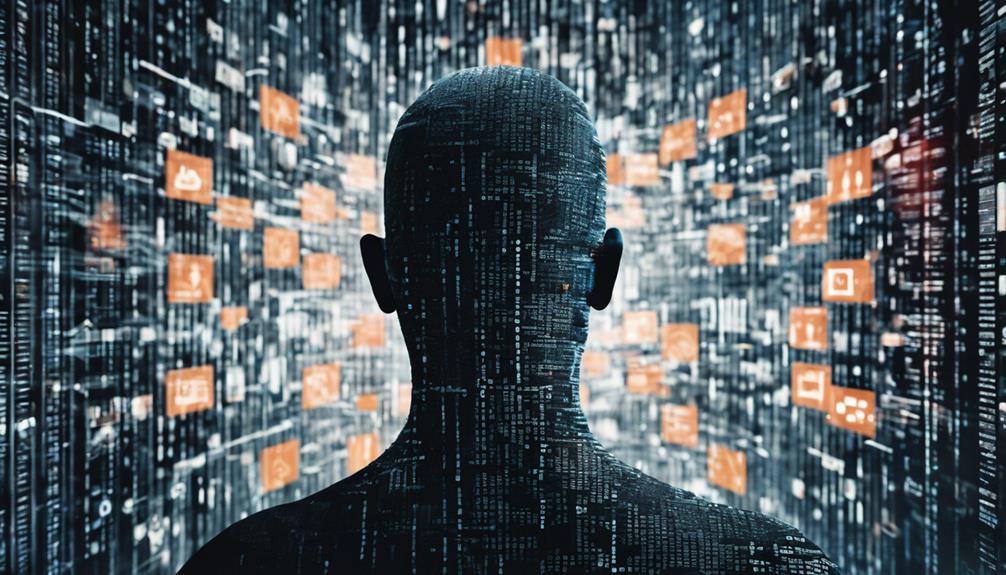
The impact of AI on the cybersecurity job market is multifaceted. Job demand is shifting, requiring professionals to evolve their skills to meet new challenges.
While automation poses threats, it also presents opportunities for cyber experts to leverage AI in enhancing their capabilities for better threat detection and response.
Job Demand Shifts
With the rapid advancements in AI technology, the landscape of cybersecurity job demand is undergoing significant shifts. The integration of AI in cybersecurity is not only enhancing the current capabilities of cyber security professionals in threat detection but also opening up new job opportunities.
As the demand for cyber security professionals continues to rise, AI is helping to fill the skills gap that has led to an expected 3.5 million unfilled cyber security jobs by 2025. AI's impact is particularly beneficial in increasing efficiency and effectiveness in handling cyber threats, making it a valuable tool for both entry-level positions and experienced professionals.
This shift in job demand towards AI-integrated roles highlights the importance of acquiring skills in AI technologies to stay competitive in the evolving cyber security job market. Embracing AI in cybersecurity jobs is not just a trend but a necessity for meeting the growing demands of the industry.
Skills Evolution Needed
As the demand for cybersecurity professionals continues to surge, the necessity for acquiring advanced skills in AI technologies becomes increasingly apparent in order to address the talent shortage and evolving landscape of cyber threats.
The cybersecurity job market is facing a significant skills gap, with an anticipated 3.5 million unfilled positions by 2025. Without prior expertise, professionals find it challenging to enter the field, highlighting the critical need for upskilling in AI tools and technology.
AI's impact on cybersecurity is undeniable, with 88% of professionals expecting significant changes in their roles due to AI in the coming years. Embracing AI tools not only enhances the capabilities of cybersecurity professionals but also paves the way for new job opportunities in the industry.
Automation Threats and Opportunities
In the ever-changing terrain of the cybersecurity job market, professionals must adapt to automation threats and opportunities posed by AI technologies. Cybersecurity professionals believe that AI is impacting their field in substantial ways.
While AI presents opportunities by creating new roles and demands for professionals, it also brings challenges as automation threatens to eliminate routine tasks. As AI continues to advance, it will greatly impact the job market, emphasizing the need for human expertise in ensuring the ethical use of AI in cybersecurity practices.
This shift underscores the evolving role of AI in the industry and highlights the lack of job security for those who do not upskill or specialize.
To navigate these changes successfully, professionals must focus on high-value tasks that require human intervention, emphasizing continuous learning and staying ahead of technological advancements to thrive in the evolving landscape of cybersecurity.
Evolution of AI in Security Roles
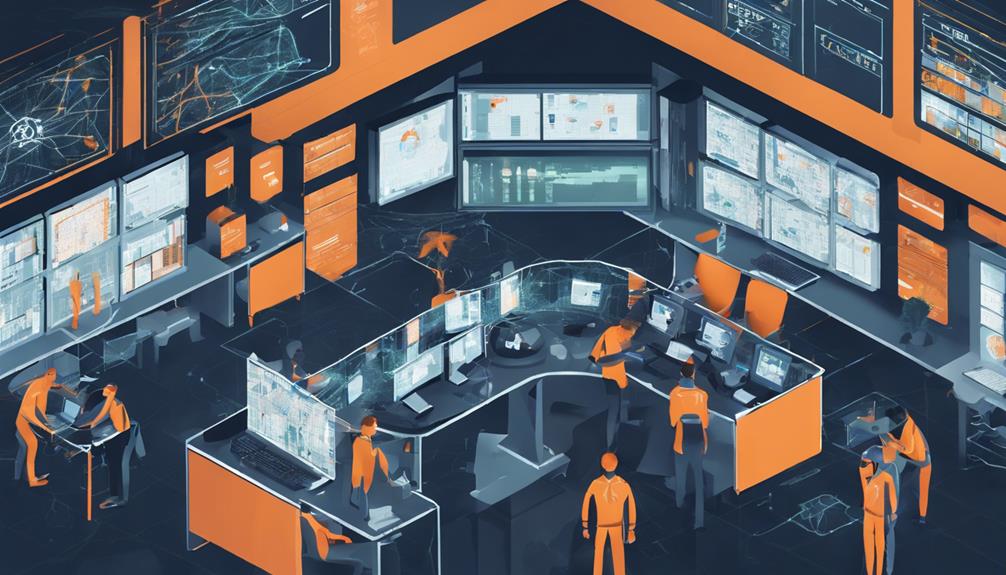
Evolving alongside the rapid advancements in technology, the integration of AI in security roles is reshaping the landscape of cybersecurity professionals' responsibilities. As AI adoption in cybersecurity accelerates, professionals are witnessing significant changes in their daily job functions.
Automation in cybersecurity is becoming more prevalent, with AI handling routine decisions to free up human experts for critical tasks. However, concerns linger about the lack of expertise and awareness within organizations regarding AI integration in security roles.
Cybersecurity jobs are now at a pivotal moment where professionals have the opportunity to lead in implementing secure technology practices through AI. The collaboration between humans and AI is evolving, emphasizing the importance of a symbiotic relationship where each complements the other's strengths.
AI for Good and Bad in Security
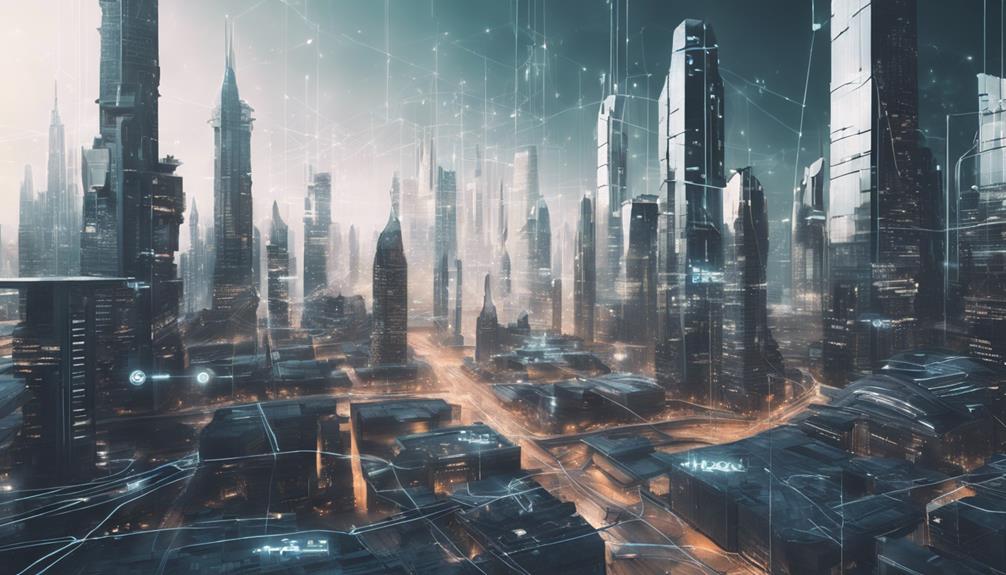
The increasing utilization of AI by malicious actors is dramatically impacting the landscape of cybersecurity, manifesting both beneficial and detrimental implications for security professionals. On one hand, AI tools offer defensive technologies that can analyze vast amounts of data to detect and respond to threats more efficiently.
However, malicious entities are leveraging AI to orchestrate more sophisticated cyber attacks, such as phishing campaigns powered by generative AI to craft convincing messages for social engineering. These advancements enable attackers to produce deep fakes and manipulate voices, escalating the risk of successful infiltrations.
As a result, cybersecurity professionals face the challenge of combating evolving threats that exploit AI capabilities for malicious purposes. Adapting to these changing tactics requires constant vigilance and upskilling to effectively defend against the intricate strategies employed by cybercriminals leveraging AI technology for nefarious activities.
Collaboration Vs. Displacement With AI
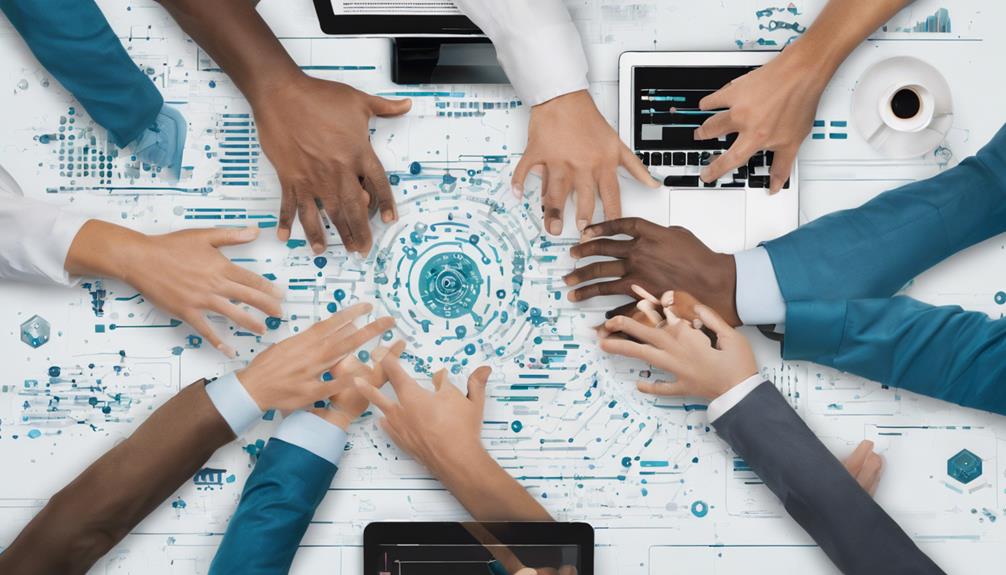
As AI continues to permeate the cybersecurity landscape, professionals are faced with the choice between embracing collaboration or risking displacement in the evolving field. Collaboration among cybersecurity experts is essential to harness the benefits of AI impact on cybersecurity jobs while mitigating the risks of displacement. Organizations must prioritize enhancing expertise and awareness regarding AI integration in cybersecurity to guarantee a smooth shift and secure technology implementation.
To illustrate the importance of collaboration vs. displacement with AI in cybersecurity, consider the following table:
Collaboration Benefits | Displacement Risks |
---|---|
Enhanced threat detection | Job redundancies |
Improved response times | Skill gaps in workforce |
Efficient resource allocation | Resistance to change |
Human Interaction Needs With AI
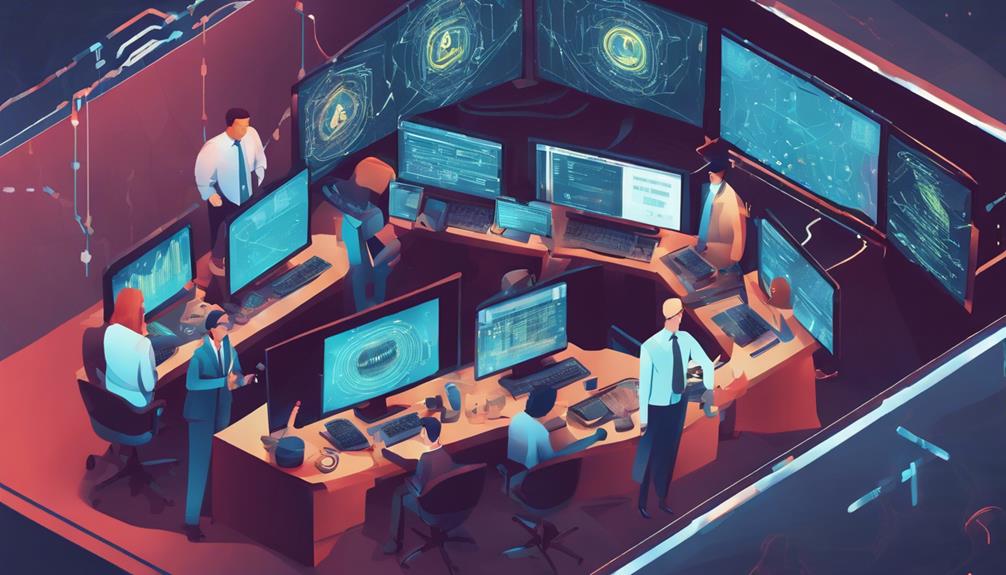
Given the increasing integration of AI in cybersecurity, maintaining effective human interaction with AI systems is essential for cybersecurity professionals to navigate the evolving landscape successfully.
To achieve this, professionals should focus on developing expertise. Continuous learning about AI technologies is vital for cybersecurity professionals to understand their impact on job roles and organizational security.
Enhancing organizational awareness is also crucial. Building a deep understanding within the organization regarding the ethical integration of AI can help in ensuring secure practices and proactive navigation of potential risks.
Moreover, promoting ethical integration is key. Encouraging the ethical use of AI technologies within cybersecurity practices can lead to a more responsible and effective approach, benefiting both professionals and organizations alike.
New Cybersecurity Job Trends
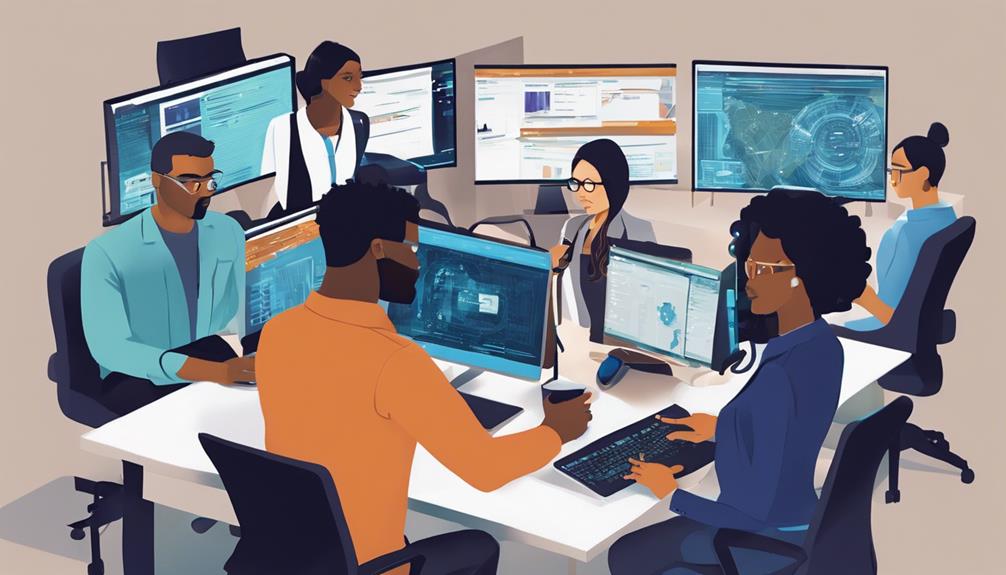
Amidst the evolving landscape of cybersecurity, emerging trends in job opportunities are reshaping the industry's workforce dynamics. With an expected 3.5 million unfilled cybersecurity jobs by 2025, the talent shortage in the industry is glaring.
One significant issue faced is the difficulty in securing entry-level positions without prior experience, exacerbating the skills gap. However, the rise of AI in cybersecurity is seen as a solution to these challenges. AI not only has the potential to fill the skills gap but also to create new job opportunities within the field.
AI adoption in cybersecurity is enhancing the capabilities of professionals, particularly in threat detection, empowering them to combat increasingly sophisticated cyber threats. As organizations integrate AI into their security operations, job growth is anticipated, opening up avenues for individuals to explore new roles and responsibilities in the cybersecurity domain.
This shift towards AI-driven cybersecurity practices is expected to offer a fresh outlook on talent acquisition and skill development, potentially bridging the gap between demand and supply in the industry.
Frequently Asked Questions
How Will AI Affect Cybersecurity Jobs?
AI is poised to revolutionize cybersecurity jobs through automation, threat detection, and response enhancements. As AI technologies evolve, professionals must adapt their skills to leverage these tools effectively, reinforcing the importance of continuous learning and upskilling.
What Is the Main Challenge of Using AI in Cybersecurity?
Amidst the evolving landscape of cybersecurity, the main challenge in utilizing AI lies in the shortage of skilled professionals adept at securing AI technologies. Addressing this expertise gap is imperative for effective AI integration in cybersecurity practices.
How Does AI Contribute to Cyber Security?
AI plays a pivotal role in cybersecurity by enhancing threat detection, incident response, threat intelligence, compliance monitoring, and improving detection quality. It acts as a reliable co-pilot for professionals, integrates with key security platforms, and offers a proactive approach to protection.
Is AI a Risk for Cyber Security?
AI presents both opportunities and risks in cybersecurity. While it enhances defense mechanisms, the misuse of AI by threat actors poses significant risks. Organizations must balance innovation with security measures to mitigate these threats effectively.
Conclusion
To sum up, the impact of AI on cybersecurity jobs is significant and rapidly changing. As AI technology continues to evolve, it offers both advantages and limitations in the field of security.
One interesting statistic to note is that by 2023, it is estimated that there will be a shortage of nearly 3 million cybersecurity professionals worldwide. This highlights the growing need for individuals to adapt to new technologies and skills to meet the demands of the ever-evolving cybersecurity landscape.
Advanced Cybersecurity
AI and Machine Learning in Cybersecurity: A Powerful Combination
Peek into how AI and machine learning revolutionize cybersecurity, enhancing threat detection and incident response with cutting-edge technology.
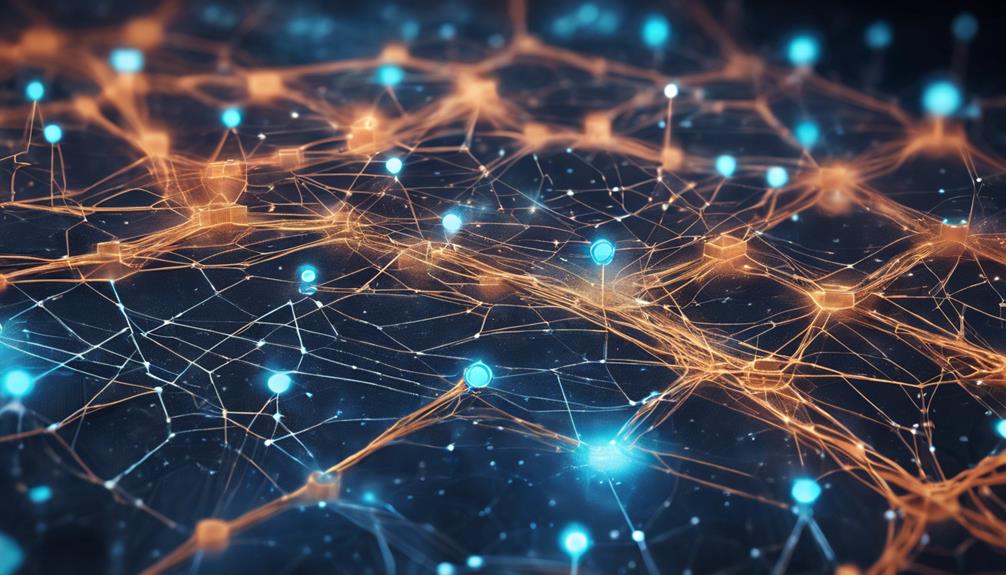
AI and machine learning are transforming cybersecurity, bolstering threat detection, incident response, and security operations. With the evolution from rule-based to deep learning systems, AI now excels in detecting anomalies and patterns. Utilizing historical data, it identifies attack patterns and clusters threats for streamlined response strategies. AI enhances incident response by analyzing data in real-time, automating actions, and providing insightful recommendations. By analyzing vast data sets, it swiftly adapts to dynamic environments, reducing response times. Future trends suggest a deeper integration of AI with security, focusing on user behavior, transparency, and autonomous security operations. The future of cybersecurity is AI-driven.
Key Takeaways
- AI enhances threat detection by analyzing vast data for patterns and anomalies.
- Machine learning identifies known attack patterns for proactive defense.
- Automation of security tasks improves incident response times and efficiency.
- Real-time anomaly detection aids in swift response to evolving threats.
- Integration of AI and ML revolutionizes cybersecurity operations for enhanced protection.
Evolution of AI in Cybersecurity
The evolution of AI in cybersecurity traces back to the mid to late 1980s when early rule-based systems were first utilized for anomaly detection. Since then, the landscape of security measures has been greatly transformed by the integration of machine learning algorithms.
This evolution has been essential in combating advanced cyber threats through enhanced threat detection capabilities. In the late 2000s, supervised learning algorithms played a pivotal role in boosting the accuracy of threat detection systems.
However, it was the advent of deep learning in the 2010s that truly revolutionized cybersecurity. Deep learning enabled the recognition of intricate patterns, providing a more robust defense against evolving threats.
As AI and machine learning technologies continue to progress, they play a crucial role in fortifying security measures across various sectors. The ongoing evolution in anomaly detection, pattern recognition, and overall threat detection capabilities underscores the importance of AI in enhancing cybersecurity protocols.
Machine Learning Techniques for Threat Detection
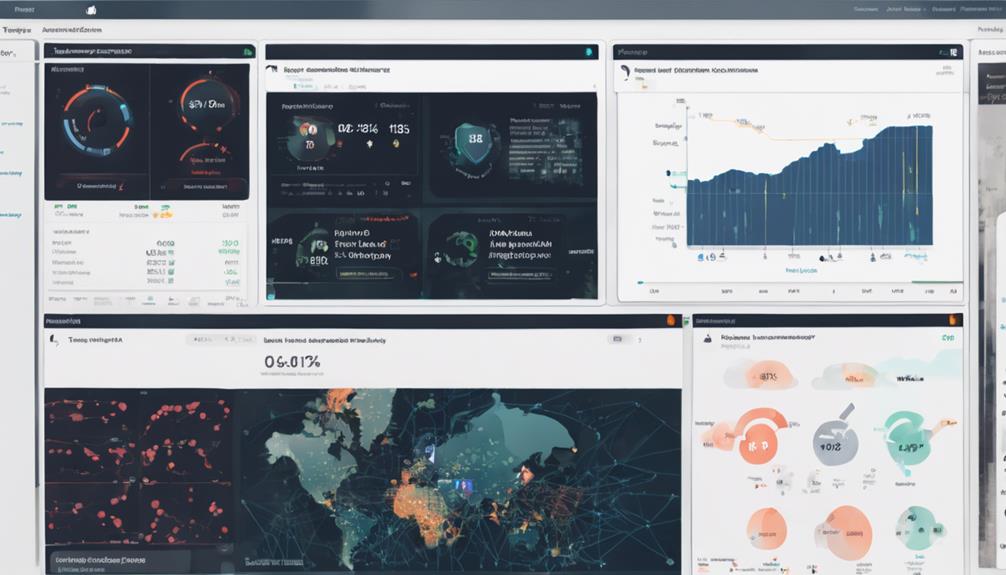
Machine learning techniques employed in cybersecurity play a pivotal role in identifying and mitigating potential threats within network environments. By leveraging AI algorithms to analyze network traffic, cybersecurity systems can proactively detect anomalies and unusual behavior, enhancing threat intelligence for a more secure environment.
These techniques utilize historical data to identify patterns and trends, enabling the detection of known attack patterns and clustering potential threats based on complexity for better risk assessment. Through machine learning, cybersecurity professionals can build a hierarchy of threats, allowing for a more efficient response to cyber threats.
This approach not only aids in threat detection but also helps in improving overall security posture by staying ahead of emerging threats. By embracing machine learning for threat detection, organizations can greatly enhance their cybersecurity defenses and safeguard their digital assets more effectively.
Enhancing Incident Response With AI
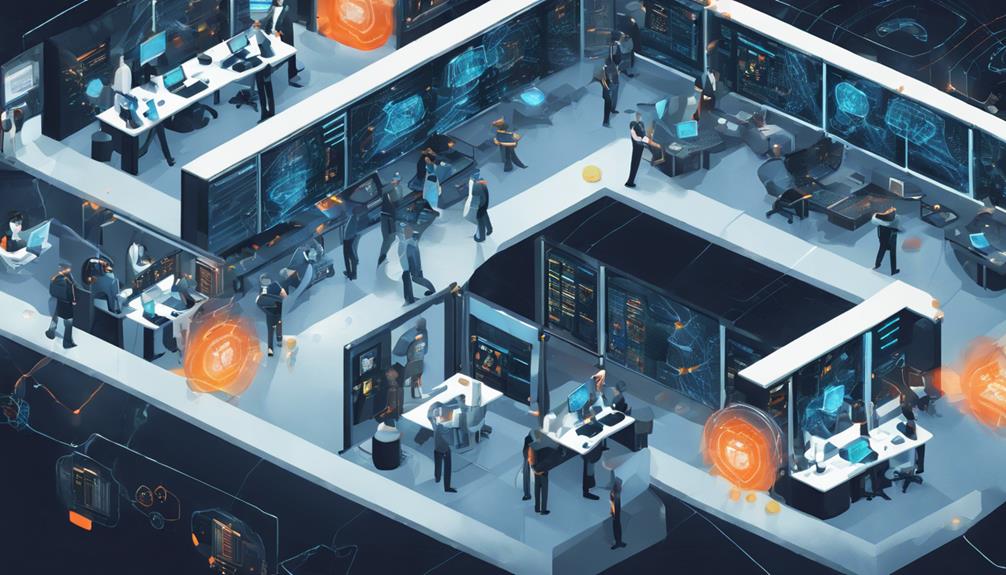
Moreover, utilizing AI technologies in incident response revolutionizes the efficiency and effectiveness of handling security breaches by leveraging advanced data analysis capabilities. Machine learning algorithms embedded in AI-powered systems can detect anomalies in real-time, allowing for swift identification and response to potential threats.
This automation of response actions based on predefined rules streamlines incident resolution processes, ensuring a proactive approach to cybersecurity incidents. Additionally, AI can prioritize alerts and escalate them to the appropriate stakeholders, facilitating efficient incident management by focusing resources where they are most needed.
Moreover, AI enhances decision-making during incident response by providing valuable insights and recommendations drawn from past incidents. By learning from historical data, AI continuously improves incident response strategies, making them more effective and adaptive to evolving cyber threats.
Incorporating AI into incident response not only boosts the speed of resolution but also enhances the overall security posture of organizations.
Leveraging AI in Security Operations
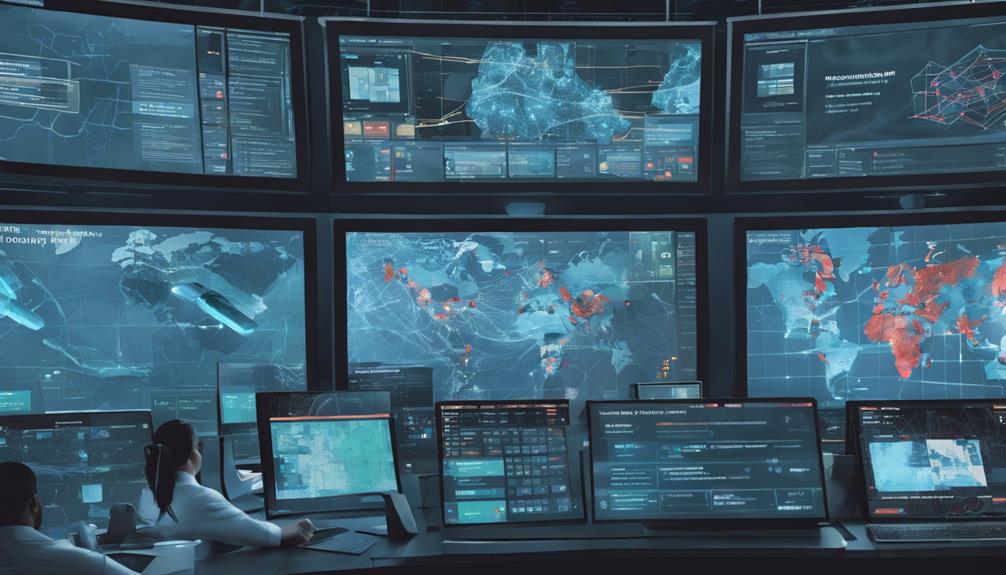
Incorporating artificial intelligence (AI) into security operations revolutionizes threat detection and response capabilities. By leveraging machine learning (ML) algorithms, AI can analyze vast amounts of data to identify patterns of suspicious behavior, enabling proactive threat mitigation. This ability to quickly identify potential threats in networks enhances overall security measures greatly.
Furthermore, AI automates repetitive security tasks, allowing human analysts to focus on more complex threats that require human intervention.
Security operations centers (SOCs) powered by AI can adapt to dynamic environments in real-time, leading to faster incident response times and an improved cybersecurity posture overall.
Future Trends in AI for Cybersecurity
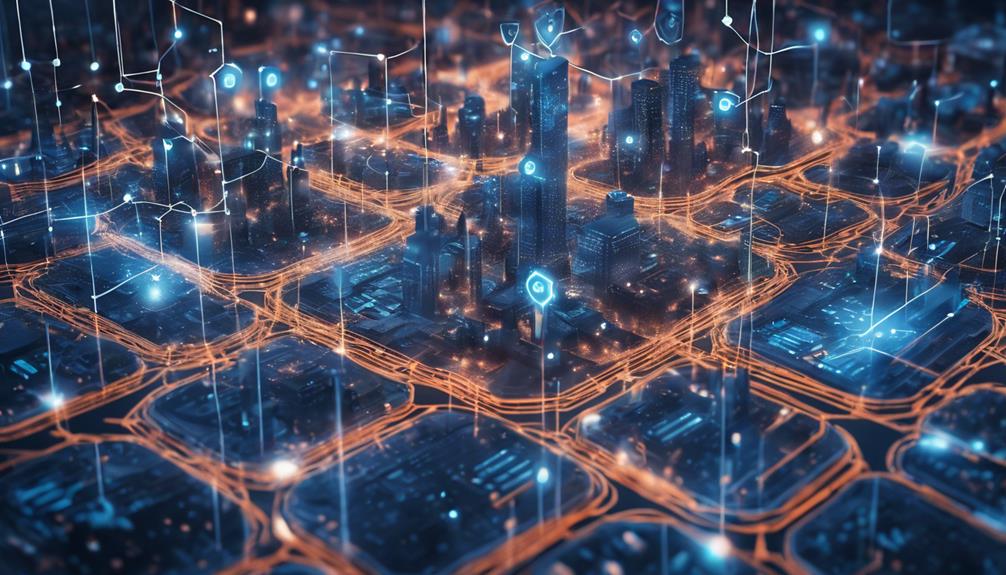
The future trajectory of AI in cybersecurity entails the evolution towards countering advanced cyber threats through innovative technologies and strategies. As the landscape of cybersecurity continues to evolve, here are three key trends shaping the future of AI in cybersecurity:
- Integration with Diverse Security Aspects:
AI and Machine Learning are increasingly being integrated with IoT, cloud security, and endpoint protection to enhance overall cybersecurity defenses and enable proactive threat detection in the face of large volumes of data.
- Significant User Behavior Analysis:
AI plays a significant role in analyzing user behavior patterns to detect anomalies and potential threats, enabling cybersecurity professionals to conduct more effective threat analysis and respond promptly to adversarial attacks.
- Explainable AI and Autonomous Security Operations:
The adoption of Explainable AI is gaining momentum, improving transparency in complex machine learning models. Additionally, AI-driven autonomous security operations centers (ASOCs) are on the rise, automating threat response mechanisms and policy enforcement while facilitating collaboration between AI systems and human experts to develop adaptive cybersecurity strategies.
Frequently Asked Questions
How Is AI and Machine Learning Used in Cyber Security?
AI and machine learning in cybersecurity entail anomaly detection, predictive analytics, phishing detection, automated threat response, and vulnerability management. These technologies enhance threat detection, predict cyber-attacks, and improve overall security by efficiently identifying potential threats and mitigating risks.
Can I Combine AI and Cyber Security?
Yes, the integration of AI in cybersecurity enhances threat detection, response, and mitigation capabilities. Leveraging AI technologies automates tasks, predicts cyber threats, and improves overall security posture. Combining AI with cybersecurity is a recommended approach for robust protection.
Will AI and ML Play a Role in the Future of Cybersecurity?
AI and ML are poised to have a substantial impact on the future of cybersecurity by enhancing defense mechanisms, predicting threats, and improving security postures. These technologies will play a pivotal role in mitigating risks and safeguarding critical infrastructure.
What Is the Intersection of AI and Cybersecurity?
In the intricate dance between artificial intelligence and cybersecurity, AI amplifies threat detection capabilities by unraveling patterns in the digital tapestry, revealing hidden vulnerabilities and fortifying defenses with predictive prowess.
Conclusion
To sum up, the integration of AI and machine learning in cybersecurity is a game-changer, revolutionizing the way organizations detect and respond to threats.
The power of these technologies to analyze vast amounts of data and adapt to evolving threats is unparalleled.
As we move forward, the future of cybersecurity will be shaped by the continued advancements in AI, creating a more secure digital environment for all.
The possibilities are endless, and the impact is immeasurable.
Advanced Cybersecurity
Cybersecurity Using AI: Modern Techniques for Enhanced Protection
Optimize your cybersecurity defenses with cutting-edge AI technology, revolutionizing protection against cyber threats and bolstering your security posture.
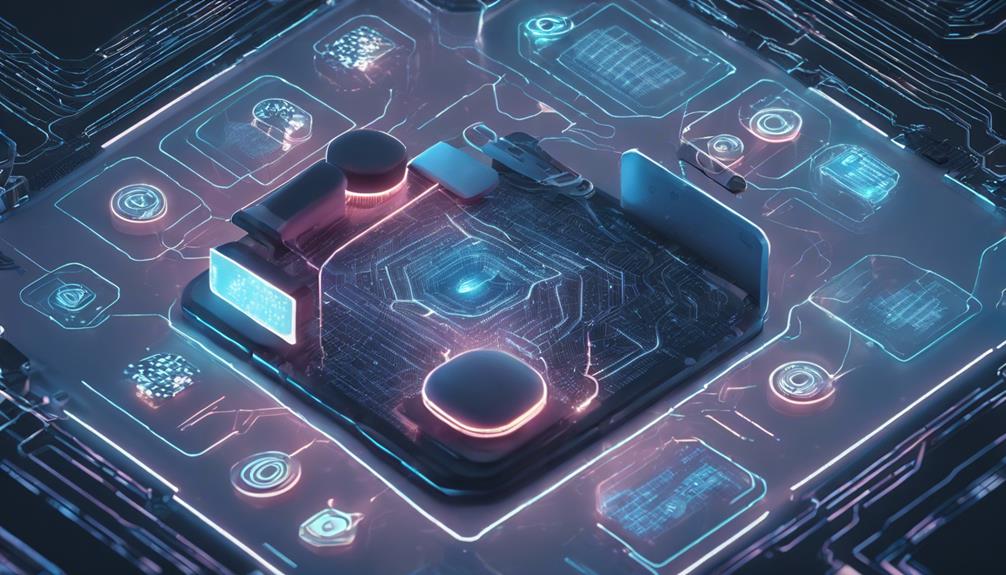
Enhance your cybersecurity with AI, reshaping protection against evolving cyber threats. AI boosts threat detection and response with machine learning technology. Behavioral analysis and anomaly detection heighten security vigilance. Predictive analytics prioritize threat action, automating tasks for efficiency. Proactive defense through AI boosts vulnerability management effectiveness. Swift identification of unknown threats secures systems effectively. Real-time monitoring and adaptive access control fortify cybersecurity posture. Dive deeper into AI's impact on modern protection techniques for thorough cybersecurity enhancement.
Key Takeaways
- AI enhances threat detection with machine learning algorithms.
- Predictive analytics prioritize potential threats for proactive defense.
- Automation streamlines tasks and manages vulnerabilities effectively.
- AI identifies unknown threats swiftly to bolster security measures.
- Integration of AI is crucial in the evolving threat landscape for robust protection.
AI in Cybersecurity Overview
AI in cybersecurity plays a pivotal role in revolutionizing threat detection and incident response through the utilization of machine learning algorithms. By harnessing the power of Artificial Intelligence (AI) and Machine Learning (ML), cybersecurity systems can efficiently detect anomalies and behavioral patterns that signal advanced threats.
Through anomaly detection and behavioral analysis, AI can handle complex security tasks that involve vast amounts of data analysis, enabling proactive defense mechanisms. Predictive analytics further enhances these capabilities by predicting potential threats and prioritizing them for immediate action.
The integration of AI in cybersecurity not only automates tasks but also streamlines incident response, providing real-time threat prevention. By reducing the workload on security teams, AI allows organizations to improve their overall cybersecurity effectiveness.
This modern approach to cybersecurity is essential in combating the ever-evolving landscape of cyber threats, where diverse data sources need to be analyzed thoroughly to ensure robust protection.
Importance of AI in Protection
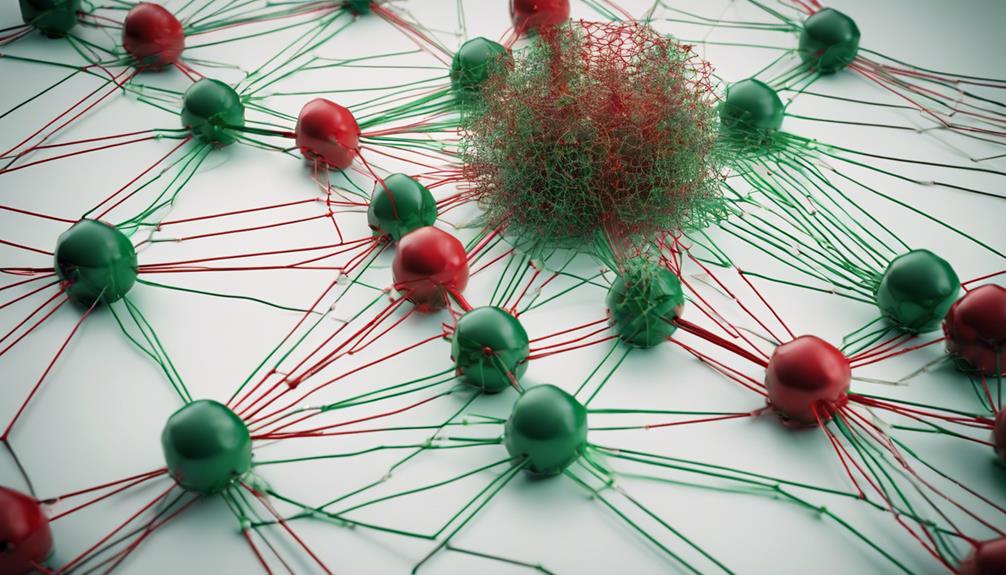
The significance of incorporating Artificial Intelligence technology in cybersecurity for heightened protection measures cannot be overstated. AI plays a pivotal role in cybersecurity by enhancing threat detection capabilities, improving response times, and enabling proactive defense through predictive analytics.
Leveraging AI allows for automating tasks, integrating diverse data sources, and efficiently managing vulnerabilities to prioritize threats effectively. By identifying unknown threats and analyzing large volumes of data swiftly, AI guarantees overall security in the digital landscape.
Implementing AI in cybersecurity not only reduces the workload for security teams but also enhances the ability to address complex cyber threats with precision. In today's ever-evolving threat landscape, where cyberattacks are becoming more sophisticated, the adoption of AI is essential for staying ahead of potential risks and safeguarding sensitive information effectively.
Threat Detection With AI
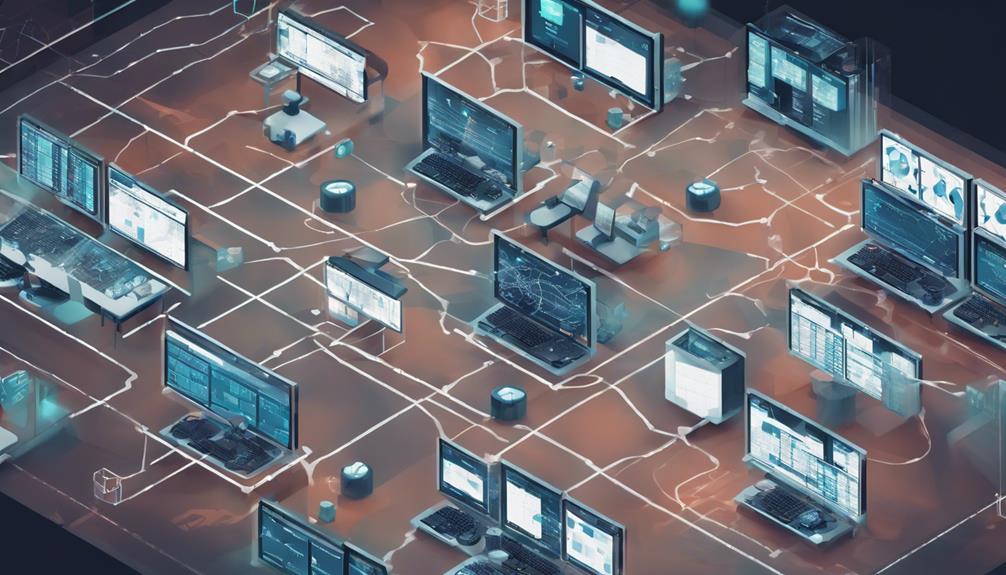
How does artificial intelligence revolutionize threat detection in cybersecurity?
AI plays a pivotal role in enhancing threat detection by leveraging Machine Learning (ML) algorithms to analyze vast amounts of data in real-time. By identifying patterns and anomalies that signify potential threats, AI empowers cybersecurity professionals with automated incident response capabilities, greatly improving response time and accuracy.
Behavioral analysis through AI models enables the proactive identification of suspicious activities, bolstering defense against emerging threats. Additionally, AI solutions continuously monitor and analyze network data, allowing organizations to stay ahead of evolving threats.
AI for Vulnerability Management
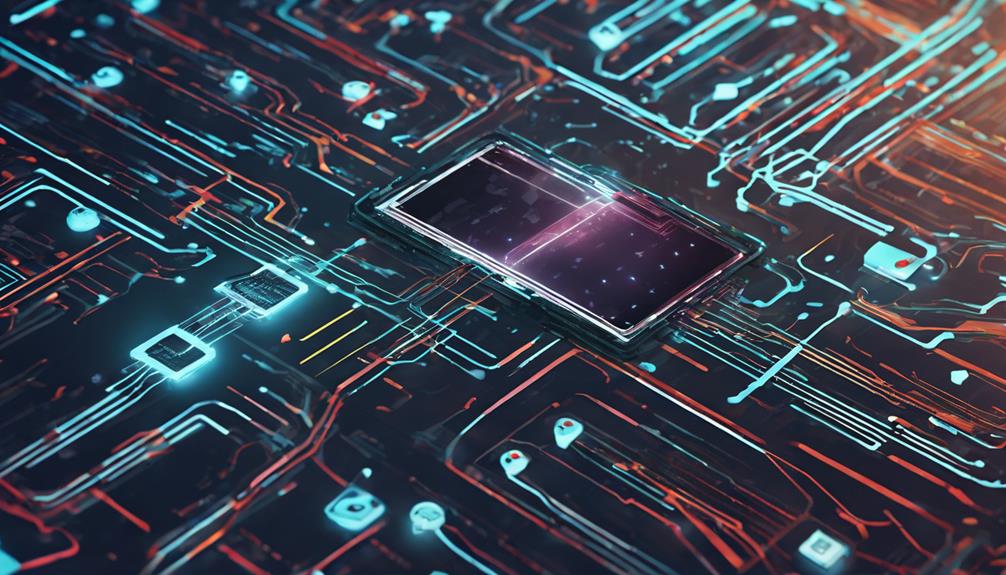
Utilizing advanced algorithms and automation, organizations leverage artificial intelligence to enhance the efficiency of identifying and prioritizing security weaknesses in vulnerability management. Machine learning algorithms play an important role in analyzing vast amounts of data to detect vulnerabilities in systems and networks. AI automates vulnerability scanning and assessment processes, greatly reducing the time required for manual analysis.
This automation not only saves time but also provides real-time insights into potential vulnerabilities, enabling proactive security measures to be implemented promptly. By addressing weaknesses before exploitation, vulnerability management with AI enhances the overall cybersecurity posture of an organization.
In the fast-evolving landscape of cybersecurity threats, utilizing AI for vulnerability management is a proactive approach that can help organizations stay ahead of potential security breaches. By incorporating AI-powered tools into their security strategies, organizations can fortify their defenses and protect against vulnerabilities effectively.
Enhanced Security Measures
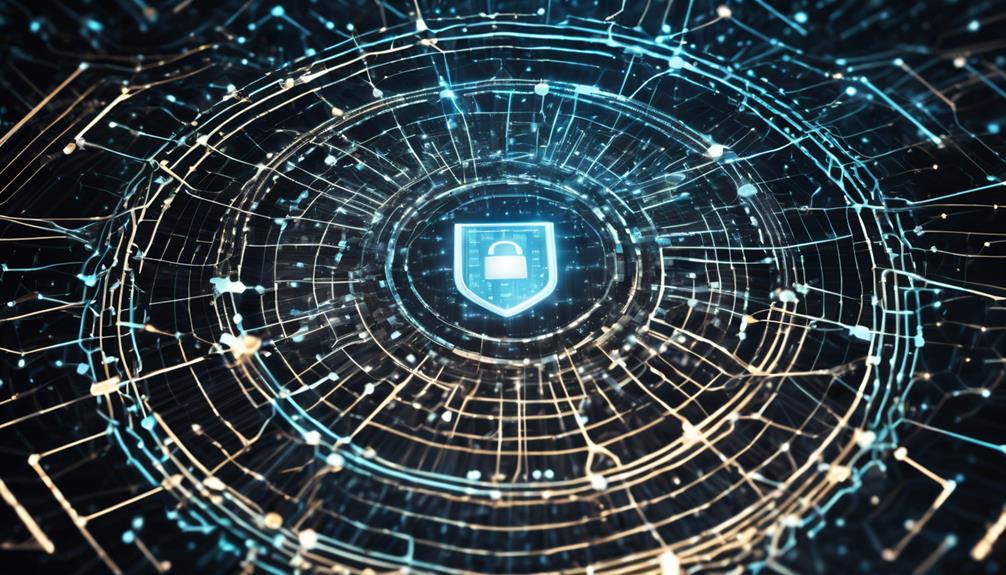
AI's role in cybersecurity extends beyond vulnerability management to encompass enhanced security measures that prioritize critical issues and automate response actions. By leveraging AI, organizations can enhance their defense against emerging threats through proactive threat management.
AI plays an important role in reducing the risk of cyber incidents by prioritizing critical security issues and swiftly fixing them. It automates response actions, enabling quick detection of anomalies and providing actionable intelligence on potential cyber threats.
Additionally, AI's ability to scan systems for risks, suggest security improvements, and support decision-making processes reinforces the least privilege principle, which limits access rights for users to only what are strictly required. This approach noticeably reduces the risk of data breaches and unauthorized access, ultimately enhancing overall cybersecurity posture.
Embracing AI-driven security measures empowers organizations to stay ahead of evolving threats and ensure robust protection against cyberattacks.
Accelerated Response Times
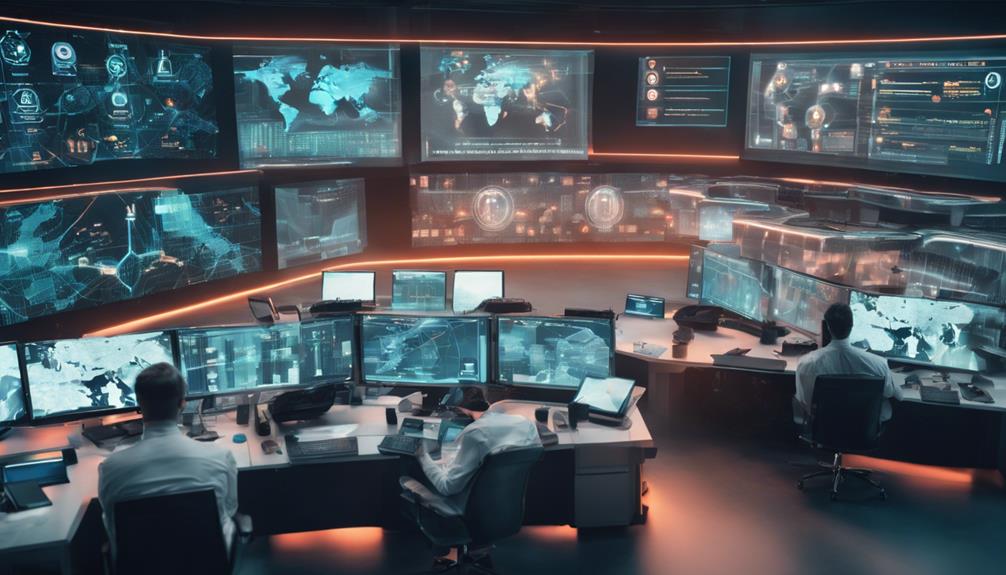
Accelerated response times in cybersecurity using AI offer a significant advantage to organizations.
Real-time threat detection guarantees immediate identification of potential risks, enabling swift action to prevent security breaches.
Automated incident analysis, coupled with instant security alerts, empowers teams to respond effectively, enhancing overall cybersecurity resilience.
Real-Time Threat Detection
Efficiency in identifying and responding to cybersecurity threats is greatly enhanced through the integration of artificial intelligence technologies. AI-powered systems enable real-time threat detection by swiftly analyzing vast amounts of data, leading to immediate threat identification.
This proactive defense mechanism allows for accelerated incident responses, prioritizing critical threats for automated actions. By continuously monitoring networks, endpoints, and applications, AI contributes to overall cybersecurity posture by reducing the time to detect and respond to cyber incidents.
Real-time threat detection with AI not only minimizes potential damages but also empowers organizations to take quick action against emerging threats. The combination of AI's immediate threat detection capabilities and automated responses strengthens the resilience of cybersecurity defenses.
Embracing AI in cybersecurity operations can greatly enhance the security posture of organizations, providing a robust shield against evolving cyber threats.
Automated Incident Analysis
Automated incident analysis plays a pivotal role in enhancing response times in cybersecurity operations. By utilizing AI algorithms, organizations can achieve real-time incident detection and rapid incident analysis, greatly reducing the mean time to detect and respond to cyber threats. This proactive approach enables quicker threat mitigation and enhances overall cybersecurity posture and security effectiveness.
AI-driven automated incident response can efficiently process and prioritize critical threats for immediate action, ensuring that the most pressing issues are addressed promptly. Compared to manual analysis, AI algorithms can process and analyze incidents within seconds, a task that would typically take human analysts hours or even days to complete. This acceleration in response times by up to 65% underscores the importance of automated incident analysis in maintaining a robust cybersecurity defense.
Incorporating automated incident analysis into cybersecurity strategies not only improves response times but also minimizes potential damages by swiftly identifying and addressing threats, ultimately fortifying the organization's security resilience.
Instant Security Alerts
Utilizing AI-powered systems for generating instant security alerts greatly enhances organizations' ability to swiftly respond to potential cyber threats. AI systems can analyze vast amounts of data in real-time, allowing for the immediate detection of suspicious activities that may indicate security incidents. This proactive approach notably reduces response times to cyber threats, sometimes by up to 65%, enabling organizations to take timely action for enhanced protection.
AI-powered tools provide real-time monitoring for swift detection of security incidents.
Instant security alerts offer actionable insights to respond effectively to cyber threats promptly.
Accelerated response times facilitated by AI systems lead to quicker containment and mitigation of potential damages.
Authentication Security With AI
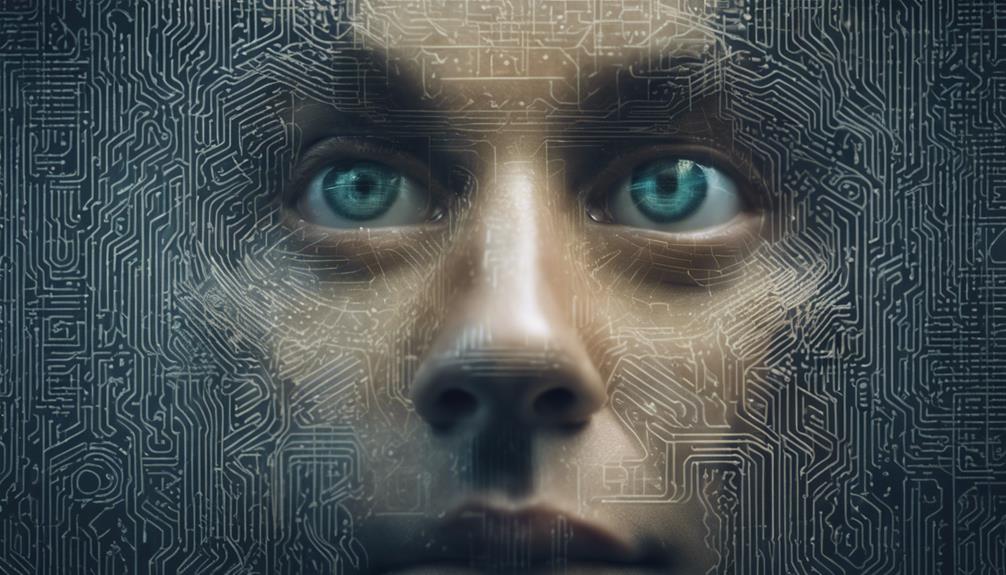
Enhancing authentication security with AI involves utilizing advanced biometric authentication methods. This includes analyzing user behavior for potential threats and implementing adaptive access controls.
By integrating AI-driven solutions, organizations can verify user identities based on unique behavioral traits like typing speed or mouse movements. This helps in bolstering security measures against unauthorized access attempts.
These innovative approaches provide a proactive defense mechanism that adapts to evolving cybersecurity risks. They ensure robust multi-factor authentication protocols for heightened protection.
AI-Driven Biometric Authentication
AI-driven biometric authentication leverages unique biological traits such as fingerprints or facial recognition to provide secure access to systems and data. This innovative approach enhances security measures by reducing the risk of unauthorized access through impersonation.
By utilizing advanced AI algorithms, biometric data is analyzed to accurately and swiftly verify user identities, thereby offering a seamless and efficient authentication process. The integration of AI in biometric authentication guarantees a high level of security, safeguarding against identity theft and fraudulent activities.
Continuous improvements in AI technology further enhance the reliability and precision of biometric authentication systems, reinforcing their efficacy in protecting sensitive information.
- Enhanced Security: Utilizes unique biological traits for secure access.
- Efficient Verification: AI algorithms analyze biometric data to verify identities.
- Protection Against Fraud: Offers a high level of security to prevent identity theft and fraud.
Behavioral Analysis for Security
Behavioral analysis for security, bolstered by artificial intelligence technology, plays a pivotal role in enhancing authentication security measures.
By utilizing AI-driven behavioral analysis, organizations can effectively monitor and detect unusual user behavior, reducing the reliance on traditional authentication methods like passwords. This proactive approach enables the real-time identification of suspicious activities, allowing for immediate response and enhanced security measures.
Through the detection of anomalies in user behavior, AI models can prevent unauthorized access to sensitive information, safeguarding against potential breaches. Additionally, behavioral analysis powered by AI offers a more secure and efficient authentication process, greatly minimizing the risk of fraudulent activities.
Adaptive Access Control
Authentication security in cybersecurity is greatly strengthened by the implementation of adaptive access control, leveraging AI technology to analyze and adjust user behavior in real-time. This advanced approach enhances authentication security by dynamically adapting access permissions based on user behavior, providing a proactive defense against potential security threats.
Key points to note about adaptive access control include:
- AI algorithms assist in identifying unusual user patterns that may indicate security risks.
- Adaptive access control systems continuously monitor user access patterns to detect anomalies and prevent unauthorized access attempts.
- By dynamically adjusting security measures based on real-time user behavior, adaptive access control guarantees a more robust defense against cyber threats.
Through the utilization of AI-driven technologies, organizations can fortify their cybersecurity posture by implementing adaptive access control mechanisms that respond intelligently to evolving user behaviors and potential security challenges.
Automation in Cybersecurity
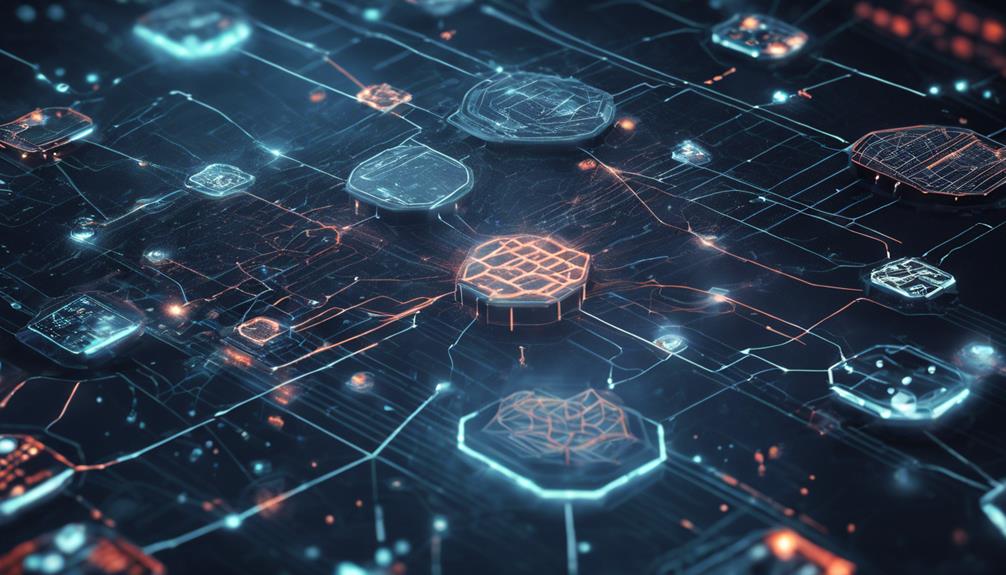
Utilizing automation in cybersecurity processes enhances operational efficiency and response capabilities in addressing cyber threats. AI-driven automation plays an essential role in securing data by enabling real-time monitoring and swift incident response.
With the ability to consolidate and analyze vast amounts of security data efficiently, AI automation streamlines vulnerability assessments and enhances overall security operations' effectiveness. By automating log analysis and vulnerability assessments, security teams can minimize manual tasks, allowing them to focus on more complex cybersecurity challenges.
Additionally, AI automation in cybersecurity not only improves efficiency but also contributes to cost reduction by optimizing the resources required for monitoring and incident response. Embracing AI-driven automation in cybersecurity operations is vital for staying ahead of evolving cyber threats, ensuring robust protection for sensitive data and critical systems.
Frequently Asked Questions
How Does AI Enhance Cybersecurity?
AI enhances cybersecurity by leveraging advanced algorithms to analyze data, detect threats efficiently, automate incident responses, and predict potential risks. It enables proactive defense by staying ahead of evolving threats and streamlining security operations for professionals.
What Is an Example of Cyber Security in Ai?
An example of cybersecurity in AI involves utilizing machine learning algorithms to analyze network data for threat detection. By identifying patterns and anomalies, AI can proactively prevent unknown threats, continuously improving security measures to mitigate cyber risks effectively.
How Can AI Help in Data Protection?
AI aids data protection by analyzing vast data sets to detect anomalies and threats. Machine learning algorithms identify patterns indicating security risks. Real-time monitoring tracks user behavior for unauthorized access. AI automates security tasks, prioritizes alerts, and enhances data protection measures.
Can Cyber Security Be Replaced by Ai?
While AI can bolster cybersecurity defenses, it cannot entirely replace human expertise. Just as a ship needs both its compass and captain, cybersecurity requires the synergy of AI's efficiency with human decision-making for effective threat mitigation and strategic defense.
Conclusion
To sum up, AI technology in cybersecurity provides advanced protection against threats, enhances vulnerability management, and ensures accelerated response times. By implementing AI-driven security measures, organizations can strengthen their defenses and safeguard sensitive data.
It is ironic that the very technology used to create cyber threats can also be leveraged to combat them effectively. Embracing AI in cybersecurity is not just a trend but a necessary step towards securing digital assets in the modern age.
-
Hackathons1 month ago
How to Find Hackathons: Discover Upcoming Hackathon Opportunities Through Community Forums and Listing Platforms.
-
Hackathons1 month ago
Do Hackathons Look Good on Resume: Participating in Hackathons Is an Impressive Accomplishment to Highlight on Resumes.
-
Hackathons1 month ago
Remote Hackathons: Virtual Hackathons Enable Global Teams to Collaborate Remotely.
-
Hackathons1 month ago
MIT Hackathons: the Prestigious MIT Hosts Innovative Student-Led Hackathons in Boston.
-
Hackathons1 month ago
Internal Company Hackathons: Corporations Leverage Internal Hackathons to Crowdsource Ideas and Drive Innovation.
-
Hackathons1 month ago
2024 Hackathons: Get Ready for the Next Wave of Cutting-Edge Hackathon Events in 2024.
-
Hackathons1 month ago
Internal Hackathons: Companies Host Internal Hackathons to Drive Innovation From Within.
-
Hackathons1 month ago
Hackathons in Usa: Don't Miss the Major National and Regional Hackathons Across the United States.